Chapter 8: Shared Mobility and Automation
Chapter Overview
In this chapter, we discuss the potential of vehicle sharing and automation to minimize the need for additional vehicles on the road and eventually reduce vehicle miles traveled (VMT). However, the focus of this chapter is to look at the unintended consequences of these technologies; specifically, the ‘rebound effect’ or the ‘induced demand’. Additionally, we will elucidate on the inefficient operation, inequitable distribution, and inadequate infrastructure related to these technologies.
Chapter Topics
- Shared Mobility
- Automation in Transportation
- Induced Demand from Shared Automated Transportation
- VMT Reduction Methods
- Transportation Efficiency Improvement
- Effect of Vehicle Automation
Learning Objectives
At the end of the chapter, the reader should be able to do the following:
- Design curb space for shared automated fleets.
- Develop a framework to analyze sustainability of any emerging transportation technology based on first principles.
- Apply the concept of induced demand to calculate the short-term activity change and the long-term behavioral changes stemmed from adoption of emerging transportation technologies.
Shared Mobility
The revolution of shared mobility started with the concept of On-Demand Mobility Services. On-demand mobility services involves arranging for travel on-demand. Usually, the travelers request their “rides” on automobile or paratransit using a smartphone app. It is to be noted that on-demand mobility existed before smartphones and the smartphone-based app, and in many parts of the US and the world, especially rural areas, it still operates without it. The service provider typically manages a fleet of vehicles and optimizes the vehicles’ locations and route assignments based on the dynamic demand of subscribers. Greenblatt and Shaheen (2017) classified on-demand mobility services in four major categories – transport network companies (TNCs), carsharing, ridesharing, and e-hailing.
TNCs such as Uber and Lyft match drivers who subscribe to their platform with trip requests from individuals. Most of the trip requests are from single travelers; therefore, the majority of the TNC trips are not shared rides. For example, according to a recent study in Boston the average occupancy rate for TNC trips is 1.5, including the driver. Average occupancy for TNC trips can have a value less than 2 since there are many empty trips without passengers made in shared mobility systems.To promote ridesharing, major TNCs offer shared ride service options such as UberPOOL and Lyft Line in major cities. However, only a small fraction of users who have access to these shared ride options request them, and even a smaller fraction of those trip requests are actually matched as shared rides.
Carsharing is subscription-based mobility where subscribers pay a short-term fee. Zipcar is an example of typical carsharing services. On the other hand, the traditional carpooling and vanpooling fall under the ridesharing category. Ridesharing through microtransit services such as Via, Chariot, and Flex is considered a potential mobility solution in highly dispersed urban areas. E-hailing services matches traditional for-hire taxis to the nearest passengers using apps similar to TNCs. As in the case of TNCs, these on-demand mobility services only reduce the total number of trips when shared rides happen.
Although on-demand mobility services include other modes such as bicycle and scooter, we have purposefully limited the discussion in this chapter to exclude those modes. Additionally, paratransit services are on-demand as well, as are airport shuttles, and shared ride services common in the Global South. We assume that mobility decisions taken by the users of those modes will not significantly impact vehicle emissions (e.g., through mode shift). In addition, TNCs will be a focal point of our discussion regarding the impacts of on-demand mobility services because the current market share of all the other categories combined is inappreciable compared to that of TNCs.
Automation in Transporation
The US personal mobility market is valued at around one trillion dollars (Walker and Johnson, 2016). In recent years, the promises of sensing, communication, and artificial intelligence technologies have attracted billions of dollars in investments to develop connected and automated vehicles. These investments were predominantly made by ‘Silicon Valley’ companies, and since almost all the traditional automotive manufacturers have followed suit.
The Society of Automotive Engineers (SAE) defines five levels of driving automation in its J3016 taxonomy (SAE, 2016). The levels increase from 0 to 5 with a higher level representing lesser intervention and attention from the human driver. Level 3 to level 5 automation, according to SAE, represents automated driving features such as traffic jam chauffeur, local driverless taxi, and steering wheel-free driving.
Connectivity allows for the exchange of information between vehicle and infrastructure. Information exchange among vehicles, termed as vehicle-to-vehicle (V2V) communication has enabled several applications in the safety, mobility, and environmental domains. Examples of such applications are forward collision warning (safety), advanced traveler information (mobility), and eco-driving (environment). Vehicle to infrastructure (V2I) communication has enabled additional applications such as red-light violation warning (safety), transit signal priority (mobility), and eco-approach and departure (environment) . The US National Highway Traffic Safety Administration (NHTSA) proposed inclusion of V2V connectivity in all new-light duty vehicles and trucks in 2016.
Induced Demand from Shared Automated Transportation
On-demand mobility services, especially those offered by TNCs, operate on a premise that people will not own vehicles, and rather use these services to meet their travel needs. In the case of TNCs, users often spend time by curbside waiting for the vehicles to pick them up. This could translate to an increase in the amount of time spent in the microenvironment with a higher level of traffic-related air pollution as compared to using personal vehicles. The extent of the increase in exposure to traffic emissions will depend on a number of factors such as the frequency of service use, the pick-up locations, and the level of traffic emissions at those locations. The literature on this increased level of exposure for TNC users is still evolving as the service models continue to develop.
Current TNC operation is primarily based on existing vehicle and fuel technologies and is expected to remain the same in the near future. However, TNCs are likely to be early adopters of fuel-efficient vehicle and alternative fuel technologies as fuel cost accounts for a significant portion of their total operation costs. Fleet vehicles are conducive towards electric technologies because mileage radius is limited, trip length is limited, and they need to come back to the same place (or can access fixed hubs enroute), where the owner can install a charging station. Hu et al. (2018) studied New York city taxi trips and found that 94% of TNC shifts needed to serve those trips are shorter than 200 miles. Considering the range of current electric vehicles in the market, TNC drivers can practically switch to electric vehicles.
The research on the vehicle miles traveled (VMT) and subsequent emissions reduction potential is still evolving. Some localities and states like California are beginning to require TNCs to report more data to regulators. Additionally, it is not clear how the operational model for TNC would evolve in a post-COVID-19 pandemic scenario as the private venture capitalists backing the TNC sector focus more on the profitability of their operations. Alonso-Mora et al. (2017) compared 14,000 New York city taxi trips with a hypothetical ride-sharing operation. They found that 98% of the total taxi trips could be replaced by 3,000 ride-share vehicles with capacity of 4 passengers per vehicle or 2,000 vehicles with 10 passengers per vehicle. Bischoff and Maciejewski (2016) simulated trips from 1.1 million personal vehicles in the city of Berlin, Germany, and found that a fleet of 100,000 shared autonomous vehicles would be able to serve those trips sufficiently. In these simulation studies, no barriers to adoption of ride sharing were assumed and ,therefore, they represent an ideal scenario.
Evidence of the real-world impacts of TNCs, especially on VMT and congestion, has begun to emerge recently. In the short term, TNCs can incur a significant amount of ”deadhead” miles, which are the miles where the driver is driving without any passenger, such as from the drop-off location of one trip to the pick-up location of the next trip (Wenzel et al. 2019). A recent paper (Komanduri et al. 2018) using RideAustin data estimated that 37% of all the miles are due to deadheading. It was estimated to be around 20% in San Francisco (Castiglione et al, 2018) and about 50% in New York City (Schaller, 2018). Castillo et al. (2018) presented a condition of matching failure by TNCs where there are fewer number of available vehicles nearby than there are trip requests. Such failure may contribute to a substantially increased amount of deadhead miles. The number of these empty passenger trips can be reduced significantly by imposing an adaptive matching radii within which the driver supply and trip demand matching must occur (Xu et al., 2019).
In longer term, TNCs may complement public transit by providing first and last mile services, or decrease transit ridership by competing. Hall et al. (2018) found that transit ridership decreased by 5.9% in smaller cities but increased by 0.8% in larger cities due to the entry of Uber. Also, small transit agencies in big cities received the most complementing effect from Uber. Clewlow and Mishra (2017) surveyed more than 4,000 residents in 7 large cities in the US and found that 15% of public transit, 17% walk, and 7% bike trips were replaced by TNC trips, and that induced trips—trips which did not have happened if TNC service was not there—consisted of 22% of the total trips. A similar study by Henao and Marshall (2018) conducted in Denver, Colorado, found that public transit trips replaced by TNCs and induced TNC trips were 22% and 12%, respectively.
Evidence to date has pointed in the direction that TNCs, in the way they are operated and used currently, increase VMT, which has also resulted in increased traffic congestion in some areas. As a result, traffic-related emissions will likely also increase. However, it should be noted that any increase in VMT caused by TNCs will not increase tailpipe emissions if those miles are zero-emission miles. And if TNCs’ zero-emission miles can displace some of the personal vehicles’ gasoline miles, there can be a net reduction in total traffic emissions. For example, Bauer et al. (2018) showed that a centrally managed shared autonomous electric taxi fleet in Manhattan Island of New York City would yield 58% reduction in energy consumption and 73% reduction in greenhouse gas (GHG) emissions as compared to an autonomous fleet of conventional gasoline vehicles. Note that the New York power grid is fueled by higher proportion of clean fuel source such as hydroelectric, solar, and nuclear. However, localities with higher level of reliance on fossil fuels will not achieve such high levels of GHG reduction as reported by Bauer et al. (2018).
In terms of equity point of view, there is a large variation in the usage of TNCs among population in different income groups. According to the 2017 National Household Travel Survey (NHTS) in 9 major metropolitan areas, people with annual income more than $200,000 made 44 TNC trips per year, whereas people with annual income less than $15,000 made only 6 TNC trips. TNCs cost significantly more than taking public transit, so it is not surprising they are serving a wealthier clientele. TNCs also require a smart phone and bank account to use, which will limit use for some riders. Thus, the increase in traffic emissions generated by TNCs are contributed more by high-income population. It is unclear, however, how the air quality and health impacts of those increased emissions are distributed in the environmental justice context.
While the real-world testing and demonstration of connected and automated vehicles (CAVs) to date have shown potential for emissions reduction in the near term, primarily as a result of improved vehicle and traffic operational efficiency, the long-term impacts of these technologies on traffic emissions are unclear as there is no real-world evidence at this time. One speculative long-term impact is that the expanded and inexpensive mobility provided by CAVs may cause increased trip frequency and, consequently, higher VMT. The price competitiveness nature of an automated operation of TNCs may lead to the substitution of TNC trips for public transit trips. Middle to lower-income riders find these automated TNCs or robo-taxis to be competitive with public transit. However, the mode shift depends on the level of price reduction. For low-ridership local transit routes, the impact may not reduce ridership greatly, but for medium to high-ridership routes, this competition could lead to significantly more VMT if left unregulated. Regulation of VMT may involve adding taxes or fees for using a CAV, and/or congestion pricing or cordon pricing on highways. Wadud et al. (2016) estimated VMT increase of 4% -60% due to the reduced cost of drivers’ time. Meyer et al. (2017) suggested that autonomous vehicles could increase accessibility in suburban areas by 10% to 14%. Such increase in accessibility may translate to urban sprawl and result in increased trip length. These uncertain yet potentially significant impacts of CAVs may result in an overall increase in emissions in the long run.
VMT Reduction Methods
As described earlier, total VMT in the US continues to grow at a steady pace. VMT was flat from 2008 to 2012, primarily because of the economic recession, but has been increasing since then.
In terms of potentially reducing VMT, we can refer back to a variety of mobility measures outlined in previous chapters. In general, these include the following:
- Use pricing mechanisms to encourage users to reduce the number and distance of their trips and increase the number of passengers per vehicle. Several regions across the US are already increasing the number of toll and commuter lanes on their roadway networks, while cities such as Singapore, London, and Stockholm have implemented cordon pricing schemes that charge drivers to enter the city center.
- Provide incentives for using alternative modes such as transit and biking, as well as shifting work locations and schedules, for instance by telecommuting.
- Reduce urban sprawl, increase land use densities, and improve the mix of jobs and housing.
Transportation Efficiency Improvement
Another important strategy for reducing emissions from transportation is to improve the efficiency of transportation system operations. As described above, today’s transportation systems are often congested, which wastes time, money, and fuel. This wasted fuel translates to increased pollutant and GHG emissions. The 2023 Urban Mobility Report published by the Texas A&M Transportation Institute found that traffic congestion caused 3.3 billion gallons of wasted fuel on the US roads in 2022. This wasted fuel accounts for more than 2% of total gasoline usage in the country. Over the last several decades, a number of intelligent transportation system ( ITS) techniques are increasingly utilized that are squarely aimed at reducing these environmental impacts. ITS techniques and applications target three general areas: (1) congestion mitigation (for example, advanced signal control, predictive ramp metering, incident management), whereby congestion is reduced and speeds increased; (2) better management of speeds for different roadway types, using techniques such as variable speed limits and intelligent speed adaptation; and (3) smoothing of traffic by using techniques such as cooperative adaptive cruise control and speed harmonization. These “eco-friendly” intelligent transportation system technologies are typically categorized into three areas: vehicle systems, traffic management systems, and travel information systems.
Vehicle Systems Improvement
Vehicle systems represent vehicle features and functions that allow a vehicle to “see,” respond to, and communicate with its surroundings. Sensors such as on-board radar and computer vision technologies enable a vehicle to monitor the distance to the vehicle in front and to detect when a vehicle is leaving a lane, and they support adaptive cruise control systems that allow a driver to select a desired speed and set a following distance. In addition, communication devices (for example, dedicated short-range communications, cellular) will likely be deployed to enable vehicle-to-vehicle, vehicle-to-infrastructure, and infrastructureto- vehicle applications that are primarily focused on improving safety. It is important to point out that improved anticollision systems may have a significant indirect energy and emissions savings: fewer crashes result in less congestion, allowing for higher average traffic speeds with less stop and go. In addition to safety applications, a variety of mobility and environmental applications have also emerged, as illustrated in Table 8.1. These applications take advantage of connected vehicle technology such as cooperative adaptive cruise control where vehicles communicate with each other to cooperatively manage following distance, braking, accelerating, and more. These technologies are allowing vehicles to become increasingly automated, with the possibility of full vehicle automation coming in the next decade.
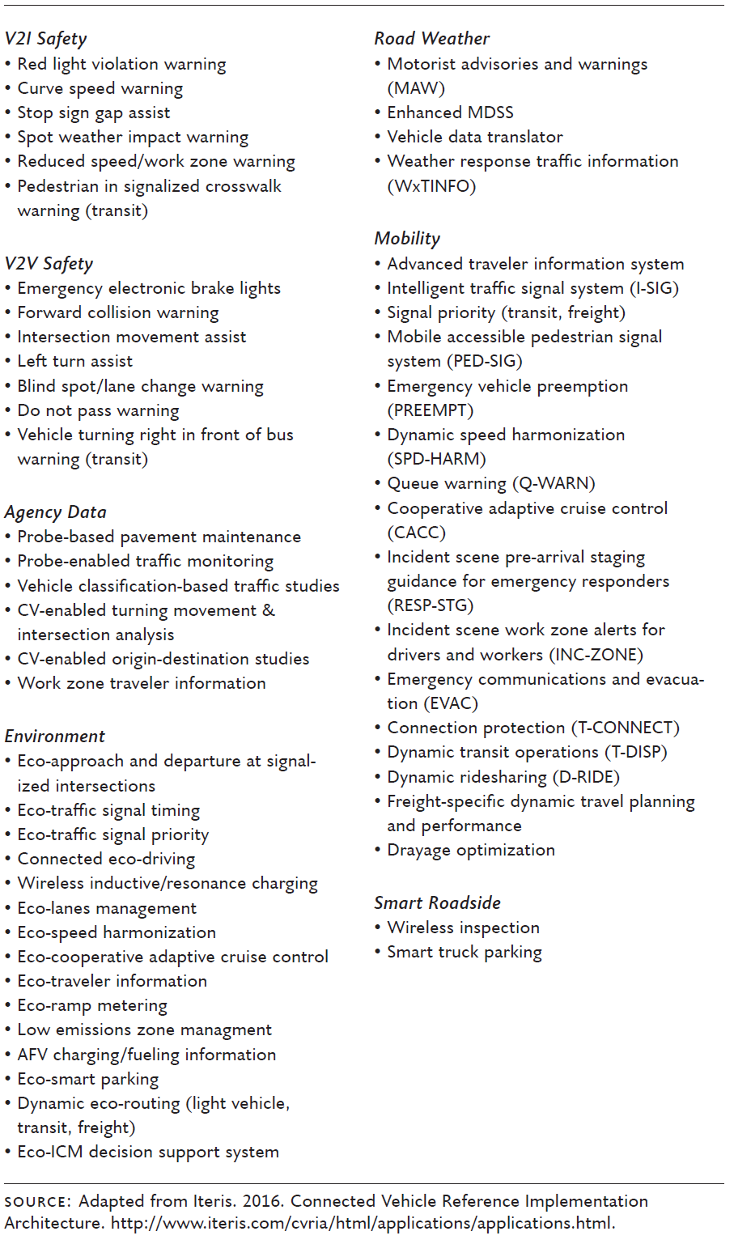
Traffic Management Systems Improvement
Traffic management systems have become more sophisticated with the advent of better sensor technology, more reliable communication channels, and advanced information processing. Transportation managers are better equipped to estimate traffic conditions, detect and remove traffic incidents, and craft better travel demand management strategies (that is, manage the number of vehicles on a congested roadway). The overarching goal of traffic management is to take full advantage of the existing roadway capacity, thus keeping traffic flowing smoothly at moderate speeds. In doing so, it will have a large impact on energy consumption and GHG emissions related to traffic. In addition, traffic management system strategies go even further by reducing the number of vehicles and VMT in the transportation network without compromising overall travel needs, thereby reducing the total contributions of energy consumption and emissions from the transportation sector.
Travel Information Systems Improvement
Travel information systems provide information to drivers, such as route guidance systems, geolocation systems, and electronic payment systems. All of these systems add convenience to the traveler while reducing energy consumption and emissions. For example, a route guidance system will cut back on unnecessary travel that may occur when a driver gets lost or chooses a long, out-of-the-way path. En route driver information can reduce energy and emissions associated with driving around in search of a specific location or parking. Electronic payment systems also eliminate the need for a driver to decelerate the vehicle, idle while a manual transaction takes place, and then accelerate the vehicle back to a desired speed. If this payment can occur without slowing down, energy consumption and emissions are greatly reduced.
In general, environmentally friendly ITS applications (that is, specific ITS applications that reduce energy and emissions) have slowly been emerging over the last decade, as have safety and mobility programs mentioned in Table 8.1. Pioneering research programs in the US, the European Union, and other regions have made significant progress in developing and testing these ITS applications and technologies with a focus on environmental benefits. From these research programs, it is clear that specific environmental benefits can be maximized when different ITS applications are “tuned” so that emissions and energy consumption are reduced. The actual energy and emissions savings vary, but they are typically on the order of 5% to 20%. It is important to point out that there is not a single ITS technology solution that has demonstrated a large reduction in energy consumption and emissions. But since most of these applications are additive, greater benefits may be achieved when a combination of environmentally friendly ITS programs is put into place.
Effect of Vehicle Automation
In recent years, interest in vehicle automation has soared. Some reports have predicted that vehicles could be fully automated (that is, not requiring a driver) by as early as 2025, though this is highly unlikely other than in tightly bounded areas with easy driving conditions. As an extension of ITS, vehicle automation could have both positive and negative effects on society (Figure 8.1). The bounds on the image are created by different automation scenarios ran by the authors of the paper. Vehicle automation could lead to reduced emissions, due to congestion reduction (for example, crash avoidance, platooning), traffic smoothing (for example, cooperative adaptive cruise control), and better speed management (for example, speed harmonization). Indeed, eco-driving behaviors could be directly programmed into the automated vehicle operation. On the other hand, vehicle automation could potentially increase emissions by increasing vehicle travel. People might use their automated vehicles for additional purposes or choose a more distant place to live, since the time cost of travel would be reduced. Automated vehicles could be used by a wider range of users, including youth and elderly. “Drop-off” errands might increase, resulting in new empty vehicle relocation trips, such as returning home without any passengers. Some early conclusions regarding automated vehicles include the following:
- Partial and full automation can reduce energy use and emissions, but only if incentives exist to encourage pooled use of vehicles.
- Automated vehicles that communicate and coordinate with other vehicles and the infrastructure will likely have greater improvements in safety, mobility, and the environment compared with autonomous vehicles without those capabilities.
- Automated vehicles have the strong potential to induce travel demand, unless incentives exist for pooled use of the vehicles.
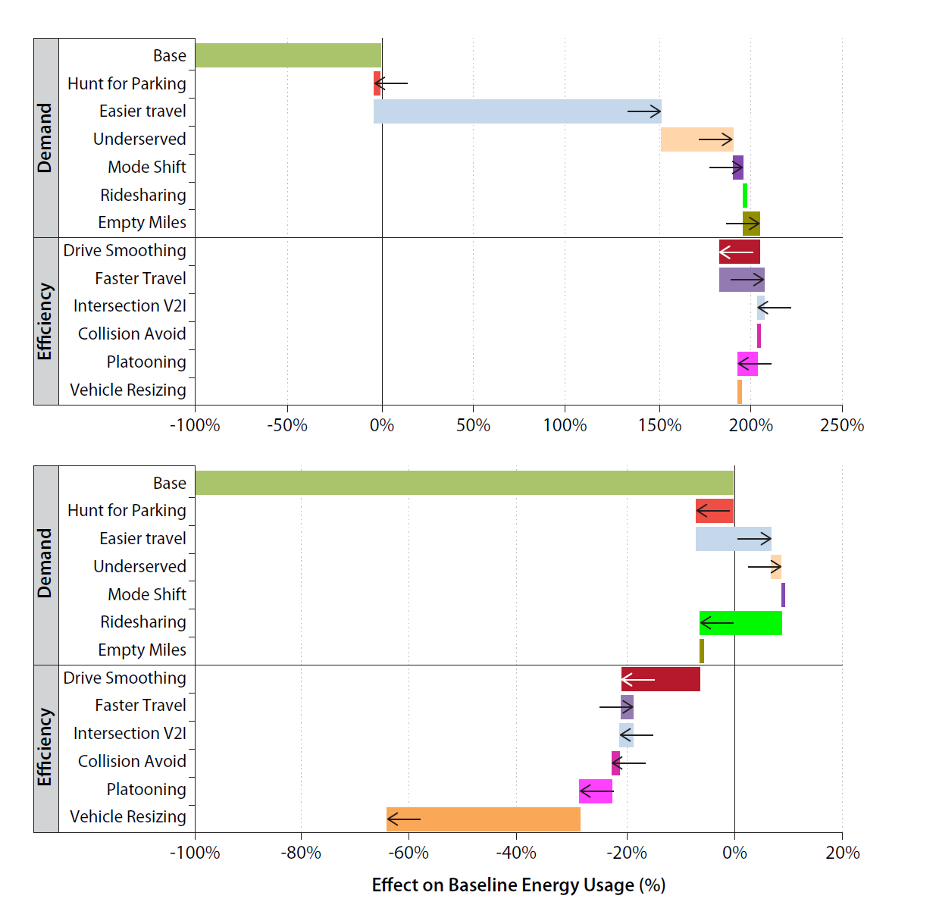
Key Takeaways
- There are generally four different ways to mitigate transportation emissions: through vehicle technology, low-carbon fuels, VMT management, and intelligent transportation systems, including the use of connected and automated vehicles.
- VMT can be reduced by increasing the use of pooled travel (for example, buses, transit, shared mobility that is pooled).
- Other VMT reduction methods may include adopting incentives and disincentives to reflect full social costs of travel and, eventually, transitioning from individual vehicle ownership to use of mobility services that are pooled.
- Vehicle automation is likely to be deployed in the near future and should be managed so as to achieve environmental sustainability.
Self-Test
Glossary: Key Terms
Cooperative Adaptive Cruise Control (CACC): an extension to the adaptive cruise control (ACC) concept using Vehicle-to-Everything (V2X) communication. Adaptive cruise control (ACC) is a type of advanced driver-assistance system for road vehicles that automatically adjusts the vehicle speed to maintain a safe distance from vehicles ahead.
Intelligent Transportation System ( ITS): an advanced application which aims to provide innovative services relating to different modes of transport and traffic management and enable users to be better informed and make safer, more coordinated, and ‘smarter’ use of transport networks.
On-Demand Mobility Services: On-demand mobility services are transport services that are available at any time that a person wants to use them, i.e., on demand.
Paratransit: an alternative mode of flexible passenger transportation that does not follow fixed routes or schedules, are common and often offer the only mechanized mobility options for the poor in many parts of the developing world.
Speed Harmonization: gradually lowering speeds before a heavily congested area in order to reduce the stop-and-go traffic that contributes to frustration and crashes.
Transport Network Companies (TNCs): Transportation Network Companies (TNCs) provide prearranged transportation services for compensation using an online-enabled application or platform (such as smart phone apps) to connect drivers using their personal vehicles with passengers.
Media Attributions
Figures
- Figure 8.1: Potential energy/emissions impacts of automated technology from “Bending the Curve: Climate Change Solutions” by Ramanathan et al. is under the following Creative Commons license: CC BY-NC-SA 4.0
Tables
- Table 8.1: Intelligent transportation system applications utilizing connected and automated vehicle technology from “Bending the Curve: Climate Change Solutions” by Ramanathan et al. is under the following Creative Commons license: CC BY-NC-SA 4.0
References
- Alonso-Mora, J., Samaranayake, S., Wallar, A., Frazzoli, E., & Rus, D. (2017). On-demand high-capacity ride-sharing via dynamic trip-vehicle assignment. Proceedings of the National Academy of Sciences, 114(3), 462-467.
- Bauer, G. S., Greenblatt, J. B., & Gerke, B. F. (2018). Cost, energy, and environmental impact of automated electric taxi fleets in Manhattan. Environmental science & technology, 52(8), 4920-4928.
- Bischoff, J., & Maciejewski, M. (2016). Simulation of city-wide replacement of private cars with autonomous taxis in Berlin. Procedia computer science, 83, 237-244.
- Castiglione, J., Cooper, D., Sana, B., Tischler, D., Chang, T., Erhardt, G., Roy, S., Chen, M., & Mucci, A. (2018, October). TNCs & congestion. Draft report, San Francisco County Transportation Authority.
- Castillo, J. C., Knoepfle, D., & Weyl, G. (2017, June). Surge pricing solves the wild goose chase. In Proceedings of the 2017 ACM Conference on Economics and Computation (pp. 241-242). ACM.
- Greenblatt, J. B., & Shaheen, S. (2015). Automated vehicles, on-demand mobility, and environmental impacts. Current sustainable/renewable energy reports, 2(3), 74-81.
- Hall, J. D., Palsson, C., & Price, J. (2018). Is Uber a substitute or complement for public transit?. Journal of Urban Economics, 108, 36-50.
- Henao, A., & Marshall, W. E. (2018). The impact of ride-hailing on vehicle miles traveled. Transportation, 1-22.
- Hu, L., Dong, J., Lin, Z., & Yang, J. (2018). Analyzing battery electric vehicle feasibility from taxi travel patterns: The case study of New York City, USA. Transportation Research Part C: Emerging Technologies, 87, 91-104.
- Komanduri, A., Wafa, Z., Proussaloglou, K., & Jacobs, S. (2018). Assessing the impact of app-based ride share systems in an urban context: Findings from Austin. Transportation Research Record, 2672(7), 34-46.
- Meyer, J., Becker, H., Bösch, P. M., & Axhausen, K. W. (2017). Autonomous vehicles: The next jump in accessibilities?. Research in Transportation Economics, 62, 80-91.
- Ramanathan, V., Aines, R., Auffhammer, M., Barth, M., Cole, J., Forman, F., et al. (2019). Bending the Curve: Climate Change Solutions. Location: Regents of the University of California. Editor: V. Ramanathan. Co-Editors: Adam Millard-Ball; Michelle Niemann; Scott Friese. Book published by the Regents of the Univ of California. Retrieved from https://escholarship.org/uc/item/6kr8p5rq. 815pp. https://creativecommons.org/licenses/by-nc-sa/4.0/
- SAE international. (2016). Taxonomy and definitions for terms related to driving automation systems for on-road motor vehicles. SAE International,(J3016).
- Schaller, B. (2018). Making Congestion Pricing Work for Traffic and Transit in New York City. Schaller Consulting.
- Wadud, Z., MacKenzie, D., & Leiby, P. (2016). Help or hindrance? The travel, energy and carbon impacts of highly automated vehicles. Transportation Research Part A: Policy and Practice, 86, 1-18.
- Walker, J., & Johnson, C. (2016). Peak car ownership: The market opportunity of electric automated mobility services. Rocky Mountain Institute.
- Wenzel, T., Rames, C., Kontou, E., & Henao, A. (2019). Travel and energy implications of ridesourcing service in Austin, Texas. Transportation Research Part D: Transport and Environment, 70, 18-34.
- Xu, Z., Yin, Y., & Ye, J. (2019). On the supply curve of ride-hailing systems. Transportation Research Part B: Methodological.
Attributions
- Bending the Curve: Climate Change Solutions by Ramanathan, V., Aines, R., Auffhammer, M., Barth, M., Cole, J., Forman, F., et al. is licensed under CC BY-NC-SA 4.0
On-demand mobility services are transport services that are available at any time that a person wants to use them, i.e., on demand.
an alternative mode of flexible passenger transportation that does not follow fixed routes or schedules, are common and often offer the only mechanized mobility options for the poor in many parts of the developing world.
Transportation Network Companies (TNCs) provide prearranged transportation services for compensation using an online-enabled application or platform (such as smart phone apps) to connect drivers using their personal vehicles with passengers.
an advanced application which aims to provide innovative services relating to different modes of transport and traffic management and enable users to be better informed and make safer, more coordinated, and 'smarter' use of transport networks.
an extension to the adaptive cruise control (ACC) concept using Vehicle-to-Everything (V2X) communication. Adaptive cruise control (ACC) is a type of advanced driver-assistance system for road vehicles that automatically adjusts the vehicle speed to maintain a safe distance from vehicles ahead.
gradually lowering speeds before a heavily congested area in order to reduce the stop-and-go traffic that contributes to frustration and crashes.