8 Transportation and New Technologies
CHAPTER OVERVIEW
Urban transportation in the United States has been profoundly influenced by evolving technologies across time. This chapter explores the transformative impact of these innovations on urban mobility, examining their potential benefits, challenges, and implications for future urban planning.
First, the historical context and evolution of transportation technologies from walking to the motor age are discussed. By learning these past transportation regimes, we set the stage for examining the current era dominated by Information and Communication Technology (ICT) and its implications for urban environments. Like the historical examples, innovations such as Shared Autonomous Vehicles (SAVs) and Smart Cities , are revolutionizing travel patterns, and enhancing efficiency, and may also come with challenges and serious implementation considerations. Modern planners also consider data privacy, equity in access, and other implications of new transportation modes.
CHAPTER TOPICS
- Transportation Technologies and Urban Form
- Current Era: ICT and the Smart City
- Shared Mobility and Technology
- Smart Cities
- Conclusion
- Quiz
- Glossary
- Acronyms
LEARNING OBJECTIVES
Learning Objectives
- Identify key historical transportation regimes based on the power sources and infrastructure that have influenced transportation and urban development patterns.
- Summarize the advantages and challenges of integrating Information and Communication Technologies (ICTs) into urban transportation systems and Smart City Mobility Solutions.
- Describe the levels of Shared Autonomous Vehicles (SAVs) and the benefits of integrating SAVs into on-demand ridesharing mobility services.
INTRODUCTION
Did you travel to work or school today? How we get around is shaped by various factors, especially the technologies dominating our transportation systems. Even if you chose a more basic mode of travel, like cycling, your journey probably involved interactions with more modern methods, such as hybrid or electric vehicles, buses, or trains. You might have used your smartphone to hail a ride or activated a shared bicycle through an app. These changes might seem small in our daily lives, but the impact of technology on transportation is significant.
Transportation technology historians generally categorize four main eras based on the power source for movement, which greatly influences the layout of urban areas; these eras do not signify abrupt shifts, but rather gradual changes that often blend old and new technologies. However, it is important to recognize that technologies do not exist in isolation, and their effects are not neutral; they frequently bring unanticipated consequences.
As discussed in Chapter Five, the implementation of transportation technologies as modes of mobility involves a complex interplay of technology, infrastructure, energy, regulations, and public policy, which collectively form what we call transportation regimes (Hoffman et al., 2017). Additionally, as outlined in Chapter Six, by providing access to previously undeveloped land, transportation enables new development. Understanding the interplay between transportation and accessibility and the larger context of transportation technology implementation is crucial for the concepts explored in this chapter, which focus on the urban implications of the current ICT era.
TRANSPORTATION TECHNOLOGIES AND URBAN FORM: A BRIEF HISTORICAL ACCOUNT
Shifts in Transportation Technology Impacting the Urban Form of US Cities
Besides walking and animal-powered mobility in the 17th and 18th centuries, rivers initially served as the main routes for colonists venturing into the interior of the United States. As a result, the expansion of settlements westward after the American Revolution primarily followed these river routes. This expansion was later facilitated by the construction of canals, with a notable example being the completion of the Erie Canal in 1833. Alongside advancements in canal lock technology, an additional four thousand miles of canals were constructed, supporting future steamboat transportation for both cargo and passengers to numerous inland and coastal cities. These technological improvements significantly reduced travel times. For instance, the journey from New York City to Louisiana decreased from four weeks in 1800 to just two weeks by 1830. Similarly, the trip from New York City to Chicago was cut in half, from six weeks to three, during the same period (see Figure 8.1). Ironically, the development of water canals spurred the construction of the first rail lines, which eventually led to the demise of canals as railroads offered greater hauling capacity and speed at a lower cost.
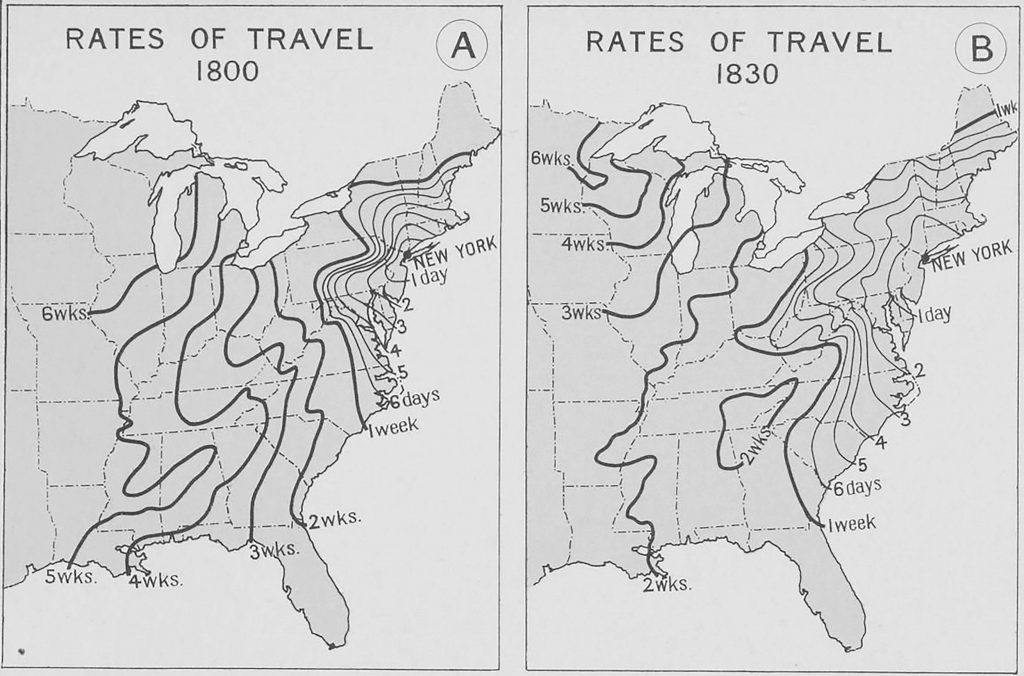
The Steam Engine Age
Steam locomotives and the expansion of over 9,000 miles of rail significantly extended transportation distances across the United States, reaching areas not easily accessible via waterways. By 1857, it became possible to travel the entire continent from New York City to California in less than four weeks. Similarly, a journey from New York City to Texas, which might have taken over six weeks in 1800, was reduced to less than two weeks by 1857. The trip from New York City to Georgia saw a remarkable decrease from three weeks in 1800 to just three days in 1857. This dramatic reduction in travel times and the increased accessibility of western territories marked a pivotal moment in the history of transportation and urbanization in the United States. Cities and industries connected by rail experienced growth, while new towns and settlements emerged around railroad depots and stations. Within cities, electric streetcars emerged in the late 1800s and provided cleaner and more efficient travel than the horse-drawn carriages and trolleys they replaced. Streetcar suburbs built along the intracity rail lines enabled workers to live on the periphery and commute into the city center for work (“Streetcar suburb,” 2024). These technologies laid the foundation for modern transportation systems and influenced subsequent developments in engineering and infrastructure.
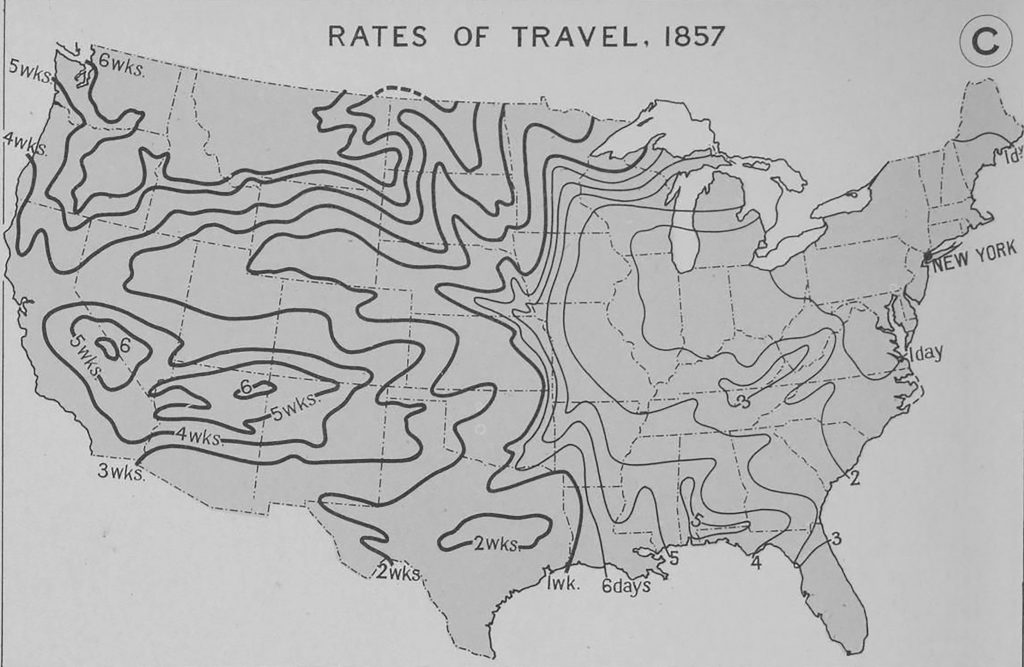
The Motor Age Era
The introduction of internal combustion engines in the early 20th century led to increased mobility through roads, highways, trucking, and personal automobiles. This transition gradually replaced passenger rail and trolley lines, both within and between cities. Since the early 20th century, particularly following the 1916 Federal Aid-Highway Program, cities and states invested significantly in road infrastructure, resulting in today’s extensive network of nearly 4,000,000 miles of public roads. This network, combined with the approximately 47,000 miles of the limited-access Interstate Highway System, solidified the dominance of trucks and automobiles as the primary modes of transportation in the United States.
As discussed in Chapter Six, the transportation network forms the foundation of urban structure, shaping cities’ built environment and development patterns. These patterns of urban development have been conceptualized in various urbanization and land use models. One such model, the sector model by Hoyt (1939), illustrates the outward expansion of urban growth along different sectors radiating from the central business district (CBD) and along intraurban rail and trolley lines in the early 20th century (see Figure 8.3).
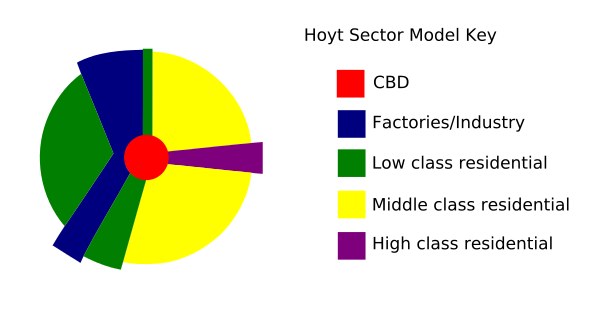
For further examination of this and other models and theories of urban form and land rent that chart the connection between transportation and urban land use, please check out Chapter Three of the Transportation Land-Use Modeling & Policy OER (Open Education Resources) text.
Speed improvements in intracity mobility—from walking and horse-drawn modes to bicycles, trolleys, and trains peaking with automobiles—were promoted by both private enterprise and public transportation policy of the Motor Age. By the 1920s, the private automobile was emerging as the dominant mode of transportation, leading to the decentralization of the central business district (CBD) in favor of locations near highway junctions accessible by car and truck (Knowles et al., 2020).
During earlier periods when mobility technologies such as bicycles or horse-drawn carriages were predominant, urban areas typically exhibited a more compact and denser development pattern. However, with the widespread adoption of automobiles, particularly after World War II, cities embraced modern city planning and segregated zoning, resulting in car-oriented sprawling suburban layouts with increasing separation between single-family homes, jobs, and shopping areas. The shift towards automobile-oriented development required significant space for roadways and parking infrastructure, ultimately shaping the vast areas of decentralized and sprawling suburban and exurban landscapes we see today (Grabar, 2023; English, 2019).
As a result, urban areas expanded their boundaries, contributing to the phenomenon of urban sprawl. Urban sprawl is characterized by uncontrolled or loosely regulated growth extending towards suburban or exurban peripheries, encompassing housing, jobs, retail, industrial, and other urban developments, as well as extensive highway and road networks.
American car- and truck-centric urban sprawl has faced widespread criticism due to its adverse effects on the environment, transportation equity, quality of life, and public costs. These criticisms were extensively documented in TCRP Report 39 by Burchell et al. (1998), which compiled these concerns.
While these developments are typically lower in density compared to the central urban area, sprawling suburban developments often contain their own specialized higher-density nuclei, as illustrated in C. D. Harris and Edward L. Ullman’s (1945) multiple nuclei model of urban structure (Figure 8.4). The model depicts the emergence of competing suburban downtown nodes of development, known today as metropolitan subcenters. In addition to concentrating a large number of jobs in commerce and industry, subcenters have spawned around airports and connecting highways as important airfreight and passenger hubs, such as Alliance in the periphery of Fort Worth, the DFW airport, and many other such urban agglomerations denominated “aerotropolis” by John Kasarda (2019).
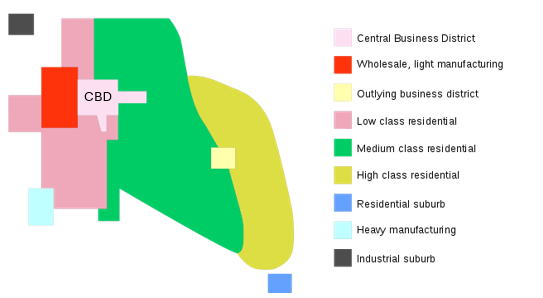
Airport-Oriented Metropolitan Subcenters
The advent of continent-spanning air travel, high-speed rail, and the potential resurgence of technologies like Maglev or even the eventual realization of hyperloop trains—all enabling high-speed movement of people and goods over extensive distances—sometimes collectively labeled “hypermobility,” point to the fact that the commercial deployment of these transportation technologies has been reshaping vast territories by extending connectivity and accessibility over larger geographical areas.
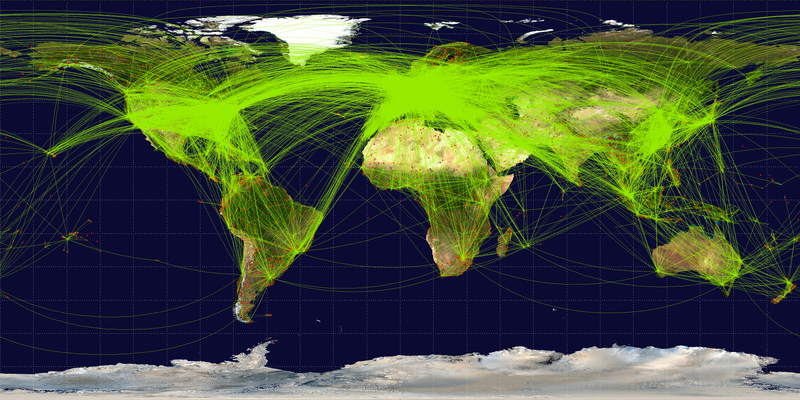
The transportation dependencies of global trade, facilitated by air freight and container cargo shipping for just-in-time production, have made airports and their air routes, according to Kasarda (2019), important drivers of urban settlements and development in the 21st century. This parallels the roles played by highways and trucks in the 20th century, railroads and depots in the 19th century, and seaports and channels in the 18th century.
Airport-centric metropolitan subcenters, or aerotropolises, as previously mentioned, have emerged as responses to the economies of speedstemming from the demands of time-sensitive commerce and production facilitated by internet-based communications and commerce. These hubs encompass logistics and e-commerce fulfillment centers, information technology parks, time-critical carriers, and distribution centers (such as FedEx, UPS, and DHL), handling documents, medical supplies, and high-value perishable goods. These airport-oriented developments also include amenities like hotels, offices, retail spaces, entertainment venues, and residential areas to accommodate workers and visitors.
Several airports worldwide serve as strategic global hubs, such as Memphis International Airport (MEM), which serves as a hub for FedEx Express; Louisville Muhammad Ali International Airport (SDF), home to UPS Worldport; and Los Angeles International Airport (LAX), a gateway for U.S.-Asia trade. These airports play crucial roles in facilitating international trade and commerce, underscoring their significance in shaping global supply networks.
Conventional airports expanding as transshipment sites for freight and passengers in response to the integration of high-tech activities and air transportation are considered airport cities or aviation agglomerations, while aerotropolis is the name given to airport cities planned from the ground up. The Atlanta region has developed an aerotropolis blueprint for Hartsfield–Jackson Atlanta International Airport (ATL); see Figure 8.7. Located approximately 10 miles south of Downtown Atlanta and the busiest airport in the world, ATL also has plans to connect with nearby mixed-use commercial and residential developments, convention and office spaces, distribution and logistics centers, etc., that recognize the critical infrastructure and human capital involved in aerotropolis economic activities.
Box 8.2 The Aerotropolis Model by John Kasarda, Aerotropolis http://aerotropolis.com/index.php/about/ , n.p.
A spatially compressed model of the Aerotropolis showing its current and likely future evolution is illustrated below. No Aerotropolis will look exactly like this, but most will eventually take on similar features, led by newer “greenfield” airports on metropolitan peripheries less constrained by decades of prior surrounding development. The Aerotropolis is, therefore, much more of a dynamic, forward-looking model than a static, cross-sectional model that often reflects historic airport area development before air commerce played such an important economic role. It is likewise more than corridors and clusters of aviation-dependent commercial, industrial, and logistics facilities. The aerotropolis also encompasses living urban places that must be designed as appealing environmental and social realms if the model is to achieve its full potential. Since economic sustainability is a prerequisite for development success, the aerotropolis must further be planned and administered to appeal to the business logic of investors, commercial real estate developers, and their facility end-users.
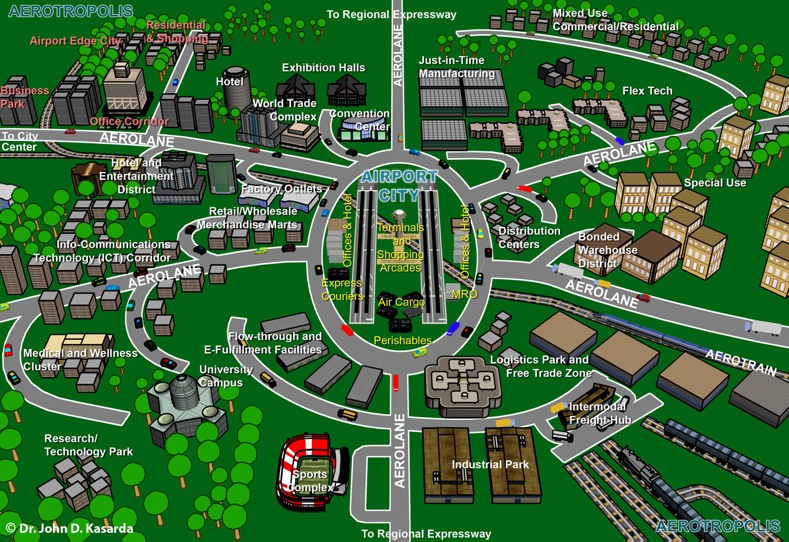
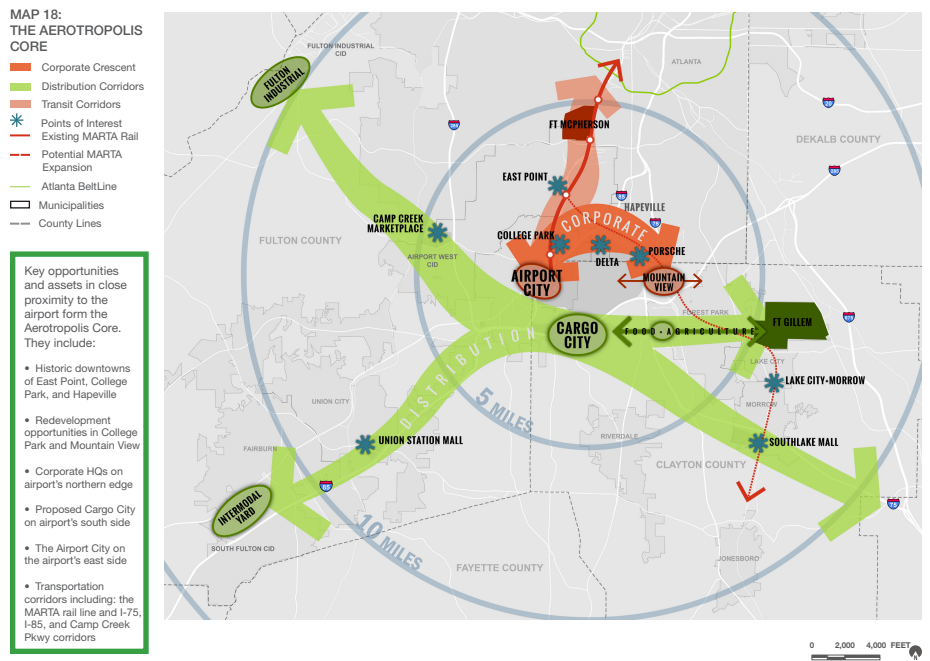
CURRENT ERA: INFORMATION AND COMMUNICATION TECHNOLOGY (ICT) AND THE SMART CITY ERA
Introduction to ICT and Travel
The widespread adoption of information and communication technologies (ICTs), since the last decades of the 20th century, has profoundly transformed society, the structure of work and organizations, people’s lifestyles, mobility capabilities, and travel. The relationship between ICT and transportation is continuously evolving with technological advancements. While it is easier to recognize these connections in hindsight, predicting them remains challenging due to the rapid pace of technological change and the two-way nature of technology and transportation interactions.
Ongoing research in transportation geography, economics, and planning related to ICT and transportation continues to expand alongside technological innovations. Some of the most important ICT impacts on travel are indirect through ICTs’ restructuring effects on industry and organizations reflected in the transformation of employment and shifts in industry and employment locations with corresponding changes in travel patterns and travel demand resulting from changes in urban development patterns and the nature of work. Other ICT impacts on travel are direct, namely those that result from replacing actual travel with online or telemediated activities or synergistically affecting each other such as online activities inducing more travel. ICTs have enabled new ways of conducting activities, accessing information, and making travel choices. They have also revolutionized transportation analysis by leveraging data generated through mobile ICT systems (Pawlak et al., 2020).
Along with ICT innovations in mobility, such as autonomous vehicles and ride-hailing services, the 21st century has seen the strong incursion of the IT sector into the realm of transportation and transportation planning, previously the domain of public transportation agencies, municipalities, and consultants. Transportation network companies (TNCs) and IT firms, as smart city solutions vendors and partners, have become important new transportation actors and stakeholders.
This section outlines what is known about ICT effects on travel, with the caveat that such effects are in constant flux and deeply intertwined in technological change. The next section delves into smart city mobility solutions and possible implications.
Indirect and Direct Effects of ICT on Travel
Spatial effects
Despite the disruptive potential of ICTs, certain long-term impacts have been extensively documented. Advanced telecommunications, such as broad-band Internet, personal computers, GPS, and mobile smartphones, have facilitated the reorganization of activities in both time and space. For businesses, since the 1980s, this has resulted in the digitization and spatial separation of intellectual and labor-intensive functions. Labor-intensive production has been decentralized to lower-cost or strategically important locations, including suburban or rural areas, or offshored to other countries. Telecommuting, while available to certain occupations and IT workers before, became ubiquitous during the COVID-19 pandemic.
High-end functions like central headquarters and management offices, which rely on face-to-face interactions, have remained physically separated from more routine functions, such as support activities, which can be suburbanized or peripheralized due to their lesser reliance on direct face-to-face communication. These centripetal and centrifugal trends have reshaped cities and regions, centralizing advanced services (e.g., finance, insurance, design) in old central business districts (CBDs) and creating new agglomerations of high-tech industries, R&D centers, universities, and headquarters in suburban subcenters. This has led to more spread-out, intensely ICT-networked urban regions (Sassen, 1995; Audirac, 2005; Circella and Mokhtarian, 2017).
E-commerce and Teleactivities
The advent of e-commerce and online shopping has promoted the growth of fulfillment centers, leading on one hand to store closures and a reduction in the number of available shopping opportunities (Lee & Circella, 2019) and, on the other, to increases in delivery-related traffic. Along with these, telemedicine, telebanking, tele-leisure, online learning, and most current online activities that were once exclusively land-use-based have been spatially disrupted by digitization with concomitant implications for travel. At the household level, the ICT restructuring effects on work and teleactivities, including social media, have resulted in changes in households’ travel behavior with implications for traffic, energy consumption, and emissions (Mouratidis et al., 2021).
The resulting effects on accessibility and physical travel stem from the highly complex interactions between ICT-networked online activities such as telebanking and distance learning and onsite activities connected via physical infrastructure like bank teller services, and on-campus learning. These accessibility effects can be indirect through shifts in land use patterns or more direct through the substitution and complementarity of travel. A Transportation Research Board Centennial report appraising the state of knowledge of ICT and travel over the previous 20 years, stated: “It has become increasingly apparent that the interactions between ICT and transportation systems typically comprise a blend of some factors that result in the reduction of travel, along with others that facilitate and ultimately increase it or modify its characteristics, such as mode, route or departure time” (Pawlak et al., 2020, n.p.).
Research on ICT effects on travel behavior began in the 1980s, amassing over time an extensive body of empirical work centered on four hypothesized effects based on Salomon’s (1986) and Mokhtarian ‘s (1990) taxonomy of interactions between ICT, activities, and travel:
- Substitution: ICT activities replace physical travel with virtual travel (e.g., replacing physical trips to a store with online shopping, replacing trips to work with telecommuting, or trips to college with online education.
- Complementarity: ICT use generates additional travel (e.g., making additional trips to stores to examine and try out items before making a purchase online.
- Modification: ICT use induces changes in travel patterns that did not exist before the ICT use, which can involve changes in destinations, travel modes, travel times, or activity durations, such as going to different stores based on online price research or searching and selecting a different food place destination while en-route to.
- Neutrality: ICT use has no effect on travel.
(Mokhtarian et al., 2004; Weltevreden & Rietbergen, 2007; Andreev et al., 2010; Lee & Circella, 2019; Ozbilen et al., 2021).
Although ICT effects on travel are widely recognized, there is still debate among researchers on whether substitution or complementary are the most significant effects with some findings supporting substitution primarily in telecommuting, while both substitution and complementarity have been found for online shopping. The substitution effect is particularly important for sustainability and transportation planning concerns in terms of potential reductions in overall travel and vehicle miles traveled (VMTs), traffic congestion, and emissions resulting from fewer vehicles on the roads.
A 2010 review of 100 conceptual and empirical studies (from 1975 to 2007) on tele-activities effects (substitution, complementarity, modification, and neutrality) on travel revealed that the substitution effect was most prevalent for telecommuting, whereas complementarity was a major impact for teleshopping and teleleisure activities. Notably, more than 50% of the reviewed projects focused on telecommuting, with significantly fewer studies examining teleshopping and teleservices, and even fewer addressing teleleisure. The duration of the ICT effects studied was mostly short-term, with blurred or inconclusive ICT effects for the long term. Some studies suggest that the long-term substitution effect of telecommuting would be offset by induced travel demand for other types of travel or by longer commutes if telecommuting induced residential relocation farther away from work (Andreev et al., 2010).
A decade later, a review of 42 empirical studies (from 1996 to 2019) of online shopping effects on travel behavior related to trip frequency, travel distance, trip chaining, mode choice, and time was conducted primarily in the US, China, and the Netherlands found more evidence for the substitute effect than any of the other three effects (Le et al., 2021, p. 273).
A pre-COVID-19 pandemic empirical study using the 2017 Puget-Sound Regional Travel Study (PSRTS) and the Environmental Protection Agency (EPA)’s Smart Location Database (SLD) analyzed time spent on different travel modes (auto, public transit and active travel) and ICT use for telework (telecommuting) and online shopping controlling for the influence of socio-demographics, individual attitudes, and land-use characteristics (Ozbilen et al., 2021). The study showed that the effects of ICT use vary across the three travel modes. While there was a negative association (substitution effect) between teleworking and auto and transit, there was a positive association (complementary effect) between online shopping and active travel. In other words, the more time spent on telework the less time spent on driving or riding transit. On the other hand, the more time spent on online shopping, the more time respondents also spent on walking and bicycling—with stronger complementarity effects the greater the level of land-use mixture in the respondents’ neighborhoods. Furthermore, respondents’ demographics modulated these results, indicating that among those shopping online, respondents with higher education (graduate degrees) spend more time driving than others. While among those with increased teleworking hours, Hispanics spent more time in transit than others (Ozbilen et al., 2021).
Since the COVID-19 pandemic, there have been significant shifts in travel behavior and the use of ICT to facilitate telework or “working from home” (WFH), the name used in official statistics. Most notably, there has been a substantial increase in working from home (WFH) and the use of online shopping and delivery services due to pandemic-related restrictions (Bloom, 2020; Circella et al., 2023; Matson et al., 2022; Patwary & Khattak, 2022). These behaviors have persisted even after social distancing measures were lifted. WFH is estimated to boost productivity due to time savings from eliminated commutes and teleconferencing and other online working improvements facilitated by ICT (Barrero et al., 2021; Wladawsky-Berger, 2023).
Students are encouraged to explore publicly available data from the U.S. Bureau of Transportation Statistics (BTS) at BTS COVID-19 Changes in Travel Behavior to observe these changes in commuting patterns across various geographical scales and to view changes in work-from-home, transit, and ride-sharing trips, trips to stores, and online purchasing.
ICT and Other Transportation Effects
- Freight Supply Chain Logistics: ICT has played a pivotal role in enhancing the efficiency of goods’ production and distribution, bringing about significant changes in the supply chain. The reduction of transportation costs facilitated by ICT can lead to an increased demand for goods. Furthermore, ICT has transformative effects on the distribution of goods, manifesting in several ways:
- Economies of Scale Benefits: ICT increases the advantages of economies of scale, allowing for more efficient production and distribution processes, such as the containerization of freight through automation of land and water ports (Jerow, 2023).
- Just-in-Time (JIT) Supply Chain: Enabled by ICT, the just-in-time supply chain model is implemented, leading to more frequent and precisely timed deliveries responding to the economies of speed (Circella & Mokhtarian, 2017).
ICT and new mobility innovations
ICT has played a direct role in the rise of carsharing and ridesharing services (Kong et al., 2020). Transportation network companies (TNCs) leverage ICT to manage service usage and maintenance, while travelers use online platforms to schedule trips and book rides. In the short term, ICT, in the form of smartphone apps, enables users to swiftly navigate “on-the-fly” and alter their travel based on available app data like Google or Apple Maps. It can also transform an individual’s space-time constraints, such that people can perform work, shopping, or other digitized tasks nearly anywhere they have their mobile phone. Activity participation and travel behavior are impacted as activities can now be divided in time or fragmented across places; for example, areas once reserved for leisure, such as a hotel on vacation, now serve the additional function of space for work (Arranz-Lopez & Soria-Lara, 2022; Ben-Elia et al., 2014; Circella & Mokhtarian, 2017, p.98).
SHARED MOBILITY AND TECHNOLOGY
What Is Shared Mobility?
Shared mobility, as defined by the United States Department of Transportation (U.S. DOT), involves the shared use of a car, bicycle, or any mode of transportation that allows users to access rides on demand without the necessity of ownership (Shaheen et al., 2016b) (see Figure 8.11 for examples of incumbent and innovative services). Frequently, shared mobility is situated in the micro-mobility space and can serve as a potential solution to the first-mile/last-mile transportation challenge. This concept is closely associated with mobility as a service (MaaS), where cities or regions integrate various mobility options across modes through a single application for smartphones or mobile devices. MaaS aims to provide a seamless and connected experience for users by offering a comprehensive range of transportation services within a unified platform.
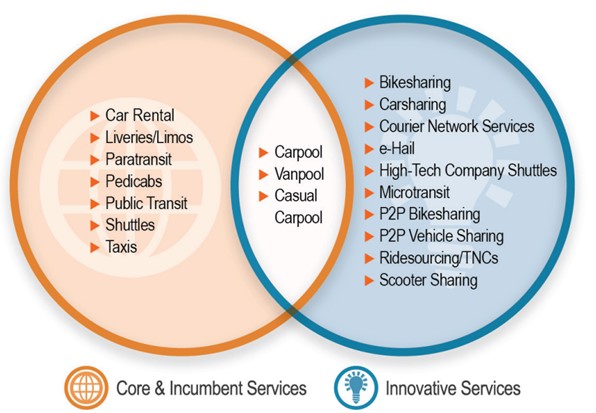
As cities move to more innovative services and adopt MaaS, the activities of passengers utilizing these services generate a vast amount of data. These data become both a potential asset and a liability for cities as they work to leverage data to transform how people, goods, and services are transported while simultaneously safeguarding these data from security and privacy concerns (Paiva et al. Ahad, Zafar, Tripathi, Khalique, & Hussain, 2020). Cities have coped with these dual demands through public-private partnerships (PPPs) in which private sector companies provide IT solutions as vendors to cities and transit authorities to meet the needs of city residents and passengers (SUMC, 2019). However, data security breaches, user data privacy, and commercialization remain business model issues of the technology. Lack of competition in a sector with a few large vendors of transportation technologies may also reduce the ability of cities to sustain convenient and affordable transportation and mobility for the wide array of needs of residents and visitors (Karlsson, I. C. M. et al., 2020; Paiva et al., 2020).
Shared mobility is not exclusively provisioned by cities, private companies (Uber/Lyft), or PPPs. Ridesharing may also happen through informal channels that directly connect people to other people to share rides or vehicles, such as Craigslist. In utilizing social media or message boards these carpooling matches may be enabled through ICT outside of smartphone applications expressly designed for mobility (Paul, 2023). Greater utilization of ridesharing and vehicle-sharing could benefit regions to meet the needs of residents, especially in car-dependent regions, though it is important to consider the potential concerns around personal safety and information sharing/data security (Paul, 2023). Safety issues are also evoked in Chapter Ten, Transportation and the Global South, where we discuss informal transportation and the benefits and challenges of transportation outside the typical regulatory channels. Benefits like operating outside of fixed routes, less expensive transportation options, and the potential for drivers to earn income apply in the case of informal ridesharing in the United States as well as for the Global South (Paul, 2023).
Who Uses and Does Not Use Shared Mobility?
In the recent past, research has delved into the socio-demographics of shared mobility users, revealing certain trends. For instance, carpooling services were found to be more commonly used by younger adults without children (Shaheen et al., 2016). Shared mobility users were characterized by predominantly being white, male, aged between 20 and 35, and possessing a higher level of education compared to the overall population. Also, factors such as land use and built environment characteristics help distinguish shared mobility usage. These characteristics encompassed elements like population density, proximity to public transit services, and levels of automobile ownership (Dill et al., 2015; Shaheen et al., 2016). However, these trends are not static and vary by the type of shared mobility studied, which includes motorized mobility like car sharing and non-motorized or electrified like bicycles and scooters.
Researchers often focus on studying mobility decisions and the determinants of mode choice. Several transportation barriers can limit the use of shared mobility services. One significant barrier is the demographics of the area, with research indicating that car-sharing vehicles are often concentrated in higher-income neighborhoods (Schmitt, 2012). This means that transit-dependent populations in lower-income neighborhoods may face challenges in accessing formal shared mobility options.
Access to credit and/or debit cards is another potential barrier. Many shared mobility services require these cards for payment, making it difficult for approximately 15% of U.S. consumers without access to credit/debit cards to use such services (Pew Research Center, 2016). Despite low-income populations and minorities theoretically being beneficiaries of shared mobility, research suggests that these barriers have been assumed away as a public sector responsibility (Schmitt, 2012).
The digital divide, which refers to those without or impaired access to the internet or telecom services, magnifies the significance of this barrier when dealing with shared mobility. Identifying the populations affected by the digital divide is crucial in planning for populations with specific needs for shared mobility access (Shaheen et al., 2017). Addressing these barriers is essential for ensuring equitable access to shared mobility services for diverse populations.
Transportation Barriers and Ridesharing Opportunities
Shaheen and colleagues (2017) categorize transportation barriers and ridesharing opportunities based on various issues:
- Economic Barriers: Travel costs can be a significant obstacle, preventing users from allocating funds to other essential needs. Housing and transportation are the most substantial expenses for American households, consuming 30% and 19% of the average family’s income, respectively.
- Ridesharing Opportunity: Ridesharing mobility can offer an affordable travel option, potentially reducing household transportation costs.
- Physiological Barriers: Individuals with physical or cognitive disabilities may face challenges in utilizing available transportation options, creating a barrier.
- Ridesharing Opportunity: Ridesharing options can address physiological barriers by providing services more efficiently designed to meet the needs of people with disabilities.
- Social Barriers: Social barriers encompass challenges faced by disadvantaged communities in using transportation services, including cultural, safety, and language barriers.
- Ridesharing Opportunity: On-demand ridesharing options can address social barriers by incorporating features such as limiting socio-demographic profiling in apps, conducting focused outreach to low-income and minority groups, and providing app information in the user’s native language (Shaheen et al., 2017). These measures aim to make users more comfortable with transportation services.
Shared Autonomous Vehicles
Automated Vehicles
Automated vehicles (A.V.s) are categorized into different levels based on the extent of automation, as defined by the National Highway Traffic Safety Administration (NHTSA, 2021). The levels are as follows:
Level 0: All driving is done by a human driver.
Level 1: An advanced driver assistance system (ADAS) on the car can occasionally assist the human driver with either steering or braking/accelerating, but not both.
Level 2: Under certain conditions, an ADAS on the car may manage both steering and braking/acceleration simultaneously. The human driver must complete the remainder of the driving duty while maintaining complete concentration on the driving environment.
Level 3: In some situations, a vehicle’s autonomous driving system (ADS) can handle every facet of the driving duty independently. The human driver must be prepared to regain control at any time when the ADS requires it. In all other cases, a human driver carries out the driving duty.
Level 4: Under specific conditions, an ADS on the vehicle may independently handle all driving duties, monitor the road environment, and practically conduct all the driving. In certain conditions, the person is not required to pay attention.
Level 5: The vehicle’s ADS can perform all driving tasks under all conditions. Human occupants are considered guests and are not required to be present (NHTSA, 2021).
These levels are in order from lesser to greater autonomy and with less human intervention at higher levels.
Benefits of Autonomous Vehicles
The integration of autonomous vehicles (AVs) into on-demand ridesharing mobility services, facilitated by fee and app integration, has the potential to make the deployment of autonomous vehicles profitable. This integration not only enhances mobility but also contributes to equity, especially when autonomous vehicles are widely deployed and integrated with existing public transit networks (Fagnant & Kockelman, 2015).
Shared autonomous vehicles (SAVs) or self-driving shuttles, accessed through mobile applications, offer a promising mode of transportation, particularly for the elderly and low-income individuals (Narayanan et al., 2020).
According to Milakis et al. (2017), autonomous vehicles have the potential to replace conventional cars by 67 to 90%, providing mobility levels equivalent to those of private automobiles. Moreover, with the implementation of strict policies, Shared Autonomous Vehicle (SAV) systems can significantly reduce the need for individual vehicle ownership. However, it is important to note that the operational costs of SAV services constitute two-thirds of the overall cost, and when considering the total expenses, SAVs may not be inexpensive enough to entirely replace all existing modes of transportation (Milakis et al., 2017).
Components of SAV Modeling
Shared Autonomous Vehicle (SAV) services come in three main types: car-sharing, ridesharing, and hybrid systems. These services can operate independently through autonomous systems, without the need for connection to any other mode. Additionally, there are integrated services known as Public Transit–Shared Autonomous Vehicle (P.T.–SAV) systems, which combine public transit with SAV systems to provide a comprehensive and integrated transportation solution (Narayanan et al., 2020).
For students interested in learning more about transportation modeling, the recommendation is to visit the OERTransport textbook “Transportation Land-Use Modeling and Policy” for in-depth insights into this field.
AV and SAV Adoption
The adoption of Shared Autonomous Vehicles (SAVs) is influenced by two key factors: the availability of Information and Communication Technology (ICT) and residential location. Additionally, socio-demographic and attitudinal factors play significant roles, according to Etminani-Ghasrodashti et al. (2002).
Socio-demographic factors
Socio-demographic factors that have been found to contribute to AV and SAV adoption include the finding that individuals with higher education and income levels are more willing to adopt new technologies with smaller potential profits and lower risks. Additionally, younger adults (18-34 years old) are more likely to use self-driving technology. However, women are less likely than men to commute alone in Autonomous Vehicles (AVs). Another interesting finding is that electric vehicle owners are more inclined to use A.V.s compared to diesel vehicle owners, and public transportation users with flexible work schedules are more likely to embrace SAVs. Finally, people with higher incomes, urban residents, and those who have experienced accidents are more inclined to use A.V.s.
Attitudinal factors
Attitudes, subjective norms, perceived behavioral control, and perceived behavioral conditions influence SAV usage intentions. Individuals who are fearful and apprehensive when using public transportation are less likely to use automated transit (Etminani-Ghasrodashti et al., 2022).
An interesting example of SAV usage can be found in Arlington, Texas, where a mobility on-demand program is in place. Further details on this program can be explored through the Shared-Use Mobility Center case study: AVs + Rideshare Rapid in Arlington, TX.
SMART CITIES
In the twenty-first century, the concept of the smart city emerged as a technology-driven approach where information and communication technologies (ICTs) embedded in digital solutions aim to tackle various urban issues. These issues range from traffic congestion, safety, and crime to CO2 and greenhouse gas emissions, energy consumption, waste recycling, and access to healthcare and education. Additionally, smart city solutions aim to improve public engagement and city government functions. In essence, smart city initiatives seek to address the environmental sustainability and social equity challenges spawned in previous eras. For instance, smart city transportation solutions include autonomous vehicles (AVs), smart buses, and IT-driven ride-hailing services, all promoted as solutions for traffic congestion and transit deserts (see Chapter Seven). These are often combined with parking and street infrastructure sensors to mitigate the negative environmental impacts, quality of life issues, and public costs associated with car-dependent urban sprawl. An example of this approach is the Sidewalk Labs Quayside Smart City project in Toronto, briefly highlighted in the following video.
Media 8.1 Sidewalk Labs Toronto – A Brief History 3:41 by Raymond Wong –a Toronto citizen. https://www.youtube.com/watch?v=Nmjtf9v-YFQ
However, the failed Quayside Smart City project points to the dangers of technological determinism, lack of transparency and data accountability, and top-down planning. People’s privacy and surveillance concerns fired back, terminating the project. Rather than the unplanned, uncontrolled chance encounters that cities and public spaces enable, smart city big data analytics, quantification, and corporate control, ran counter to the cultural, democratic, and community values of Toronto’s citizens. These and other criticisms leveled at complex digitally networked systems including data ownership and cybersecurity threats; environmental sustainability (e.g., intense energy use by data centers); and social equity and inclusion (e.g., digital divides, digital profiling, and algorithmic racial and ethnic bias in AI applications) highlight the importance of ethical and social scrutiny of smart city solutions, typically sold on efficiency terms vis-à-vis traditional cities and their capacity to address the legacy shortcomings of our current cities (Benjamin, 2019; Ziosi et al., 2022).
Thus, smart city proposals have reignited concerns cautioning against perpetuating the technical determinism of the Motor Age—characterized by planning, engineering, and public policy decisions that, in the name of science, prioritized fast automobility and car-oriented development over all other modes of surface travel and urban development patterns.
In the chapter “Livable City: The Limits and Dangers of New Technology” from the book “The Smart Enough City: Putting Technology in its Place to Reclaim Our Urban Future,” Ben Green offers a thought-provoking critique of transportation planning and policy. He draws on Peter Norton’s work, “Fighting Traffic: The Dawn of the Motor Age in the American City,” to examine how solutions driven by a technologist vision like Futurama in the early 20th century have shaped today’s transportation options making streets exclusively a domain of motorists to the detriment of other modes of travel and city livability. Recognizing the shortcomings of past “techgoggle” visions can assist transportation planners in dealing with new technologies such as AVs and avoiding similar Smart City Era pitfalls (Green, 2020).
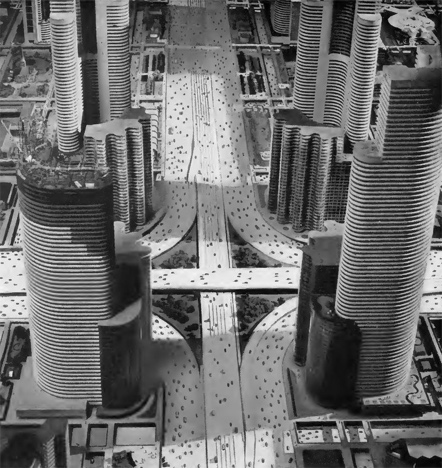
The Smart City a Globalizing Framework
By the first decade of the 2000s, cities worldwide began embarking on “smart city” programs and projects either as local government initiatives or in partnership with IT firms marketing their smart city vision, such as IBM’s “Smart City Challenge,” Google’s “Sidewalk Lab’s Toronto-Smart City,” Microsoft’s “CityNext,” Cisco’s “Intelligent Urbanization,” in the US, and Alibaba’s “Brain City” in China.
Media 8.2 IBM (2022, June 18). Smart City. [Video]. Vimeo. https://vimeo.com/721670104
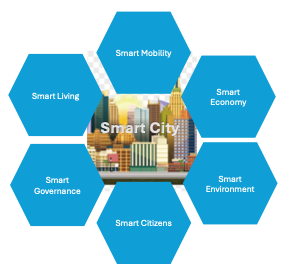
Note: Smart mobility: intelligent transportation and infrastructure, intelligent parking and traffic management, mobility-as-a-service; Smart Economy: bitcoin and smart grids, circular economy; Smart Environment: water and waste management intelligent utility systems and waste collection and recycling; Smart Citizens: connected citizens, visitors, e-health, e-learning; Smart Governance: open platforms for public participation, open data, e-government; Smart Living: smart public spaces and buildings, public security and urban resiliency (Moura and De Abreu, 2019 and “Smart City,” 2024).
Media 8.3 IBM (2011, August 12). Smarter Cities: Portland, Oregon. [Video.] YouTube. https://www.youtube.com/watch?v=uBYsSFbBeR4&t=3s
Smart City and Smart Mobility Solutions
Cities and regions often pursue sustainability goals through “smart mobility” initiatives, such as reducing CO2 emissions by alleviating traffic congestion, promoting the transition to electric vehicles, and supporting electric infrastructure. Smart mobility solutions are a crucial element of the smart city models proposed by IT companies worldwide, offering an efficient alternative to building more roads to address congestion and its environmental, economic, and societal impacts (see Table 8.1).
The European Commission’s Mobility Strategy aims for a green and digital transformation by reducing the EU’s transportation system emissions by 90% by 2050 (European Commission 2024). Similarly, the U.S. National Blueprint for Transportation Decarbonization, discussed in Chapters One and Ten, targets net-zero transportation GHG emissions by 2050, primarily through the widespread adoption of electric vehicles. The U.S. Blueprint recognizes the ongoing smart mobility transformations, including ridesharing, connectivity, automation, and remote work. However, it also emphasizes the need for better integration of policies and smart mobility solutions to prevent potential increases in travel that could arise if new technologies are not properly managed (US DOE 2023, p. 33).
Tables 8.1 and 8.2 cross lists some well-known smart city models by four U.S. Big Tech companies and their smart mobility features, summarized by the authors from responses to the prompt “What are the smart city mobility solutions of Company ________?” submitted to OpenAI’s ChatGPT and Google’s Gemini. Five main categories of features include:
- Connectivity and integration through IoT (Internet of Things), AI, and extensive use of sensors to collect data across private platforms and public (city) transportation management and operation functions including:
- Intelligent traffic management,
- Public transit optimization,
- Smart parking,
- Predictive maintenance of transportation infrastructure
- Safety and security, and
- Emergency management.
- Data-driven land use transportation planning and decisionmaking leveraging big data and advanced analytics such as mobility data from sensors, smartphones, and transportation networks, for travel demand modeling, “digital twins” modeling, “what if scenarios,” road flow and transit optimization including:
- Community engagement and stakeholder collaborations through digital platforms and open data initiatives.
- Electric and autonomous vehicles, including managing EV infrastructure and charging stations.
- Multimodal integration through platforms for various transportation modes, e.g., public transit, ridesharing, bike-sharing, micro-mobility options, and pedestrian safety analytics to offer MaaS (Mobility as a Service) seamless, multi-modal journeys for residents and visitors.
- Sustainability and Resource Management include monitoring real-time vehicle emissions and air quality for pollution reduction policies.
Microsoft | CISCO | IBM | Sidewalk Labs | IT Company |
---|---|---|---|---|
CityNext | Intelligent Urbanization | Smart City | Quayside Toronto | Smart City Mobility Solutions |
X | X | Connected Transportation: Leveraging IoT (Internet of Things) technology to create connected transportation systems that improve traffic flow, reduce congestion, and enhance safety. | ||
X | X | X | Intelligent Traffic Management: Using data analytics and AI (Artificial Intelligence) to optimize traffic signals, manage traffic flow in real time, and predict and prevent traffic incidents. | |
X | X | X | Public Transit Optimization: Developing solutions to improve public transit efficiency, reliability, and accessibility through real-time monitoring, scheduling optimization, and rider information systems. | |
X | X | X | X | Smart Parking Solutions: Deploying smart parking technologies, including sensors and mobile apps, to help drivers find available parking spaces more easily, reduce congestion, and improve urban mobility. |
X | X | Electric and Autonomous Vehicles: Supporting the adoption of electric and autonomous vehicles through infrastructure planning, data-driven policy development, and collaboration with automotive industry partners. | ||
X | Predictive Maintenance for Transportation Infrastructure: IBM’s predictive maintenance solutions use IoT sensors and predictive analytics to monitor the condition of transportation infrastructure, such as roads, bridges, and tunnels, and identify maintenance needs before they lead to disruptions or safety hazards. | |||
X | X | Mobility as a Service (MaaS): Cisco supports cities in implementing Mobility as a Service (MaaS) platforms, which integrate various transportation modes, such as public transit, ridesharing, and bike-sharing, into a single, seamless mobility solution for residents and visitors. | ||
X | X | Integrated Mobility Platforms: Providing platforms that integrate various transportation modes, such as public transit, ridesharing, bike-sharing, and micro-mobility options, to offer seamless, multi-modal journeys for residents and visitors. | ||
X | Dynamic Street Design: Sidewalk Labs planned to design streets that adapt to changing needs throughout the day, such as allocating space for pedestrians, cyclists, and public transit during peak hours, and repurposing lanes for deliveries or parking during off-peak times. | |||
X | Advanced Transportation Systems: The project aimed to implement advanced transportation systems, including autonomous shuttles, electric vehicles, and bike-sharing programs, to provide residents with diverse and sustainable transportation options. | |||
Note: Compiled by authors from answers from Open AI’s ChatGPT to the prompt “What are the smart city mobility solutions of Company name? |
Despite the above benefits associated with smart cities and smart mobility, they also imply drawbacks and challenges related to:
- Privacy and potential misuse of personal data stemming from increased surveillance and big data collection through IoT, sensors, and devices.
- Lack of transparency about how the collected data is stored and used.
- Digital divide concerns related to inequitable access to smart city technologies exacerbating existing social and economic inequalities.
- Vulnerability: connected systems are vulnerable to disruptive cyberattacks.
- High infrastructure and implementation costs and potential job displacement due to automation in the private and public transport sectors.
- In the absence of widely used shared mobility networks, more solo autonomous driving has the potential to exacerbate congestion and promote more urban sprawl.
- Potential to disrupt existing transport systems like public transit.
- Corporate-driven vs. public-driven smart-city decision-making models.
The features of mobility solutions—described above—being implemented in cities throughout the world mark the widespread diffusion of our current Smart City Era. The optimistic acceptance of this era’s fast-paced technological change hinges on its promise to address the Motor Age’s legacies of congestion, GHG emissions, and unequal accessibility. Central to this promise are IoT and AI-powered, big data-driven solutions for monitoring, modeling, and planning the transformation of transportation systems for a more environmentally sustainable, socially equitable, and livable future. However, the pushback against Toronto’s Quayside Smart City underscores this era’s challenges (highlighted above), which can be summed up as citizens’ mistrust of the power and influence of tech-driven public-private partnerships (Wachter, 2019).
Feature |
Microsoft CityNext |
Cisco Intelligent Urbanization |
IBM Smart City |
Google Smart City |
---|---|---|---|---|
Key Technologies |
Azure cloud, AI, IoT, digital twin |
Cisco Meraki, DNA Center, IoT platform, analytics |
Watson AI, IoT, blockchain, cloud |
Cloud, AI, machine learning, data analytics |
Connected Transportation |
IoT integration, vehicle connectivity, data analytics |
Connected vehicles, V2X communication, network infrastructure |
IoT, vehicle connectivity, data exchange platforms |
Connected vehicles, data platforms, cloud infrastructure |
Intelligent Traffic Management |
Traffic flow optimization, real-time traffic data, predictive analytics. London, UK |
Traffic data collection, analysis, congestion reduction, and signal optimization. Rio de Janeiro, Brazil |
Traffic analytics, incident management, traffic simulation.
Singapore |
Traffic forecasting, congestion pricing, route optimization. Toronto, Canada |
Public Transit Optimization |
Route optimization, passenger information, payment systems, fleet management. New York City, US |
Public transit integration, real-time information, payment systems. Barcelona, Spain |
Transit management, passenger experience, fare collection, optimization. Melbourne, Australia |
Transit data analysis, route optimization, passenger experience, payment. Los Angeles, CA |
Smart Parking |
Parking guidance systems, occupancy sensors, dynamic pricing. Seattle, WA |
Real-time parking availability, dynamic pricing, parking management. San Francisco, CA |
Parking management, occupancy sensors, payment systems, data analytics. Chicago, IL |
Parking availability data, payment integration, demand-based pricing. San Francisco, CA |
EVs and AVs |
EV charging infrastructure, autonomous vehicle testing, data platforms. Copenhagen, Denmark |
Connected vehicles, V2X communication, infrastructure support. Amsterdam, Netherlands |
EV charging infrastructure, autonomous vehicle platforms, data analytics. Oslo, Norway |
Mapping, autonomous vehicle testing, charging infrastructure. Los Angeles, CA |
MaaS (Mobility as a Service) |
Integration of transportation modes, payment systems, user experience. London, UK |
Mobility data platform, integration of services, user experience. Helsinki, Finland |
Mobility platform, multimodal journey planning, payment. Barcelona, Spain |
Mobility data, multimodal journey planning, user experience. New York City, US |
Predictive Maintenance for Transportation |
Predictive analytics, IoT sensors, asset management. Berlin, Germany |
Predictive maintenance models, IoT data, asset optimization. London, UK |
Predictive analytics, IoT, asset management, optimization. New York City, US |
Predictive analytics, machine learning, asset optimization. Toronto, Canada |
Integrated Mobility Platforms |
Unified platform for transportation data, services, and users. Helsinki, Finland |
Mobility data platform, integration of systems, open APIs. Barcelona, Spain |
Mobility platform, data integration, application development. Lyon, France |
Mobility data platform, open data, application development. Tokyo, Japan |
Urban Planning Big Data Applications |
Data-driven urban planning, transportation modeling, simulation. Dubai, UAE |
Mobility data analysis, urban planning insights, visualization.
Singapore |
Data-driven urban planning, transportation modeling, simulation. Amsterdam, Netherlands |
Data-driven urban planning, transportation modeling, visualization. Toronto, Canada |
Safety and Security |
Public safety, incident management, emergency response |
Public safety, incident management, connected vehicle safety |
Public safety, emergency response, security analytics |
Public safety, traffic safety, security infrastructure |
Note: Compiled by authors from answers provided by Google’s Gemini to the prompts: “compare in matrix format MS CityNext, CISCO Intelligent Urbanization, IBM Smart City and Google’s Smart City in terms of: Connected transportation, intelligent traffic management, public transit optimization, smart parking, EVs and AVs, MaaS, predictive maintenance for transportation, integrated mobility platforms, urban planning . . .” and “provide actual city/region and category by company.” Note: Gemini offered URLs to sources, but the URLs were invalid or had been removed. The city information was unverifiable. |
CONCLUSION
The evolution of transportation technologies has profoundly shaped the urban landscapes of the United States. Each era, driven by advancements in power sources and infrastructure, has not only accelerated travel but also redefined urban development patterns. The advent of ICT marks another pivotal moment, fostering smart city solutions and reimagining mobility through innovations like autonomous vehicles and ride-hailing services. As we continue to navigate these technological frontiers, understanding their complex interplay with urban form and societal dynamics remains crucial for shaping sustainable and equitable cities of the future.
Shared mobility and its integration with advancing technologies represents a pivotal shift in urban transportation paradigms. Shared mobility, encompassing diverse modes from traditional carpooling to futuristic autonomous vehicles, promises to address critical urban challenges such as traffic congestion, environmental sustainability, and equitable access to transportation. Similarly, Mobility as a Service (MaaS) exemplifies a transformative approach where seamless integration of various transport modes through digital platforms enhances convenience and accessibility for users. However, this integration also introduces complex challenges, including data security, privacy concerns, and the equitable distribution of benefits across socio-economic strata.
Furthermore, the promise of Shared Autonomous Vehicles (SAVs) holds potential to further revolutionize urban mobility by reducing reliance on personal car ownership and enhancing mobility options, particularly for marginalized groups such as the elderly and economically disadvantaged. Yet, amid these technological advancements and their promises, it is essential to navigate carefully, mindful of the ethical, social, and environmental implications inherent in smart city initiatives. The need for transparency, accountability, and public engagement remains paramount to ensure that smart city solutions genuinely serve the interests of all residents while addressing historical inequalities and promoting sustainable urban development.
As cities continue to innovate and adapt, striking a balance between technological innovation and human-centric urban planning will be crucial in shaping inclusive and resilient urban futures. Ultimately, smart city initiatives, like the previous transportation regimes, have the potential to redefine urban landscapes, provided they are implemented with foresight, sensitivity to community needs, and a commitment to sustainability and equity.
QUIZ
Exercises
GLOSSARY
Aerotropolis: An airport metropolitan subregion with aviation-linked infrastructure, land use, economy, businesses, etc. (“Aerotropolis,” 2024).
Artificial intelligence (AI): A field of research in computer science that develops and studies methods and software that enable machines to perceive their environment and use learning and intelligence to take actions that maximize their chances of achieving defined goals (“Artificial intelligence,” 2024).
Bike sharing provides either one-time or ongoing membership-based access to bicycles to enable micro-mobility, predominately in urban areas. Riders access bicycles for one-way (point-to-point) or roundtrip use with pickup and drop-off locations either utilizing docking stations or freestanding locations.
Cloud computing: the on-demand availability of computer system resources, especially data storage (cloud storage) and computing power, without direct active management by the user. Large clouds often have functions distributed over multiple locations, each of which is a data center (“Cloud computing,” 2024).
Digital Divide: The unequal access to digital technology, including smartphones, tablets, laptops, and the internet. The digital divide worsens inequality around access to information and resources (“Digital Divide,” 2024).
Digital Nomad: Someone who can work from anywhere and takes advantage of this to relocate their home or continuously travel.
Digitization: The process of converting, storing, and analyzing information in a digital format able to be manipulated by computers.
Economies of Speed: Whereas economies of scale leverage efficiencies in process improvement, duplication, and quantity, economies of speed prioritize quickly prototyping, piloting, and pivoting to produce innovative practices that are responsive to real-time data and meet customer demands for time-sensitive deliveries.
First Mile / Last Mile: The first part of a commute trip before connecting to a fixed-route transit service and the final segment of the trip indicating the gaps in reaching a destination not directly proximal to the transit line or route.
Futurama: Futurama was an exhibit and ride at the 1939 New York World’s Fair designed by Norman Bel Geddes, which presented a possible model of the world 20 years into the future (1959–1960). The installation was sponsored by the General Motors Corporation and was characterized by automated highways and vast suburbs (“Futurama [New York World’s Fair],” 2024).
Information and Communication Technologies (ICTs): Information technology that serves the role of communications. The term has been utilized since the 1980s and evolved over time to adapt as new technologies have been introduced into the communications landscape from telephones and fax machines to computer networks, the Internet, and videoconferencing.
Hypermobility: Mobility at greater scales that travels at faster speeds. High Speed Rail and proposals like The Hyperloop (https://en.wikipedia.org/wiki/Hyperloop) would be considered hypermobility (“Hyperloop,” 2024).
Internet of Things (IoT): Devices with sensors, processing ability, software and other technologies that connect and exchange data with other devices and systems over the Internet or other communications networks. Relevant to transportation, IoT can assist in the integration of communications, control, and information processing across various transportation systems and all aspects comprising the system (i.e., the vehicle, the infrastructure, and the driver or user). Dynamic interaction between these components of a transport system enables inter- and intra-vehicular communication, smart traffic control, smart parking, electronic toll collection systems, logistics and fleet management, vehicle control, safety, and road assistance (“Internet of Things,” 2024).
Mobility as a service (MaaS) is a type of service that enables users to plan, book, and pay for multiple types of mobility services through a combined platform or app. The key concept behind MaaS is to offer travelers flexible mobility solutions based on their travel needs. Thus, “mobility as a service” also refers to the broader concept of a shift away from personally owned modes of transportation and towards mobility provided as a (shared) service (“Mobility as a service,” 2024). Mobility as a service. (2024, August 2). In Wikipedia. https://en.wikipedia.org/wiki/Mobility_as_a_service
Micro-mobility: Mobility at smaller neighborhood scales that typically utilizes small, lightweight transportation modes that travel at slower speeds. Human-powered or electric bicycles, kick-scooters, and skateboards that are either privately owned or part of shared fleets would be considered micromobility.
Shared Autonomous Vehicle (SAV): Shared Autonomous Vehicles are sometimes referred to as autonomous taxis. The concept is not just a single vehicle, but rather a system and service that provides shared mobility via autonomous vehicles, commonly called self-driving cars although the functional level of automation may vary as technologies continue to evolve (Carrese et al., 2023).
Shared mobility: This term is used for any mode which is not privately owned and may be rented sequentially or shared simultaneously. Rental cars, bikeshare or ride-hail services could all be considered shared mobility. The introduction of applications (Apps) on smart phones and mobile devices has facilitated the increased use of shared mobility modes.
Smart Cities: City planning, and operations utilizing ICT to collect data to manage and improve efficiencies of services such as transportation, utilities, waste management, and criminal investigations. Connected services collect data from citizens, buildings, and other city service nodes and analyze the trends and flows of resources to predict and provide more efficient services (“Smart city,” 2024).
Streetcar suburb: a residential community whose growth and development were strongly shaped by the use of streetcar lines as a primary means of transportation. Such suburbs developed in the United States in the years before the automobile, when the introduction of the electric trolley or streetcar allowed the nation’s burgeoning middle class to move beyond the central city’s borders (“Streetcar suburb,” 2024).
Tele-activities: The emerging activities including transportation technologies, social media, shared economy, teleworking, and online shopping enabled by the widespread adoption of mobile applications (apps).
Telecommuting: Working from home or another non-workplace location while using the Internet, email, and the telephone.
Time-sensitive: Deliveries of goods or services as soon as possible, as exemplified by Amazon’s Prime same-day delivery.
Transportation Network Companies (TNCs): Organizations that link drivers utilizing their vehicles with clients via an internet platform (such as a smartphone app) to provide planned transportation services for payment. (FHWA, 2016)
Transportation Regime: A standardized way or dominant mode of personal mobility including the technological, industrial, political, and cultural elements of transportation (Hoffmann, Weyer, & Longen, 2017).
Paratransit: offers transportation for those with disabilities who cannot use the region’s standard fixed-route transit service. Paratransit often provides door-to-door service for those who call to make a reservation (ICI, 2016).
Urban Sprawl: Urban sprawl is characterized by uncontrolled or loosely regulated growth extending towards suburban or exurban peripheries, encompassing housing, jobs, retail, industrial, and other urban developments, as well as extensive highway and road networks.
Zoom Town is a municipality where the population increased through the in-migration of remote workers, especially during the COVID-19 pandemic (“Zoom to
REFERENCES
Adkins, S. (2022, June 18). IBM Smart City [Video]. Vimeo. https://vimeo.com/721670104
Aerotropolis. (2024, June 29). In Wikipedia. https://en.wikipedia.org/wiki/Aerotropolis
Allosso, D. (n.d.) American environmental history. https://mlpp.pressbooks.pub/americanenvironmentalhistory/
Andreev, P., Salomon, I., & Pliskin, N. (2010). Review: State of teleactivities. Transportation Research Part C: Emerging Technologies, 18(1) 3-20. https://doi.org/10.1016/j.trc.2009.04.017
Arranz-Lopez, A. & Soria-Lara, J. (2022). ICT use and spatial fragmentation on activity participation in post-COVID-19 urban societies. Land Use Policy, 120, 106302. https://doi.org/10.1016%2Fj.landusepol.2022.106302
Artificial intelligence (AI). (2024, July 14). In Wikipedia. https://en.wikipedia.org/wiki/Artificial_intelligence
Audirac, I. (2005). Information technology and urban form: Challenges to Smart Growth. International Regional Science Review (IRSR), 28(2). https://doi.org/10.1177/0160017604273624
Barrero, J. M., Bloom, N., & Davis, S. J. (2021). Why working from home will stick. National Bureau of Economic Research (NBER) Working Paper Series. https://www.nber.org/system/files/working_papers/w28731/w28731.pdf
Ben-Elia, E., Alexander, B., Hubers, C. & Ettema, D. (2014). Activity fragmentation, ICT, and travel: An exploratory Path Analysis of spatiotemporal interrelationships. Transportation Research Part A: Policy and Practice, 68, 56-74. https://doi.org/10.1016/j.tra.2014.03.016
Benjamin, R. (2019). Race After Technology: Abolitionists tools for the New Jim Code. Pollity.
Bloom, N. (2020). How working from home works out. Stanford Institute for Economic Policy Research (SIEPR). https://siepr.stanford.edu/publications/policy-brief/how-working-home-works-out
Burchell, R.W., Shad. N.A., Listokin, D., Phillips, H., Downs., A., Seskin, S. Davis, J.S., Moore, and Gall, M. (1998). The costs of sprawl revisited. Transportation Research Board, TCRP Report 39. National Academy Press. https://onlinepubs.trb.org/onlinepubs/tcrp/tcrp_rpt_39-a.pdf
Carrese, F., Sportiello, S., Zhaksylykov, T., Colombaroni, C., Carrese, S., Papaveri, M., and Patella, S. M. (2023). The integration of shared autonomous vehicles in public transportation services: A systematic review. Sustainability, 15(17), 13023. https://doi.org/10.3390/su151713023
Circella, G., Iogansen, X., Makino, K., Compostella, J., Young, M., & Malik, J. K. (2023). Investigating the temporary and longer-term impacts of the COVID-19 pandemic on mobility in California. Research Report from the National Center for Sustainable Transportation. https://escholarship.org/content/qt0xm768km/qt0xm768km.pdf?t=rwx93v
Circella, G., & Mokhtarian, P. (2017). Impacts of information and communication technology. The geography of urban transportation (p.86).
Cloud computing. (2024, July 14). In Wikipedia. https://en.wikipedia.org/wiki/Cloud_computing
Digital divide. (2024, July 5). In Wikipedia. https://en.wikipedia.org/wiki/Digital_divide
Digitization. (2024, June 4). In Wikipedia. https://en.wikipedia.org/wiki/Digitization
Dill, J., Mathez, A., McNeil, N., & Howland, S. (2015). Who uses peer-to-peer car sharing? An early exploration. Transportation Research Part C: Emerging technologies, 54, 183-189. https://core.ac.uk/download/pdf/81252983.pdf
English, J. (2019, August 29). The commuting principle that shaped urban history. Bloomberg CitylabMaplab. https://www.bloomberg.com/news/features/2019-08-29/the-commuting-principle-that-shaped-urban-history
European Commission (2024). Mobility strategy. Directorate-General for Mobility and Transport. https://transport.ec.europa.eu/transport-themes/mobility-strategy_en
Fagnant, D. J., & Kockelman, K. (2015). Preparing a nation for autonomous vehicles: Opportunities, barriers, and policy recommendations. Transportation Research Part A: Policy and Practice, 77, 167–181. https://doi.org/10.1016/j.tra.2015.04.003.
Fleming, K. L. (2018). Social equity considerations in the new age of transportation: Electric, automated, and shared mobility. Journal of Science Policy & Governance, 13(1). https://www.sciencepolicyjournal.org/uploads/5/4/3/4/5434385/fleming.pdf
Futurama (New York World’s Fair). (2024, July 1). In Wikipedia. https://en.wikipedia.org/wiki/Futurama_(New_York_World%27s_Fair)
Grabar, H. (2023). Paved paradise: How parking explains the world. Penguin Press.
Green, B. (2019). The Smart Enough City: Putting Technology in its Place to Reclaim Our Urban Future. MIT Press https://smartenoughcity.mitpress.mit.edu/
Green, B. (2020, October 12). The smart enough city [Video]. Vimeo. https://vimeo.com/672748826
Harris, C. D., & Ullman, E. L. (1945). The nature of cities. The Annals of the American Academy of Political and Social Science, 242, 7-17. https://doi.org/10.1177/000271624524200103
Hoffmann, S., Weyer, J., & Longen, J. (2017). Discontinuation of the automobility regime? An integrated approach to multi-level governance. Transportation Research Part A: Policy and Practice, 103. https://doi.org/10.1016/j.tra.2017.06.016
Hoyt, H. (1939) The Structure and Growth of Residential Neighborhoods in American Cities. Federal Housing Administration
Hyperloop. (2024, July 12). In Wikipedia. https://en.wikipedia.org/wiki/Hyperloop
Internet of Things (IoT). (2024, July 5). In Wikipedia. https://en.wikipedia.org/wiki/Internet_of_things
Jerow, R. (2023, November 06). The evolution of freight logistics: How technology is transforming the industry. GoShip Blog https://www.goship.com/blog/freight-logistics-technology/
Kasarda, J. (2019). Aerotropolis. Wiley. https://doi.org/10.1002/9781118568446.eurs0436
Karlsson, I. C. M., Mukhtar-Landgren, D., Smith, G., Koglin, T., Kronsell, A., Lund, E., Sarasini, S., & Sochor, J. (2020). Development and implementation of Mobility-as-a-Service – A qualitative study of barriers and enabling factors. Transportation Research Part A: Policy and Practice, 131. https://doi.org/10.1016/j.tra.2019.09.028
Knowles, R. D., Ferbrache, F., & Nikitas, A. (2020). Transport’s historical, contemporary, and future role in shaping urban development: Re-evaluating transit-oriented development. Cities, 99, Article 102607, https://doi.org/10.1016/j.cities.2020.102607
Kong, H., Moody, J., & Zhao, J. (2020). ICT’s impacts on ride-hailing use and individual travel. Transportation Research Part A: Policy and Practice, 141, 1-15. https://doi.org/10.1016/j.tra.2020.08.012
Kreyon. (2017, May 10). ICT applications for smart city. https://www.kreyonsystems.com/Blog/ict-applications-for-smart–city/
Le, Huyen T. K, Carrel, Andre L. & Shah, Harsh (2022). Impacts of online shopping on travel demand: a systematic review, Transport Reviews, 42:3, 273-295, http://dx.doi.org/10.1080/01441647.2021.1961917
Lee, Y. & Circella, G. (2019). ICT, millennials’ lifestyles, and travel choices. Advances in Transport Policy and Planning, 3. 107-141. https://doi.org/10.1016/bs.atpp.2019.08.002
Milakis, D., Van Arem, B., & Van Wee, B. (2017). Policy and society related implications of automated driving: A literature review and directions for future research. Journal of Intelligent Transportation Systems, 21(4), 324–348. https://doi.org/10.1080/15472450.2017.1291351
Mobility as a service. (2024, August 2). In Wikipedia. https://en.wikipedia.org/wiki/Mobility_as_a_service
Mokhtarian, P. L. (1990). A typology of relationships between telecommunications and transportation. Transportation Research Part A, 24, 231-242. https://doi.org/10.1016/0191-2607(90)90060-J
Mokhtarian, P. L. (2009). Social networks and telecommunications. Handbook of Research on Social Interaction Technologies and Collaboration Software: Concepts and Trends, 429–438. https://doi.org/10.4018/978-1-60566-264-0.ch030
Mokhtarian, P., Salomon, I., & Handy, S. L. (2004). A taxonomy of leisure activities: The role of ICT. ITS UC Davis Institute of Transportation Studies. https://escholarship.org/uc/item/0nk475qx
Moura, F. and De Abreu e Silva, J (2019). Smart Cities: Definitions, evolution of the concept and examples of initiatives. Industry, Innovation and Infrastructure Encyclopedia of the UN Sustainable Development Goals, 2019, p. 1-9. https://doi.org/10.1007/978-3-319-71059-4_6-1
Mouratidis, K. Peters, S., van Wee, B. (2021). Transportation technologies, sharing economy, and teleactivities: Implications for built environment and travel. Transportation Research Part D: Transport and Environment, 92, 102716. https://doi.org/10.1016/j.trd.2021.102716
Narayanan, S., Chaniotakis, E., & Antoniou, C. (2020). Shared autonomous vehicle services: A comprehensive review. Transportation Research Part C: Emerging Technologies, 111, 255–293. https://doi.org/10.1016/j.trc.2019.12.008
National Highway Traffic Safety Administration (NHTSA). (2021). Automated vehicles for safety. https://www.nhtsa.gov/technology-innovation/automated-vehicles-safety
Ozbilen, B. Slagle, K. M., Akar, G. (2021). Perceived risk of infection while traveling during the COVID-19 pandemic: Insights from Columbus, OH. Transportation Research Interdisciplinary Perspective,10. https://doi.org/10.1016/j.trip.2021.100326
Paiva, S., Ahad, M. A., Zafar, S, Tripathi, G., Khalique, A., & Hussain, I. (2020). Privacy and security challenges in smart and sustainable mobility. Springer Nature Switzerland AG, 2. https://doi.org/10.1007/s42452-020-2984-9
Patel, R. K., Etminani-Ghasrodashti, R., Kermanshachi, S., Rosenberger, J. M., & Foss, A. (2022). Modeling users’ adoption of shared autonomous vehicles employing actual ridership experiences. Journal of Transport Geography, 96, https://ascelibrary.org/doi/10.1061/9780784484876.022
Paul, J. (2023). Hitchhiking on the digital highway: assessing the costs and benefits of informal ridesharing. Transportation. https://doi.org/10.1007/s11116-023-10446-5
Patwary, A. L., & Khattak, A., J. (2022). Interaction between information and communication technologies and travel behavior: Using behavioral data to explore correlates of the COVID-19 pandemic. Transportation Research Record, https://doi.org/10.1177/03611981221116626
Pawlak, J., Circella, G., Mahmassani, H., & Mokhtarian, P. (2020). Information and communication technologies (ICT), activity decisions, and travel choices: 20 years into the second millennium and where do we go next. Washington, DC: http://Onlinepubs.Trb.Org/Onlinepubs/Centennial/Papers/ADB20-Final.Pdf.
Pew Research Center. (2016, June 22). What do consumers without bank accounts think about mobile payments? http://pew.org/2667iU1.
Salomon, I. (1986). Telecommunications and travel relations: A review. Transportation Research Part A 20(3). 223-238. https://doi.org/10.1016/0191-2607(86)90096-8
Sassen, S. (1995). The state and the global city: Notes towards a conception of place-centered governance. Competition & Change, 1(1). https://doi.org/10.1177/102452949500100103
Schmitt, A. (2012, October 3). Why isn’t bike share reaching more low-income people? Streetsblog USA. https://usa.streetsblog.org/2012/10/03/why-isnt-bike-share-reaching-more-low-income-people/.
Sector Model. (2024, February 16). In Wikipedia. https://en.wikipedia.org/wiki/Sector_model
Shaheen, S. A., Martin, E. W., Cohen, A. P., Chan, N. D., & Pogodzinski, M. (2014). Public bike sharing in North America during a period of rapid expansion: Understanding business models, industry trends and user impacts. (MTI Report 12-29). Mineta Transportation Institute, College of Business, San José State University. https://transweb.sjsu.edu/sites/default/files/1131-public-bikesharing-business-models-trends-impacts.pdf
Shaheen, S., & Chan, N. (2016). Mobility and the sharing economy: Potential to facilitate the first-and last-mile public transit connections. Built Environment, 42(4), 573–588. http://www.jstor.org/stable/44131893.
Shaheen, S., Cohen, A., & Zohdy, I. (2016). Shared mobility: current practices and guiding principles (No. FHWA- HOP-16-022). United States. Federal Highway Administration.
Shaheen, S., Bell, C., Cohen, A., & Yelchuru, B. (2017). Travel behavior: Shared mobility and transportation equity. Federal Highway Administration, U.S. Department of Transportation.
Shared-Use Mobility Center (SUMC). (2019). Equity and shared mobility services: Working with the private sector to meet equity objectives. Shared-Use Mobility Center Report. https://sharedusemobilitycenter.org/wp-content/uploads/2019/12/EquitySharedMobilityServices-FINAL.pdf
Smart city. (2024, July 15). In Wikipedia. https://en.wikipedia.org/wiki/Smart_city
Streetcar suburb. (2024, May 25). In Wikipedia. https://en.wikipedia.org/wiki/Streetcar_suburb
United States (U.S.) Census Bureau (2021, March 18). Census Bureau estimates show average one-way travel time to work rises to all-time high. Press Release Number CB21-TPS.29. https://www.census.gov/newsroom/press-releases/2021/one-way-travel-time-to-work-rises.html
United States (US) Department of Energy (DOE) (2023). The U.S. National Blueprint for Transportation Decarbonization. A joint strategy to transform transportation. https://www.energy.gov/eere/us-national-blueprint-transportation-decarbonization-joint-strategy-transform-transportation
Urban Sprawl. (2024 March 4). In Wikipedia. https://en.wikipedia.org/wiki/Urban_sprawl
Wachter, S. (2019). What’s fueling the smart city backlash? Knowledge Wharton, September 24, 2019. https://knowledge.wharton.upenn.edu/article/whats-behind-backlash-smart-cities/
Weltevreden, J. W. J., & Rietbergen, T. V. (2007). E-shopping versus city center shopping: The role of perceived city attractiveness. Journal of Economics and Human Geography, 98(1), 68–85.
Wladawsky-Berger, I. (2023, September 07). Will work from home stick? Irving Wladawsky-Berger Blog. https://blog.irvingwb.com/blog/2023/09/will-work-from-home-stick.html
Wong, R. (2020, June 14). Sidewalk Labs Toronto – A brief history [Video]. YouTube. https://www.youtube.com/watch?v=Nmjtf9v-YFQ
Ziosi, M., Hewitt, B. Juneja, P., Taddeo, M., & Florida, L. (2022). Smart cities: Mapping their ethical implications. SSRN. http://dx.doi.org/10.2139/ssrn.4001761
Zoom town (2023, July 30). In Wikipedia. https://en.wikipedia.org/wiki/Zoom_town
Zoning. (2024, March 10). In Wikipedia. https://en.wikipedia.org/wiki/Zoning
Information technology that serves the role of communications. The term has been utilized since the 1980s and evolved over time to adapt as new technologies have been introduced into the communications landscape from telephones and fax machines to computer networks, the Internet, and videoconferencing.
Shared Autonomous Vehicles are sometimes referred to as autonomous taxis. The concept is not just a single vehicle, but rather a system and service that provides shared mobility via autonomous vehicles, commonly called self-driving cars although the functional level of automation may vary as technologies continue to evolve (Carrese et al., 2023).
Smart Cities: City planning and operations utilizing ICT to collect data to manage and improve efficiencies of services such as transportation, utilities, waste management, and criminal investigations. Connected services collect data from citizens, buildings, and other city service nodes and analyze the trends and flows of resources to predict and provide more efficient services (“Smart city,” 2024).
Transportation regime: A standardized way or dominant mode of personal mobility, including the technological, industrial, political, and cultural elements of transportation (Hoffmann, Weyer, & Longen, 2017).
Streetcar suburb is a residential community whose growth and development were strongly shaped by the use of streetcar lines as a primary means of transportation. Such suburbs developed in the United States in the years before the automobile, when the introduction of the electric trolley or streetcar allowed the nation’s burgeoning middle class to move beyond the central city’s borders (“Streetcar suburb,” 2024).
Urban sprawl is characterized by uncontrolled or loosely regulated growth extending towards suburban or exurban peripheries, encompassing housing, jobs, retail, industrial, and other urban developments, as well as extensive highway and road networks.
An airport metropolitan subregion with aviation-linked infrastructure, land use, economy, businesses, etc. (“Aerotropolis,” 2024).
Mobility at greater scales that travels at faster speeds. High Speed Rail and proposals like The Hyperloop (https://en.wikipedia.org/wiki/Hyperloop) would be considered hypermobility (“Hyperloop,” 2024).
Economies of speed: Whereas economies of scale leverage efficiencies in process improvement, duplication, and quantity, economies of speed prioritize quickly prototyping, piloting, and pivoting to produce innovative practices that are responsive to real-time data and meet customer demands for time-sensitive deliveries.
Time-sensitive: Deliveries of goods or services as soon as possible, as exemplified by Amazon’s Prime same-day delivery.
Transportation Network Companies (TNCs): Organizations that link drivers utilizing their vehicles with clients via an internet platform (such as a smartphone app) to provide planned transportation services for payment (FHWA, 2016).
Digitization: The process of converting, storing, and analyzing information in a digital format able to be manipulated by computers.
Telecommuting: Working from home or another non-workplace location while using the Internet, email, and the telephone.
The emerging activities including transportation technologies, social media, shared economy, teleworking, and online shopping enabled by the widespread adoption of mobile applications (apps).
Shared mobility: This term is used for any mode that is not privately owned and may be rented sequentially or shared simultaneously. Rental cars, bikeshare, or ride-hail services could all be considered shared mobility. The introduction of applications (Apps) on smartphones and mobile devices has facilitated the increased use of shared mobility modes.
Mobility at smaller, neighborhood scales that typically utilizes small, lightweight transportation modes that travel at slower speeds. Human-powered or electric bicycles, kick-scooters, and skateboards that are either privately owned or part of shared fleets would be considered micromobility.
The first part of a commute trip before connecting to a fixed-route transit service and the final segment of the trip indicating the gaps in reaching a destination not directly proximal to the transit line or route.
Mobility as a service (MaaS) is a type of service that enables users to plan, book, and pay for multiple types of mobility services through a combined platform or app. The key concept behind MaaS is to offer travelers flexible mobility solutions based on their travel needs. Thus, "mobility as a service" also refers to the broader concept of a shift away from personally owned modes of transportation and towards mobility provided as a (shared) service ("Mobility as a service," 2024).
The digital divide is the unequal access to digital technology, including the internet, tablets, laptops, smartphones, and smartphone applications ("Digital divide," 2024).
Futurama was an exhibit and ride at the 1939 New York World's Fair designed by Norman Bel Geddes, which presented a possible model of the world 20 years into the future (1959–1960). The installation was sponsored by the General Motors Corporation and was characterized by automated highways and vast suburbs (“Futurama [New York World’s Fair],” 2024).