Chapter 5: Energy Consumption and Emissions from Transportation
Chapter Overview
In this chapter, we move the focus from non-motorized transportation to automobility. The basics of energy consumption pathways for automobility are introduced. Students learn about the history of energy mix and emissions control processes at both stationary and mobile emissions sources. We then discuss the methods for calculating energy requirements for different transportation activities. This chapter introduces the methods to calculate greenhouse gas emissions and other transportation related pollutant emissions. The concept of near-road exposure to public health outcomes is explained next.
Chapter Topics
- Energy Pathways for Automobility
- Life Cycle Analysis
- Fuel Use and Emissions for Road Traffic
- Aggregation and Estimation of Systemwide Energy Consumption and Emissions
Learning Objectives
At the end of the chapter, the reader should be able to do the following:
- Recognize the historical context of fossil fuel dependency in the transportation sector and list the various energy pathways to meet the same transportation needs.
- Estimate energy consumption for multimodal transportation activities.
- Aggregate energy consumption and emissions of different transportation components to estimate the systemwide energy consumption and emissions.
- Calculate total emissions for different policy alternatives related to automobility.
- Calculate the energy and environmental footprints of the existing scenario and the proposed improvements.
Energy Pathways for Automobility
Pollutant and greenhouse gas (GHG) emissions can be reduced in many ways. See Figure 5.1 for a simplified framework. GHG emissions may be treated as primary energy carbon intensity multiplied by vehicle and transportation efficiency multiplied by total travel demand.
Primary energy carbon intensity can be reduced by using lower-carbon fuels or low-carbon electrification, which is described later in this section. The energy needed to drive a specific distance can be reduced by improving both (1) vehicle efficiency and (2) transportation system efficiency, again assuming no induced demand. This analytical construct—separating the determinants of emissions into carbon intensity, efficiency, and demand—can be used as a policy framework. A large carbon tax would address all three strategies, though it must be very large to be effective. In practice, an environmentally sustainable transportation solution will depend on a mix of policies
and strategies.
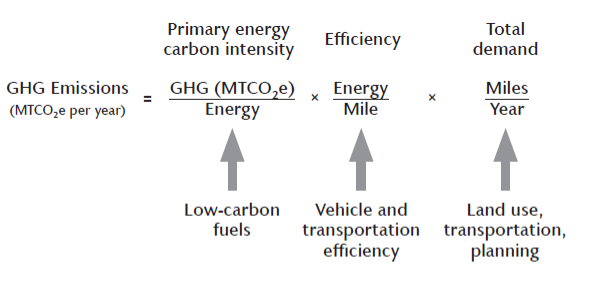
As you can see from Figure 5.1, different vehicle technologies and fuel technologies will directly impact the total amount of GHG emissions from the transportation sector. In the following subsections, we will discuss various vehicle and fuel technologies available in the market.
Vehicle (Powertrain) Technology
There has been considerable effort over the years to make vehicles more energy efficient, thereby reducing pollutant and GHG emissions. Many of these vehicle-based technologies are described in Chapter 6. In recent years, vehicles have benefited from lighter materials and more-efficient combustion engines and powertrains. In just the past few years, the greater use of electric powertrains, including gasoline-electric, plug-in hybrid, battery electric, and fuel cell electric technologies, has provided the promise of even much greater efficiency improvements. Overall vehicle efficiency improvements are illustrated in Figure 5.2 for different areas of the world.
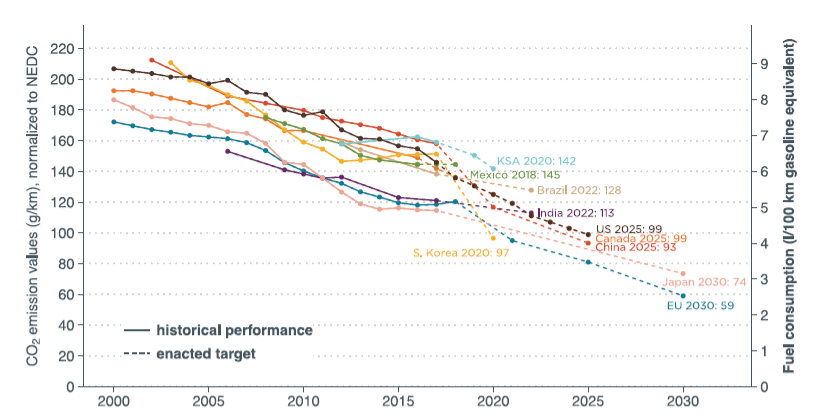
The improvements are due to a combination of aggressive policies and large technology investments by automobile manufacturers. The increasing use of electric powertrains provides the promise for continued improvements in energy efficiency. The continuing drop in battery costs assures that this trend will continue into the foreseeable future. Figure 5.3 illustrates the number of electric vehicles (EVs) that are being introduced in different parts of the world.
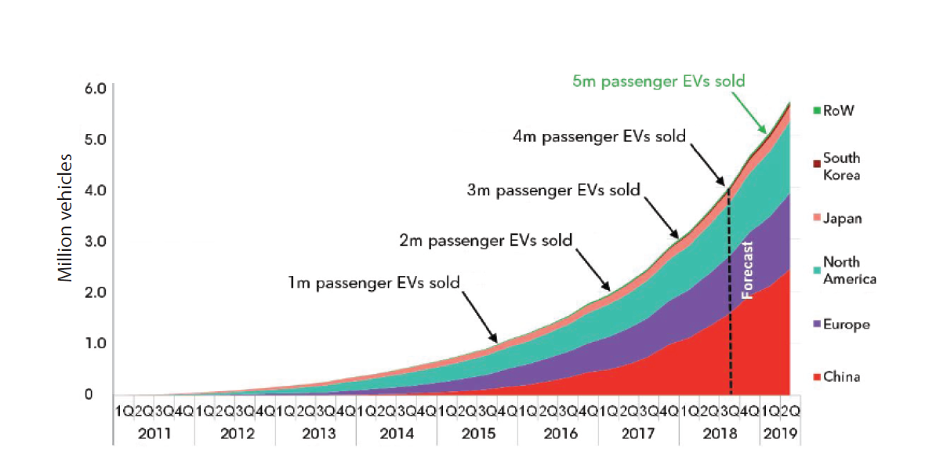
Fuel Technology
Another key strategy for reducing GHG emissions is to utilize low-carbon fuels. Today’s dominant fuel for transportation is gasoline, followed by diesel fuel and then jet fuel (Figure 5.4). All of these fuels are petroleum based and contribute significantly to CO2 emissions. A number of other fuels are being introduced that are less carbon intensive, including bio-based fuels, electricity, and hydrogen. Their market share is currently quite small when compared with petroleum-based fuels. As described in Chapter 6, both electricity and hydrogen (as well as biofuels) can be utilized as effective energy carriers for transportation. Liquid biofuels have the advantage of being easily portable and having high energy density, like petroleum fuels. When made from crop and food wastes, liquid and gaseous biofuels have very low life cycle greenhouse gas emissions, sometimes even less than zero because waste disposal and methane leakage are avoided. With steady improvements in processing and farming, even biofuels made from crops, such as corn and sugarcane, tend to be significantly superior to petroleum fuels. As processes for converting grasses, trees, and other cellulosic material into liquids are improved, resulting in even lower life cycle greenhouse gas emissions, biofuels will likely prove the superior alternative fuel for aviation and perhaps long-haul trucking, where portability and high energy density are valued most highly.
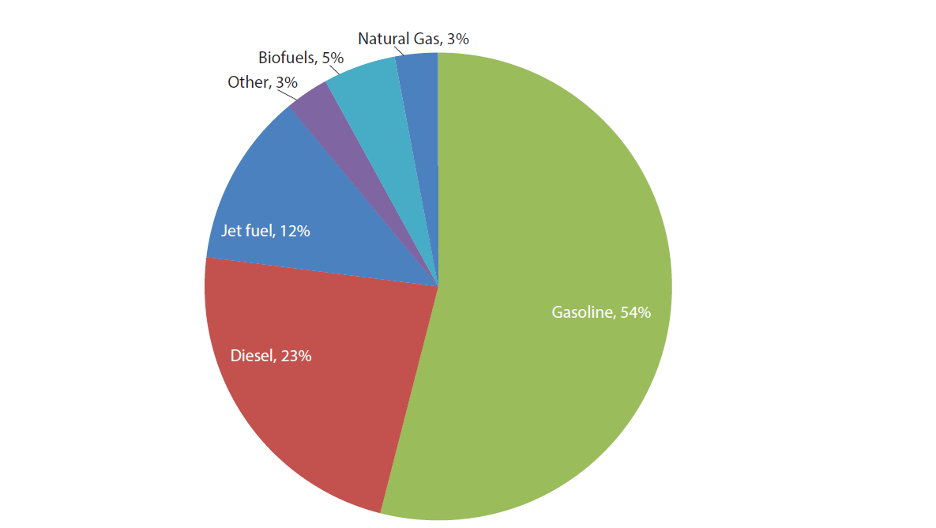
Life cycle analysis
Life cycle analysis is necessary for comparing emissions of different fuels. A life cycle analysis includes all emissions from extraction through combustion, including, for example, the energy from farm machinery and carbon released from soils when growing biofuels, emissions from the operation of refineries, and the transport of fuels in tankers, pipelines, and trucks.
Table 5.1 provides rough estimates of life cycle emissions of different vehicle-fuel combinations, compared with gasoline-powered internal combustion engine vehicles. Note that these life cycle emission comparisons (per kilometer) could vary considerably since they rely on a large number of assumptions. For example, GHG emissions for an electric vehicle depend on the carbon intensity of the electricity used to charge the vehicle. This varies widely across space and time, from close to zero carbon in regions powered predominately by nuclear and low-carbon renewable sources, to carbon emissions exceeding those from internal combustion engines in places where electricity is generated from coal.
In general, petroleum-based fuels are convenient fuels for vehicles, since they have high energy density (per unit of volume), are easily portable and refuel vehicles quickly (because they are liquid), and have energy infrastructure already in place. However, petroleum-based fuels have high GHG emissions and emit large quantities of conventional pollutants. As a society, we have grown dependent on petroleum and have become quite cost-efficient at extracting and refining fossil fuels, resulting in low prices.
In some cases, though, alternative fuels are demonstrably cheaper than petroleum, even in the US, where petroleum products tend to have lower prices than elsewhere. For example, as of 2019, a kilowatt hour costs about $0.12 in the US on average—equivalent to about 3–4 cents per mile, an energy cost about one-third that of gasoline-powered cars. In areas with low-carbon electricity, these electric vehicles also offer significant GHG emission savings.
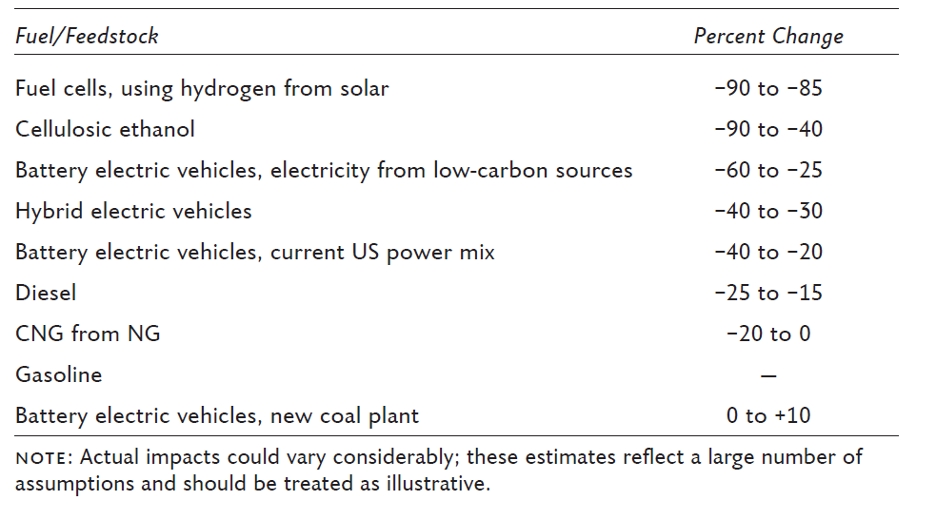
Fuel Use and Emissions from Road Traffic
Road transportation is a major consumer of energy and contributor to emissions of deleterious pollutants. Motorized vehicles are the second highest source of CO2 emissions in the United States. Transportation sources are causing 28% of total CO2 emissions in 2012 and 84% of that is from on road traffic (EPA, 2014). Emissions from anthropogenic sources, particularly burning of fossil fuels, is attributed as a key factor in increase in atmospheric CO2 concentration in recent years (Etheridge et al., 1996). In 2013, the United States consumed 97.1 quadrillion BTUs (Quads) of energy; 26.7 Quads (more than 25% of the total energy supply) were used in transportation sector. In doing so 173,493 million gallons of motor fuel was used including both gasoline and diesel (BTS, 2015). All major urban areas are experiencing widespread congestion due to increased demand of vehicular traffic (D. L. Schrank & Lomax, 2007). Yearly delay per commuter has increased to 42 hours in 2014 compared to 18 hours in 1982 resulting in a congestion cost of $160 billion (D. Schrank, Eisele, Lomax, & Bak, 2015). Increased demand for travel has caused increase in number of motorized vehicles, resulting in increased congestion on roadways. Drivers are facing frequent flow disruption and increased waiting time at intersections. These factors can lead to increased emission of deleterious pollutants such as carbon dioxide (CO2), carbon monoxide (CO), nitrogen oxides (NOx), hydrocarbons (HCs) (Oduyemi & Davidson, 1998). Oversaturation is the main contributor to predicted total emissions for CO and HC (Smit, 2006). Increased emission from congestion may be a result of more idling time, and more acceleration and deceleration events associated with stop and go conditions.
Techniques for reducing fuel use and emissions from road traffic
There have been many improvements in prevention and control of mobile source emissions in recent years such as modifying fuel, vehicle operation, engine design, behavior, regular maintenance, and most commonly by exhaust gas treatment. Improvements such as low sulfur fuels, computerized fuel metering, electronic ignition, air injection, exhaust gas recirculation, and 3-way catalytic converters have substantially reduced emissions on an individual vehicle basis (Faiz, Weaver, & Walsh, 1996). During hard acceleration events emissions of HC and CO can increase as vehicle engines operate in a fuel rich mode (Alkidas, 2007). Emissions of PM and HC can increase under deceleration due to the presence of unburned fuel (Cappiello, 2002). A common emissions mitigation measure considered by transportation planners is to enhance the capacity of the roadways. But the effect of capacity augmentation on reduction of emissions is not well quantified; such augmentation may increase induced travel resulting in quick decrease of initial emission reduction benefits (Noland & Quddus, 2006).
Many transportation planning and operations strategies are planned and being implemented to reduce this problem (Eliasson, Hultkrantz, Nerhagen, & Rosqvist, 2009; Tonne, Beevers, Armstrong, Kelly, & Wilkinson, 2008). Changing intersection design, traffic signal design, freeway metering technologies are some examples of operational strategies that are practiced (Greene & Plotkin, 2011). The United States Environmental Protection Agency (USEPA) is implementing ‘Tier 3 Motor Vehicle Emissions and Fuel Standard’ from 2017; which is going to be an important shift in standards for ‘regulatory classes’ that follows EPA’s adoption of ‘Tier 2’ program in 2000. By the year 2030, ‘Tier 3’ class of vehicles are expected to reduce onroad NOX, VOC, CO, SO2, Benzene emissions by 25%, 16%, 24%, 56%, 26% respectively. Furthermore, the phasing in of more stringent vehicle emission regulations, and fleet turnover to lower emitting vehicles, can be a factor in reducing on-road emissions of regulated pollutants such as CO, NOx, and HC.
In contrast to all the above mentioned techniques, an alternative and complementary approach could be the use of technologies to target the behavioral and system factors to improve the overall energy efficiency without altering the mechanical efficiency of each mode (car, bus, truck). Passenger vehicles run with 60% unutilized capacity (BTS, 2015); improving occupancy can make a big difference in total travel demand. Online taxi and ride sharing services such as Waze, Uber, RubyRide, Zipcar, Lyft has provided travelers with unique flexibility in terms of mode choice. Inefficient driving styles cause loss of 45% of the optimal fuel economy (Sivak & Schoettle, 2012). Emergence of communication technologies such as cellular and internet networks and social networks such as Facebook, Twitter has made it possible to influence travel behavior of individuals. Moreover, congestion arising from suboptimal route choice and oversaturation increases transportation energy use up to 33% (Roughgarden, 2012). Informed travel mode, route, and departure time choice is possible through real time travel information services such as Google Maps, INRIX and personalized navigation systems. A practical framework with real time response capability for monitoring, communicating, incentivizing, and controlling trip making and driving behavior attributes can make energy efficiency an integral part of the optimized transportation network.
Integrated Traffic Simulation and Energy Use-Emissions Estimation
Traffic simulation models at different levels of complexity and scales have been and are being developed for assessing energy use-emissions impact. To conduct project-level traffic environmental impact studies, microscopic emissions models are often adopted in transportation evaluation projects (Ahn, Rakha, Trani, & Van Aerde, 2002; Nam, Brazil, & Sutulo, 2002; Stathopoulos & Noland, 2003). Microscopic traffic simulation tools have been widely used to generate vehicle emissions estimates by evaluating driving speed and acceleration characteristics/profiles on a vehicle-by-vehicle and second-by-second basis. Although a high-fidelity traffic simulator is desirable for analyzing individual movement delays and facilities with complex geometric configurations, microscopic simulation can be computationally intensive and typically requires a wide range of detailed geometric data and driving behavior parameters, which can be difficult to calibrate, especially for the purpose of producing high fidelity emissions estimates. This has limited their applicability to small- and medium-scale corridors.
Alternatively, many organizations have utilized post-processing techniques for estimating vehicle emissions from their travel demand model results. Large scale air pollution maps are generally produced by using static estimates of average traffic and weather conditions. Most of the existing research for regional or city level emission assessment have used historic O-D matrices (Gualtieri & Tartaglia, 1998), land use transport models (Lautso & Toivanen, 1999), travel demand models (Karppinen et al., 2000), traffic assignment modules (Namdeo, Mitchell, & Dixon, 2002). These estimates lack the sensitivity of dynamic vehicular travel demand and cannot reflect temporal fluctuations of road conditions.
Recognizing that conventional static traffic assignment models are not sensitive to the dynamic interaction of vehicular travel demand and time-dependent road conditions, planning practitioners have increasingly recognized the capabilities of mesoscopic Dynamic Traffic Assignment (DTA) models. However, many planners and engineers are still concerned that DTA tools, typically based on fine-grained network representations, are computationally intensive and lack model components/details necessary for accurately representing high-fidelity traffic dynamics. Differences in resolution between traffic simulation and emissions estimation models is a barrier to integrating them into one framework. In recent years, a multi-resolution modeling approach has been exploited by many practitioners. Typically, this approach aims to integrate many existing simulation tools in a loosely coupled software platform that can provide multiple levels of modeling detail regarding network dynamics and traveler/driver choices. For example, in a subarea study, one can simply extract vehicle path data from a (macroscopic/mesoscopic) DTA tool for use in a microscopic simulation model (e.g. VISSIM, Paramics, TRANSIMS) to generate second-by-second vehicle speed and acceleration outputs for microscopic emissions or mobility-related analysis.
Estimation of emissions is dependent on the simulated driving activity such as instantaneous speeds and accelerations. Therefore, the accuracy of fuel use and emissions estimation hinge on the accuracy of traffic simulation. Traditional traffic simulations are focused on the mobility and safety aspects of the network. In contrast, traffic simulations to assess fuel use and emissions are focused on the capability of the model to emulate driving activity parameters properly. In addition to the fundamental difference in simulation purpose, a few functionality and compatibility issues are equally important in energy use and emissions estimation. The simulator framework can be visualized as Figure has two different modules namely a traffic simulation module and an emissions estimation module. Data flow between these two modules should be consistent and uninterrupted in ideal conditions.
There have been many efforts in the past to couple an emission model with a traffic simulator either manually or directly. AIMSUN has been used with a European modal emissions model, VERSIT+ (Ligterink & Lange, 2009). MOBILE6 emissions model has been coupled with EMME/2 and PARAMICS (Bartin et al., 2007). MOVES emission model has been used with PARAMICS, DynusT, and VISSIM (Lin, Chiu, Vallamsundar, & Bai, 2011b; Song et al., 2012; Xie et al., 2011). Dynamic linkage of traffic and emissions models is challenging and can lead to significantly longer run times. Evaluation for a large-scale network is a trade-off between estimation accuracy and computational tractability. Therefore, it is a challenge to properly estimate emissions-related impacts that is greatly exceeded by the imprecision and/or inaccuracy of the estimate.
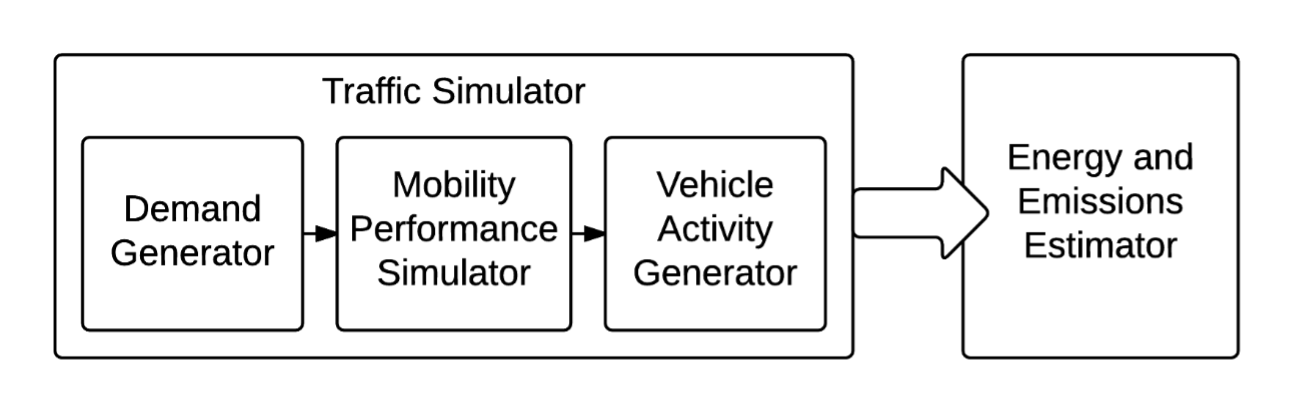
Multi-resolution modeling system such as a mesoscopic DTA has a relatively low simulation resolution (e.g. 6 second update interval), while microscopic traffic simulators typically use 0.1 seconds as the simulation interval. To ensure theoretical convergence of the integrated models, it is necessary to use multiple iterations between different simulation/assignment components to determine the mobility and emission impact of high-level demand and traveler behaviors. However, internal discrepancies between different modeling resolutions make tight interconnections and consistent modeling extremely challenging.
Energy use and emissions estimation
Effective tools to estimate emissions for different scenarios are required to assess the effect of these strategies at different spatial and temporal resolution. There are several emissions models available that can estimate vehicle emissions for the prediction and management of air pollution levels near roadways. These models use information on weather, fuel type, fleet composition, vehicle type, and activity schedule as input.
For planning purposes, average speed and flow based models have been used for a long time. However, these models cannot adequately represent the dynamic effects of driving styles. Average speed based models use predetermined speed trajectories upon which relationships between cycle or link-based average-speed and average emission rates are estimated (e.g. MOBILE (USEPA, 2007), EMFAC (CARB, 2002), COPERT (Ntziachristos et al., 2000)). US Environmental Protection Agency (EPA) have developed MOVES that can take into account a second-by-second vehicle speed trajectory (Chamberlin, Swanson, Talbot, Dumont, & Pesci, 2011). There are other models, such as CMEM (An, Barth, Norbeck, & Ross, 1997), that also consider operating modes in estimating emissions. Thus, MOVES is attractive in being able to represent a wide range of driving cycles for any user specified speed trajectory.
Vehicle specific power (VSP) based estimation
VSP is a well-evaluated and widely used quantitative indicator of engine power demand that is an excellent predictor of vehicle fuel use and that is also highly correlated with vehicle tailpipe exhaust emissions for a wide range of pollutants (Jimenez-Palacios, 1998). VSP is a function of vehicle speed, road grade, and acceleration which accounts for kinetic energy, rolling resistance, aerodynamic drag, and gravity (Zhai, Frey, Rouphail, Goncalves, & Farias, 2009). It is usually reported as power required per mass of the vehicle (for example: kilowatts per ton). Calculated VSP is categorized into different operating mode bins by speed and VSP ranges to estimate emissions factor for vehicles. Therefore, VSP is a parameter with important practical application. But accurate determination of VSP depends on proper quantification of measured vehicle operating characteristics, such as speed, acceleration, road grade etc. Microscopic traffic characteristics e.g. speed, acceleration, headway etc. are highly dependent on the roadway, traffic, driver behavior characteristics.
VSP is usually estimated using developed equations for different classes of vehicles. According to MOVES the equation to calculate VSP is expressed as
[latex]VSP=\left(\frac{A}{M}\right)v+\left(\frac{B}{M}\right)v^2+\left(\frac{C}{M}\right)v^3+\left(a+\sin{\left(\mathrm{\Phi}\right)}\right)v[/latex]
Where: A, B and C refer to the rolling term, rotating term and the drag term respectively. M is the vehicle mass, is the vehicle speed, a is vehicle acceleration and Φ is road grade. The parameters are different for each vehicle type.
MOVES provides default coefficients for different group of vehicles. Derivation of these coefficients is based on chassis dynamometer tests. The VSP formulation for light-weight vehicles provided by MOVES is
[latex]VSP=(A*v+B*v2+C*v3+m*v*a)/m[/latex]
VSP = vehicle specific power, kW/ton
v= speed at time t, m/s ; a = acceleration at time t, m/s2
A= rolling resistance coefficient = 0.1565 kW-sec/m
B= rotational resistance coefficient = 2.002X10-3 kW-sec2/m2
C= aerodynamic drag coefficient = 4.926X10-4 kW-sec3/m3
m = vehicle mass = 1.479 ton.
EPA MOVES model
On March 2, 2010, USEPA announced the official release of the Motor Vehicle Emissions Simulator (MOVES2010) for use in state implementation plan (SIP) submissions to EPA and regional emission analysis for transportation conformity (Koupal, Cumberworth, Michaels, Beardsley, & Brzezinski, 2002). It replaced MOBILE 6.2 model where vehicle emissions rates represent averages over a driving schedule with defined average speed. MOVES2010 considers the relative time spent and emissions rate in vehicle speed and vehicle specific power bins (Fujita et al., 2012). Except for braking and idling, these OpMode bins are stratified by 21 speed ranges (<25 mph, 25 to 50 mph, and >50 mph) and by Vehicle Specific Power (VSP) (Koupal, Michaels, Cumberworth, Bailey, & Brzezinski, 2002; Vallamsundar & Lin, 2011). The main purpose of this tool is to quantitatively predict emissions from mobile sources for a wide range of user-defined parameters e.g. vehicle type, time periods, geographical areas, pollutants, vehicle operating characteristics and road type (EPA, 2012). Therefore, MOVES is a significant improvement in the state-of-art for emissions estimation.
The inputs from traffic simulation software can be linked to MOVES in three different formats:
- Average speeds for the links of the network (similar to MOBILE)
- Link driving schedule (LDS) for each link of the network. LDS is a time dependent speed profile for a particular link. Generally LDS is selected for a representative vehicle or by sampling.
- Operating mode distribution of vehicles of the link.
However, MOVES is computationally intensive. Some investigators have attempted to use traffic simulation output for vehicle speed trajectories as input to MOVES, leading to time consuming computations for evaluation of different traffic management strategy scenarios.
Simplified emissions estimator – MOVESLite
As an alternative approach, a reduced form version of MOVES, referred to as MOVES Lite, has been recently developed (Frey & Liu, 2013). MOVES Lite is based on the same computational structure as MOVES with respect to Op Mode bins and, therefore, is capable of estimating emissions for any specified speed trajectory. MOVES Lite is less computational intensive than MOVES because it is calibrated to a base cycle and employs a cycle correction factor to adjust for differences in emission rates between any cycle of interest and the base cycle. MOVES Lite is based on a more limited set of vehicle types and pollutants than MOVES. Since traffic simulations are often for periods of a few hours, MOVES Lite does not take into account variations in factors such as fuel properties, inspection and maintenance programs, and ambient conditions that do not change substantially or at all during such short periods of time. MOVES Lite is 3,000 times faster and can produce emissions estimates within ±5% deviation compared to MOVES.
Because many factors where MOVES is sensitive are approximately constant during the time period of a typical simulation, there is no need to run MOVES in its entirety for every link in a network. Furthermore, because MOVES estimates emission factors based on weighted combinations of OpMode bins, a similar approach can be used as part of a simplified model that can be directly coded as part of a traffic simulation model. MOVESLite harnesses these benefits to develop a less computationally intensive vehicle emission estimation module. The conceptual model of MOVESLite is based on (a) base emission rate for site-specific characteristics (b) a cycle correction factor for speed trajectories and OpMode bin emission rates. The cycle correction factor is calculated using the following equation
[latex]{\rm CCF}_{p,c,a,v}=\left(\ \frac{\left(\sum_{m\ }{f_m^c\times{\rm ER}_{p,a,v,m}}\right)\ }{(\sum_{m\ }{f_m^b\times}{\rm ER}_{p,a,v,m})}\right)\left(\frac{V^b}{V^c}\right)[/latex]
Where,
ERp,a,v,m = default emission rate for pollutant p, age a, vehicle type v, in operating mode bin m, gram/hour
fm c = fraction of time in OpMode bin m in cycle c
fmb = fraction of time in OpMode bin m for base cycle b
Vc = cycle average speed for cycle c, mph
Vb = cycle average speed for base cycle b, mph
The base emissions rate is then corrected for the simulated cycle using the following equation
[latex]\mathrm{CE}_{\mathrm{p}, \mathrm{c}}=\sum_{\mathrm{v}}\left\{\left[\sum_{\mathrm{a}}\left(\mathrm{EF}_{\mathrm{p}, \mathrm{b}, \mathrm{a}, \mathrm{v}} \times \mathrm{CCF}_{\mathrm{p}, \mathrm{c}, \mathrm{a}, \mathrm{v}} \times \mathrm{f}_{\mathrm{a}, \mathrm{v}}\right)\right] \times \mathrm{f}_{\mathrm{v}}\right\}[/latex]
Where,
CE p,c, = cycle average emission factor for any arbitrary driving cycle c, for pollutant p, for a fleet of vehicles with mixed types and ages, gram/mi
ER p,b,a,v = base emission rate for base cycle b, age a, vehicle type v, and pollutant p, gram/mi
CCFp,c,a,v = cycle correction factor for driving cycle c, age a, vehicle type v, and pollutant p
fa,v = age fraction for age a and vehicle type v
fv = vehicle type fraction for vehicle type v
c = cycle c
b = base cycle
p = pollutant
Comparison of the 2 models across similar criteria shown in Table 5.2 and Table 5.3.
Criterion | MOVES |
---|---|
Accuracy | Accurate comparing with empirical emission factors. |
Runtime | Relatively slow. |
Requirement for Input | Substantial input data requirements. |
Requirement for Platform | Need to install MOVES package, JAVA, and MySQL. |
Connection with TDM and TSM | Difficult to be coupled into TDM or TSM. |
Usability | Errors, warnings arise frequently, especially for beginning users. |
Time consuming procedures | Adjust fuel property, temperature, humidity, air conditioning use, and I/M program for each link in the network. |
Vehicle dynamic data | Second by second data, or OpMode distribution |
Vehicle Types | 13 Vehicle types: Passenger Car, Passenger Truck, Refuse Truck, Single-Unit Short-Haul, Truck Single-Unit Long-Haul Truck, Motor Home, Intercity Bus, Transit Bus, School Bus, Combination Short-Haul Truck , Combination Long-Haul Truck, Motorcycle |
Adjusted emission rate map (reflecting vehicle distribution and climate) | Yes, took vehicle distribution and weather condition (temperature and humidity) into account. |
Criterion | MOVES lite |
---|---|
Accuracy | Within [latex]±[/latex]5% errors comparing with MOVES. |
Runtime | 3000 times faster than MOVES. |
Requirement for Input | Limited input data requirements. |
Requirement for Platform | Can be run in MS EXCEL or MATLAB. It has a computational algorithm. |
Connection with TDM and TSM | Can be integrated into TDM or TSM easily. |
Usability | User-friendly. |
Time consuming procedures | Set fuel property, temperature, humidity, air conditioning use, and I/M program constant by link in the network. |
Vehicle dynamic data | Same as MOVES |
Vehicle Types | For U.S. based model: Five vehicle types that comprise of more than 95% of the fleet: Passenger Cars, Passenger Trucks, Light Commercial Trucks, Single Unit Short Haul Trucks, and Combination Long Haul Trucks. |
Adjusted emission rate map (reflecting vehicle distribution and climate) | Yes, took vehicle distribution into account. |
Key Takeaways
- Different energy pathways exist to meet the same transportation needs.
- Combinations of vehicle technologies and fuel technologies result in change in total energy consumption and emissions.
- It is important to analyze the life-cycle of a vehicle-fuel combination to ascertain the energy efficiency of the technology.
- Microsimulation is a computaionally intensive way to estimate energy consumption from road traffic, macroscopic simulation may be too crude, and mesoscopic simulation is a balance between the two.
Self-Test
Glossary: Key Terms
Carbon intensity: A measure of carbon dioxide and other greenhouse gases (CO2e) per unit of activity, like generating a product
Energy density: The amount of energy stored in a given system, substance, or region of space per unit volume.
Energy efficient: Use of less energy to perform the same task or produce the same result.
Induced travel: Adding roadway capacity actually increases network-wide vehicle miles traveled (VMT) by a nearly equivalent proportion within a few years, reducing or negating any initial congestion relief. That increase in VMT is called “induced travel”
Media Attributions
Figures
- Figure 5.1: Bending the Curve: Climate Change Solutions by Ramanathan, V., Aines, R., Auffhammer, M., Barth, M., Cole, J., Forman, F., et al. is licensed under CC BY-NC-SA 4.0
- Figure 5.2: Average passenger car GHG emissions normalized by distance traveled for different regions by Ramanathan et al. (2019) is licensed under CC BY-NC-SA 4.0
- Figure 5.3: The number of electric vehicles (EVs) being introduced for different regions is licensed under CC BY-NC-SA 4.0
- Figure 5.4: Fuel utilization for the US transportation market, 2018 is licensed under CC BY-NC-SA 4.0
- Figure 5.5: Modules of integrated traffic simulation and emission estimation. Produced with permission from the author Tanvir (2018).
Tables
- Table 5.1: Greenhouse Gas Emissions per Kilometer, relative to Gasoline-Powered Internal Combustion Engines, Full Energy Cycle by Ramanathan, V., Aines, R., Auffhammer, M., Barth, M., Cole, J., Forman, F., et al. is licensed under CC BY-NC-SA 4.0
References
- Ahn, K., Rakha, H., Trani, A., & Van Aerde, M. (2002). Estimating vehicle fuel consumption and emissions based on instantaneous speed and acceleration levels. Journal of Transportation Engineering, 128(2), 182-190.
- Alkidas, A. C. (2007). Combustion advancements in gasoline engines. Energy Conversion and Management, 48(11), 2751-2761.
- An, F., Barth, M., Norbeck, J., & Ross, M. (1997). Development of comprehensive modal emissions model: Operating under hot-stabilized conditions. Transportation Research Record: Journal of the Transportation Research Board, 1587(1), 52-62.
- Barth, M., & Boriboonsomsin, K. (2009). Traffic congestion and greenhouse gases. ACCESS Magazine, 1(35)
- Bartin, B., Mudigonda, S., & Ozbay, K. (2007). Impact of electronic toll collection on air pollution levels: Estimation using microscopic simulation model of large-scale transportation network. Transportation Research Record: Journal of the Transportation Research Board, 2011(1), 68-77.
- BTS. (2015). State transportation statistics 2015. Washington DC: US Department of Transportation, Bureau of Transportation Statistics.
- Bureau of Transportation Statistics. (2013). National transportation statistics (table 1-11: Number of U.S. aircraft, vehicles, vessels, and other conveyances). Retrieved from http://www.bts.gov/publications/national_transportation_statistics/html/table_01_11.html
- Cambridge Systematics. (1996). Quantifying air quality and other benefits and costs of transportation control measures. ( No. Task 1 Report. Project 8-33.). Washington D.C: National Cooperative Highway Research, Transportation Research Board.
- Cappiello, A. (2002). Modeling traffic flow emissions (MS Thesis).
- CARB. (2002). EMFAC 2002, california air resources board’s emission inventory . ( No. Series, September).California Air Resource Board.
- Chamberlin, R., Swanson, B., Talbot, E., Dumont, J., & Pesci, S. (2011). Analysis of MOVES and CMEM for evaluating the emissions impact of an intersection control change. Paper presented at the Transportation Research Board 90th Annual Meeting, (11-0673)
- Daganzo, C. F. (2006). In traffic flow, cellular automata = kinematic waves. Transportation Research Part B: Methodological, 40(5), 396-403. doi://dx.doi.org.prox.lib.ncsu.edu/10.1016/j.trb.2005.05.004
- De Vlieger, I., De Keukeleere, D., & Kretzschmar, J. G. (2000). Environmental effects of driving behaviour and congestion related to passenger cars. Atmospheric Environment, 34(27), 4649-4655.
- Eliasson, J., Hultkrantz, L., Nerhagen, L., & Rosqvist, L. S. (2009). The stockholm congestion–charging trial 2006: Overview of effects. Transportation Research Part A: Policy and Practice, 43(3), 240-250.
- EPA. (1999). Regulatory announcements. Ann Arbor, MI: United States Environmental Protection Agency.
- EPA. (2008). Integrated science assessment for oxides of nitrogen – health criteria (final report). ( No. EPA/600/R-08/071). Washington D.C.: U.S. Environmental Protection Agency.
- EPA. (2010). Integrated science assessment for carbon monoxide (final report). ( No. EPA/600/R-09/019F). Washington D.C.: U.S. Environmental Protection Agency.
- EPA. (2012). User guide for MOVES2010b. ( No. EPA-420-B-12-001b).United State Environmental Protection Agency.
- EPA. (2014). Inventory of U.S. greenhouse gas emissions and sinks: 1990-2012. ( No. EPA 430-R-14-003). Washington D.C.: U.S. EPA.
- Etheridge, D. M., Steele, L. P., Langenfelds, R. L., Francey, R. J., Barnola, J., & Morgan, V. I. (1996). Natural and anthropogenic changes in atmospheric CO2 over the last 1000 years from air in antarctic ice and firn. Journal of Geophysical Research: Atmospheres (1984–2012), 101(D2), 4115-4128.
- Faiz, A., Weaver, C. S., & Walsh, M. P. (1996). Air pollutation from motor vehicles: Standards and technologies for controlling emissions World Bank-free PDF.
- Frey, H. C., Zhai, H., & Rouphail, N. M. (2009). Regional on-road vehicle running emissions modeling and evaluation for conventional and alternative vehicle technologies. Environmental Science & Technology, 43(21), 8449-8455. doi:10.1021/es900535s; 10.1021/es900535s
- Frey, H. C., & Liu, B. (2014). Development and evaluation of a simplified version of MOVES for coupling with a traffic simulation model. Proceedings, 91st Annual Meeting of the Transportation Research Board,
- Frey, H. C., Rouphail, N. M., Unal, A., & Colyar, J. D. (2001). No title. Emissions Reduction through Better Traffic Management: An Empirical Evaluation Based upon on-Road Measurements,
- Frey, H. C., Yazdani-Boroujeni, B., Hu, J., Liu, B., & Jiao, W. (2013). Field measurements of 1996 to 2013 model year light duty gasoline vehicles. Paper presented at the Proceedings, 106th Annual Conference, Air & Waste Management Association, Chicago, IL,
- Fujita, E. M., Campbell, D. E., Zielinska, B., Chow, J. C., Lindhjem, C. E., DenBleyker, A., . . . Lawson, D. R. (2012). Comparison of the MOVES2010a, MOBILE6. 2, and EMFAC2007 mobile source emission models with on-road traffic tunnel and remote sensing measurements. Journal of the Air & Waste Management Association, 62(10), 1134-1149.
- Gordon, P., Kumar, A., & Richardson, H. W. (1990). Peak-spreading: How much? Transportation Research Part A: General, 24(3), 165-175.
- Greene, D. L., & Plotkin, S. E. (2011). Reducing greenhouse gas emission from US transportation. Arlington: Pew Center on Global Climate Change,
- Gualtieri, G., & Tartaglia, M. (1998). Predicting urban traffic air pollution: A GIS framework. Transportation Research Part D: Transport and Environment, 3(5), 329-336.
- Health Effects Institute. Panel on the Health Effects of Traffic-Related Air Pollution. (2010). Traffic-related air pollution: A critical review of the literature on emissions, exposure, and health effects Health Effects Institute.
- Hurdle, V. F., & Son, B. (2000). Road test of a freeway model. Transportation Research Part A: Policy and Practice, 34(7), 537-564. doi://dx.doi.org.prox.lib.ncsu.edu/10.1016/S0965-8564(99)00031-2
- Jimenez-Palacios, J. L. (1998). Understanding and quantifying motor vehicle emissions with vehicle specific power and TILDAS remote sensing
- Karppinen, A., Kukkonen, J., Elolähde, T., Konttinen, M., Koskentalo, T., & Rantakrans, E. (2000). A modelling system for predicting urban air pollution: Model description and applications in the helsinki metropolitan area. Atmospheric Environment, 34(22), 3723-3733.
- Koupal, J., Cumberworth, M., Michaels, H., Beardsley, M., & Brzezinski, D. (2002). Design and implementation of MOVES: EPA‘s new generation mobile source emission model. Ann Arbor, 1001, 48105.
- Koupal, J., Michaels, H., Cumberworth, M., Bailey, C., & Brzezinski, D. (2002). (2002). EPA’s plan for MOVES: A comprehensive mobile source emissions model. Paper presented at the Proceedings of the 12th CRC on-Road Vehicle Emissions Workshop, San Diego, CA,
- Krzyzanowski, M., Kuna-Dibbert, B., & Schneider, J. (2005). Health effects of transport-related air pollution World Health Organization Copenhagen.
- Lautso, K., & Toivanen, S. (1999). SPARTACUS system for analyzing urban sustainability. Transportation Research Record: Journal of the Transportation Research Board, 1670(1), 35-46.
- Ligterink, N. E., & Lange, R. d. (2009). Refined vehicle and driving-behaviour dependencies in the VERSIT emission model. Paper presented at the ETAPP Symposium,
- Lin, J., Chiu, Y., Vallamsundar, S., & Bai, S. (2011a). Integration of MOVES and dynamic traffic assignment models for fine-grained transportation and air quality analyses. Paper presented at the Integrated and Sustainable Transportation System (FISTS), 2011 IEEE Forum On, 176-181.
- Lin, J., Chiu, Y., Vallamsundar, S., & Bai, S. (2011b). Integration of MOVES and dynamic traffic assignment models for fine-grained transportation and air quality analyses. Paper presented at the Integrated and Sustainable Transportation System (FISTS), 2011 IEEE Forum On, 176-181.
- Lomax, T., Turner, S., Shunk, G., Levinson, H. S., Pratt, R. H., Bay, P. N., & Douglas, G. B. (1997). NCHRP report 398: Quantifying congestion. Transportation Research Board, National Research Council, Washington, DC,
- Mahmassani, H. S., & Liu, Y. (1999). Dynamics of commuting decision behaviour under advanced traveller information systems. Transportation Research Part C: Emerging Technologies, 7(2), 91-107.
- Meyer, M. D. (1999). Demand management as an element of transportation policy: Using carrots and sticks to influence travel behavior. Transportation Research Part A: Policy and Practice, 33(7), 575-599.
- Mitchell, G., Namdeo, A., Lockyer, J., & May, A. D. (2002). The impact of road pricing and other strategic road transport initiatives on urban air quality. Paper presented at the Proceedings of the 8th International Conference on Urban Transport and the Environment in the 21st Century, 481-490.
- Nam, E. K., Brazil, H., & Sutulo, S. (2002) The integration of a fuel rate based modal emissions model (CMEM modified) into the VISSIM microscopic traffic model – inventory comparison with MOBILE6 in southfield michigan. . Proceedings of the 12th CRC on-Road Vehicle Emissions Workshop,
- Namdeo, A., Mitchell, G., & Dixon, R. (2002). TEMMS: An integrated package for modelling and mapping urban traffic emissions and air quality. Environmental Modelling & Software, 17(2), 177-188.
- Newell, G. F. (1993). A simplified theory of kinematic waves in highway traffic: (I) general theory; (ii) queuing at freeway bottlenecks; (iii) multi-dimensional flows . Transportation Research Part B: Methodological, 27(4), 281-313.
- Newell, G. F. (2002). A simplified car-following theory: A lower order model. Transportation Research Part B: Methodological, 36(3), 195-205.
- Ni, D., Leonard, J. D., & Williams, B. M. (2006). The network kinematic waves model: A simplified approach to network traffic. Journal of Intelligent Transportation Systems, 10(1), 1-14.
- Noland, R. B., & Quddus, M. A. (2006). Flow improvements and vehicle emissions: Effects of trip generation and emission control technology. Transportation Research Part D: Transport and Environment, 11(1), 1-14.
- Ntziachristos, L., Samaras, Z., Eggleston, S., Gorissen, N., Hassel, D., & Hickman, A. J. (2000). Copert iii. Computer Programme to Calculate Emissions from Road Transport, Methodology and Emission Factors (Version 2.1), European Energy Agency (EEA), Copenhagen,
- Oduyemi, K., & Davidson, B. (1998). The impacts of road traffic management on urban air quality. Science of the Total Environment, 218(1), 59-66.
- Ramanathan, V., Aines, R., Auffhammer, M., Barth, M., Cole, J., Forman, F., et al. (2019). Bending the Curve: Climate Change Solutions. Location: Regents of the University of California. Editor: V. Ramanathan. Co-Editors: Adam Millard-Ball; Michelle Niemann; Scott Friese. Book published by the Regents of the Univ of California. Retrieved from https://escholarship.org/uc/item/6kr8p5rq. 815pp https://creativecommons.org/licenses/by-nc-sa/4.0/
- Roughgarden, T. (2012). The price of anarchy in games of incomplete information. Paper presented at the Proceedings of the 13th ACM Conference on Electronic Commerce, 862-879.
- Samaranayake, S., Glaser, S., Holstius, D., Monteil, J., Tracton, K., Seto, E., & Bayen, A. (2014). Real‐Time estimation of pollution emissions and dispersion from highway traffic. Computer‐Aided Civil and Infrastructure Engineering, 29(7), 546-558.
- Schrank, D. L., & Lomax, T. J. (2007). The 2007 urban mobility report Texas Transportation Institute, Texas A & M University.
- Schrank, D., Eisele, B., Lomax, T., & Bak, J. (2015). 2015 urban mobility scorecard. Texas: Texas A&M Transportation Institute and INRIX, Inc.
- Sivak, M., & Schoettle, B. (2012). Eco-driving: Strategic, tactical, and operational decisions of the driver that influence vehicle fuel economy. Transport Policy, 22, 96-99.
- Sjodin, A., Persson, K., Andreasson, K., Arlander, B., & Galle, B. (1998). On-road emission factors derived from measurements in a traffic tunnel. International Journal of Vehicle Design, 20(1), 147-158.
- Smit, R. (2006). An examination of congestion in road traffic emission models and their application to urban road networks
- Song, G., Yu, L., & Zhang, Y. (2012). Applicability of traffic microsimulation models in vehicle emissions estimates. Transportation Research Record: Journal of the Transportation Research Board, 2270(1), 132-141.
- Stathopoulos, F. G., & Noland, R. B. (2003). Induced travel and emissions from traffic flow improvement projects. Transportation Research Record: Journal of the Transportation Research Board, 1842(1), 57-63.
- Tanvir, S., Karmakar, N., Rouphail, N. M., & Schroeder, B. J. (2016). Modeling freeway work zones with mesoscopic dynamic traffic simulator: Validation, gaps, and guidance. Transportation Research Record: Journal of the Transportation Research Board, (2567), 122-130.
- Tanvir, Shams. Modeling and Simulation of Driving Activity from an Energy Use-Emissions Perspective. North Carolina State University, 2018.
- Tonne, C., Beevers, S., Armstrong, B., Kelly, F., & Wilkinson, P. (2008). Air pollution and mortality benefits of the london congestion charge: Spatial and socioeconomic inequalities. Occupational and Environmental Medicine, 65(9), 620-627.
- Twigg, M. V. (2007). Progress and future challenges in controlling automotive exhaust gas emissions. Applied Catalysis B: Environmental, 70(1), 2-15.
- USEPA. (2007). MOBILE 6 vehicle emission modelling software and documentation. (). Washington, DC: US Environmental Protection Agency.
- Vallamsundar, S., & Lin, J. (. (2011). MOVES versus MOBILE. Transportation Research Record: Journal of the Transportation Research Board, 2233(1), 27-35.
- Xie, Y., Chowdhury, M. A., Bhavsar, P., & Zhou, Y. (2011). An integrated tool for modeling the impact of alternative fueled vehicles on traffic emissions: A case study of greenville, south carolina. Paper presented at the Transportation Research Board 90th Annual Meeting, (11-3880)
- Yperman, I. (2007). The link transmission model for dynamic network loading (Ph.D. Thesis).
- Zhai, H., Frey, H. C., Rouphail, N. M., Goncalves, G. A., & Farias, T. L. (2009). Comparison of flexible fuel vehicle and life-cycle fuel consumption and emissions of selected pollutants and greenhouse gases for ethanol 85 versus gasoline. Journal of the Air & Waste Management Association (1995), 59(8), 912-924.
- Zhang, K., & Batterman, S. A. (2009). Time allocation shifts and pollutant exposure due to traffic congestion: An analysis using the national human activity pattern survey. Science of the Total Environment, 407(21), 5493-5500.
- Zhou, X., Tanvir, S., Lei, H., Taylor, J., Liu, B., Rouphail, N. M., & Christopher Frey, H. (2015). Integrating a simplified emission estimation model and mesoscopic dynamic traffic simulator to efficiently evaluate emission impacts of traffic management strategies. Transportation Research Part D: Transport and Environment, 37, 123-136. doi://dx.doi.org/10.1016/j.trd.2015.04.013
Attributions
- Bending the Curve: Climate Change Solutions by Ramanathan, V., Aines, R., Auffhammer, M., Barth, M., Cole, J., Forman, F., et al. is licensed under CC BY-NC-SA 4.0
A measure of carbon dioxide and other greenhouse gases (CO2e) per unit of activity, like generating a product
Use of less energy to perform the same task or produce the same result.
The amount of energy stored in a given system, substance, or region of space per unit volume.
Adding roadway capacity actually increases network-wide vehicle miles traveled (VMT) by a nearly equivalent proportion within a few years, reducing or negating any initial congestion relief. That increase in VMT is called “induced travel”