2 Analyzing Microbiomes
Analyzing Microbiomes
Choosing the correct approach when characterizing a microbiome is important when considering what questions are trying to be answered. Who are the players? What is the function? What genes are being expressed? How do they interact?
Even though when assessing a microbiome all members of the microbiota should be considered, some insight can be gained by viewing individual groups, such as the prokaryotic component, which can be further compartmentalized into the bacteriome and archaeome. In some cases, it is difficult to completely characterize all of the members due to inherent biological differences between these communities, and in other situations, certain groups may be absent or irrelevant to the study. In so, analyzing these individual pieces could help reveal their distinct importance and contribution to the overall big picture.
The type of analysis also depends on the research question. If the sole interest is to identify all the bacteria in a sample, then a metagenomic approach may work best. Whereas, if an interest lies in what the microorganisms are doing, then a metatranscriptomics or metaproteomics approach would be more appropriate. If there is an interest in one or a few particular members of the microbiome, culture-based techniques (culturomics) could be implemented, which can often catch rare microbes that are missed using sequencing techniques because of data filtering protocols (Lagier et al., 2012, Allaband et al., 2019). However, it is usually difficult to characterize an entire microbiome based on just a few microbes and achieving multiple isolations is extremely difficult as a ‘one-size-fits-all’ type media does not exist.
Sample Collection
Depending on the type of research questions and the particular microbiome of interest, there are different ways to collect microbial communities that will be subjected to analysis. That is, the approach to collecting microbes from the human gut is much different than getting a soil sample. Really, there is no perfect method for any one microbiome, though some may be more difficult to obtain than others, and will depend on a variety of factors such as feasibility, cost, patient acceptance, and downstream analytical methods (Allaband et al., 2019). It can also be very difficult to capture all microbes within a microbiome sample, as inevitably some will be missed or cannot be collected by a particular technique. For example, when analyzing the gut microbiome, stool collection is preferred due to its ease and frequent accessibility, but this method often misses microbes in the small intestine and others which are mucosally adherent as opposed to excreted (Eckburg et al., 2005, de Carcer et al., 2010, Allaband et al., 2019). Additionally, collection and storage materials, storage time, and transport options should be considered as these can compromise sample integrity and create problems for various analytical approaches (Costello et al., 2009, Caporaso et al., 2011, McDonald et al., 2018).
Approaches to Characterization
What is the Difference between Culture-Dependent and Independent Methods of Microbial Community Characterization?
-Excerpt taken from Findley and Grice, 2014 available under the Creative Commons CC0 public domain dedication:
Up until the 1980s, microbiologists routinely relied on culture-dependent methods for microbial isolation, identification, and characterization. Colony morphology, stains (i.e., Gram stain), biochemical characteristics (i.e., coagulase test), motility tests, antibiotic resistance profiles, and other characteristics guided bacterial and/or fungal identification and taxonomy. However, this approach has several limitations, including an inability to mimic in vivo conditions and selection against slow-growing and/or fastidious organisms. With recent advances in sequencing technologies and development of bioinformatics tools and reference databases, researchers are now better equipped to capture microbial diversity without the biases of culture-based approaches.
Culture-independent methods of microbial identification rely on a targeted amplicon strategy, which employs highly conserved microbe-specific molecular markers and does not rely on growing isolates in pure culture. The 16S ribosomal RNA (rRNA) gene is used for bacterial identification, while fungi and other microeukaryotes are identified using either the 18S rRNA gene or the Internal Transcribed Spacer (ITS) region. A complementary approach to amplicon-based surveys is whole genome shotgun metagenomics. With this approach, one can identify the microbiota present and gain insight into the functional potential of the microbiota in an untargeted manner.
Within the culture-independent targeted approach, PCR amplification techniques, like quantitative PCR (qPCR) and reverse transcriptase PCR (RT-PCR) can be implemented to detect and quantify specific organisms, genes, or expression values within the microbiome sample. Untargeted approaches typically give whole community characterization and include metagenomics, metatranscriptomics, metaproteomics, and metabolomics which have a variety of applications depending on the type of study.
Culturomics
Cultivating all the microorganisms within a certain microbiome can be quite challenging and time-consuming, but nonetheless can provide important information that would otherwise be missed with other approaches. Creating media that mimics the original environment can help to capture organisms that exist at lower concentrations than that of the detectable threshold of molecular tools (Lagier et al., 2012). The application of matrix-assisted laser desorption ionization time-of-flight mass spectrometry (MALDI-TOF MS) has enhanced culture-dependent studies by allowing fast and reliable identification of bacterial taxa in a cost-effective manner (Seng et al., 2009, Lagier et al., 2012). Even though there have been advances in culturomics, the approach still has certain biases based on the types of microbes that can be easily grown (e.g. aerobic microbes are much easier to grow than anaerobic ones in most cases), as well as the selection for those that grow more rapidly and outcompete the others in a sample (Allaband et al., 2019). Thusly, it is imperative to close the gap between microbial richness observed in nature versus what is cultivable in the lab in order to better understand in vivo microbial functioning within a microbiome (Sarhan et al., 2019).
Metagenomics and DNA sequencing
Next-generation sequencing (NGS), also known as high-throughput sequencing (HTS), allows the generation of thousands of sequencing reads in a cost-effective manner (Nkrumah-Elie, et al., 2018). These reads can then be bioinformatically processed and annotated to identify microbial taxa, genes, potential functions, etc. depending on the sample type and experimental conditions (Rivera-Pinto et al., 2018). However, differences in wet lab work, sequencing platforms, processing pipelines, and statistical approaches can affect data output, which make reproducibility come into question (Gloor et al., 2016). Usually, information about microbiome compositions are provided as relative abundances instead of the true abundances due to sequencing platform imitations. This data is considered an arbitrary sum and is referred to as compositional data (Jiang et al., 2019). As sequence generation and pipelines improve and become standardized, the development and accessibility for analytical tools can help to streamline and produce consistent and more accurate results while aggregating data for microbiome characterization and meta-analysis without advanced expertise (Markowitz et al., 2007, Dhariwal et al., 2017, Gonzalez et al., 2018, Chong et al., 2020, Mitchell et al., 2020, Bharti and Grimm, 2021, Chen et al., 2021).
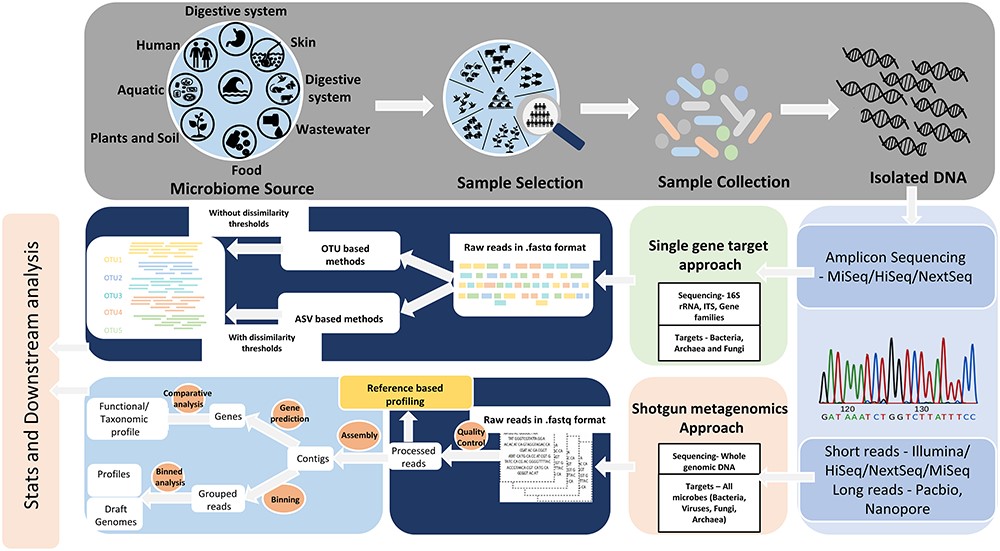
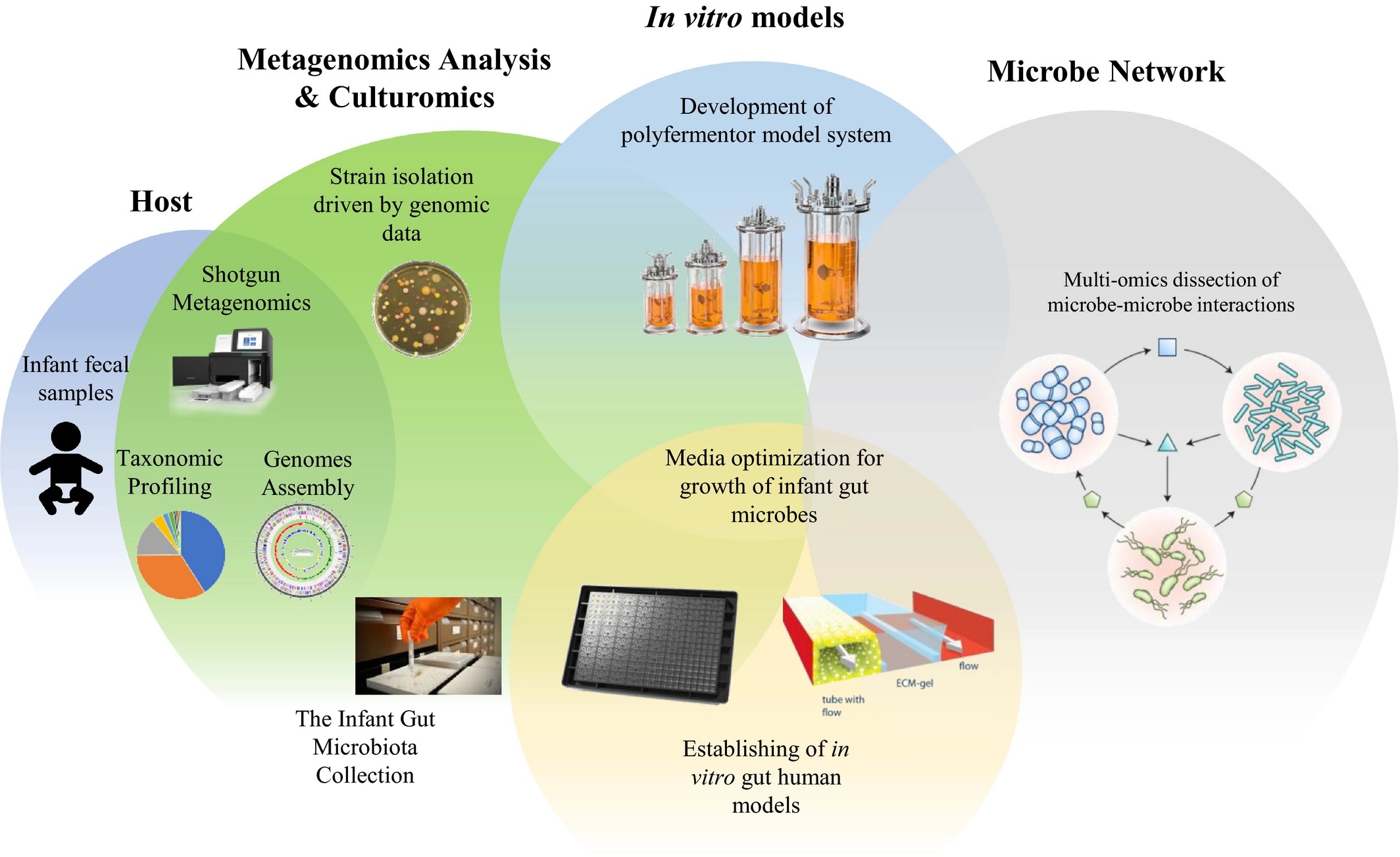
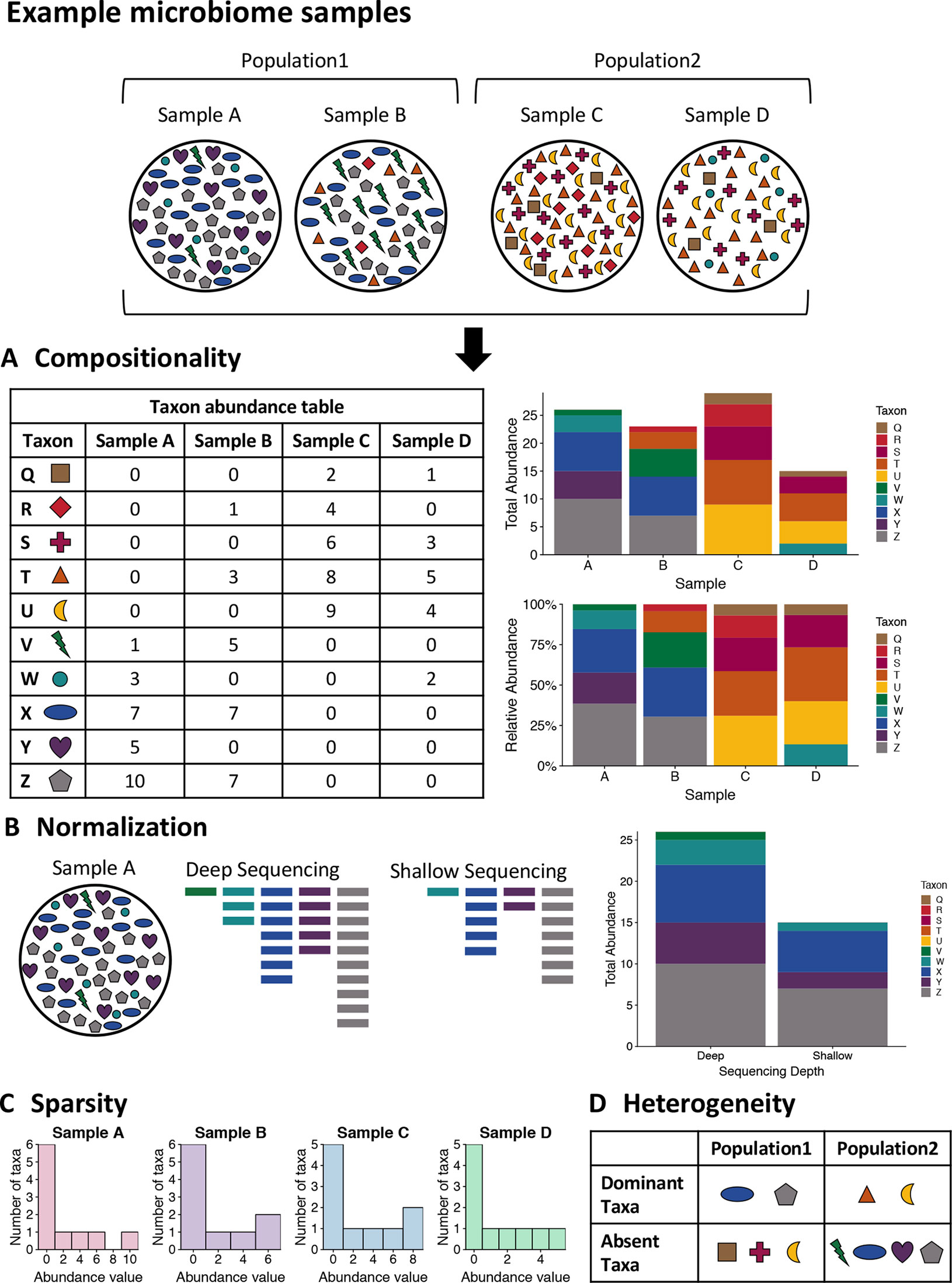
Metatranscriptomics and RNA sequencing
While metagenomic studies have been instrumental in identifying various microbes and genes in a given sample, it does not differentiate between viable cells or when those genes are being expressed (Gosalbes, et al., 2011, Bashiardes et al., 2016). Metatranscriptomic strategies of microbiome analysis allow researchers to capture gene activity which can delineate the functional dynamics of a particular community and it’s interactions with its host or environment. These RNA-based approaches can complement metagenomics, as the extent of expression of those annotated genes can be measured (Franzosa et al., 2014). This is considerably important concerning certain disease states because the presence of a pathogen does not always produce deleterious conditions, but rather it is the functional activity of certain organisms.
RNA-seq experiments usually begin with isolation of total RNA in a sample, then selection is based upon whether it is prokaryotic or eukaryotic in origin, and mRNA must be isolated from other RNA species (i.e. rRNA and tRNA) (Bashiardes et al., 2016). cDNA is then synthesized from the mRNA, adapters are ligated to create a library, amplification and sequencing follows, and the generated reads are mapped to a reference genome where expression can be measured (Giannoukos et al., 2012, Bashiardes et al., 2016). These experiments can be difficult and sensitive, as care must be taken with sample collection, and avoiding contamination and degradation from ribonucleases is important to maintain integrity of the samples as well. Furthermore, metatranscriptomics may not always give the whole picture of community expression due to the high complexity of organisms, the delicateness of RNA, the wide range of transcript expression, and various limitations with current technology (Shakya et al., 2019).
Metaproteomics
Metaproteomics gives a snapshot of the entire protein content of a microbiome sample under various conditions, which can further provide insight into gene activity and metabolic functions. This strategy identifies peptides by matching tandem mass spectrometry (MS/MS) data to protein sequence databases, which can be further assigned to functional groups via annotation databases (Sajulga et al., 2020). This approach can be convoluted though, as many peptides match to similar proteins produced by different organisms, and proteins can be multi-functional making it difficult for assignment to any one group. Additionally, bioinformatic analysis of metaproteomes isn’t exactly standardized which makes reproducibility and comparison of studies problematic (Schiebenhoefer et al., 2019, Sajulga et al., 2020).
Metabolomics
Metabolomics analyzes the non-protein metabolites that are produced and/or regulated by a given microbiome, which adds another angle of characterization to community-host-environmental interaction and functional dynamics. By understanding how various microbes process metabolites like natural products, nutrients, and medications, we can better determine how they influence their ecosystem through molecular interactions. Researchers typically obtain these molecules through gas or liquid chromatography and subsequently analyze them by mass spectrometry (Allaband et al., 2019, Bauermeister et al., 2021). Metabolomics strategies can either be targeted or untargeted, where the former is more sensitive and compares metabolites to a pre-determined bank with reference standards, however, it cannot detect novel molecules. Since most metabolites produced by microbiomes are unknown, an untargeted approach using tandem mass spectrometry is useful in detecting many small molecules, though annotation of modified metabolites is challenging (Watrous et al., 2012, Wang et al., 2016, Allaband et al., 2019). As with other microbiome analytical approaches, various data analysis tools and pipelines of the metabolome need to be standardized to a degree to improve overall research context. Moreover, major advancements in the known inventory of microorganism-derived metabolites and their functions are required to enhance metabolomics studies (Lee-Sarwar et al., 2020, Bauermeister et al., 2021).
Drag and Drop Quiz
Conclusion and Future Directions
While various approaches to characterizing a microbiome each have their advantages and drawbacks, network analyses integrating all the -omics (i.e. metagenomics, metatranscriptomics, metaproteomics, and metabolomics) could be best to gain a holistic view of compositional and functional dynamics (Bashiardes et al., 2016, Jiang et al., 2019). Novel and improved approaches to collect and analyze various microbiomes will help to minimize loss of information and provide a better picture of how these communities interact with and influence their host or environment. Also, the establishment of more comprehensive publicly available databases, and protocols for streamlining computational procedures will allow more consistent and reproducible research for upon which knowledge can be accumulated.
Check your Understanding
- What is the difference between culture-dependent and independent methods of microbiome analysis?
- What is the importance of culturomics in the scope of microbiome analysis?
- How can inaccurate or inconsistent results be produced from different metagenomic approaches to microbiome analysis?
- When would a metatranscriptomic analysis be more appropriate than a metagenomic approach? How do these approaches complement each other?
- What are the benefits and limitations of metabolomic analysis?
Media Attributions
- Figure 1 – Targeted amplicon and metagenomic sequencing approaches to microbiome analysis by Bharti and Grimm, 2021. Licensed under Creative Commons: By Attribution 4.0 License http://creativecommons.org/licenses/by/4.0/
- Figure 2 – In Vitro Model Schematic by Ventura et al., 2020. Licensed under Creative Commons: By Attribution 4.0 License http://creativecommons.org/licenses/by/4.0/
- Figure 3 – Example microbiome samples and challenge of analysis by Jiang et al., 2019. Licensed under Creative Commons: By Attribution 4.0 License http://creativecommons.org/licenses/by/4.0/
- Video 1 – Life in the Lab: Working with human gut microbiota by yourgenome. Licensed under Creative Commons: By Attribution 3.0 License https://creativecommons.org/licenses/by/3.0/
- Video 2 – New technique explores gut microbiome one microbe at a time by Research Square. Licensed under Creative Commons: By Attribution 3.0 License https://creativecommons.org/licenses/by/3.0/
References
- Allaband, C., McDonald, D., Vázquez-Baeza, Y., Minich, J. J., Tripathi, A., Brenner, D. A., Loomba, R., Smarr, L., Sandborn, W. J., Schnabl, B., Dorrestein, P., Zarrinpar, A., & Knight, R. (2019). Microbiome 101: Studying, Analyzing, and Interpreting Gut Microbiome Data for Clinicians. Clinical Gastroenterology and Hepatology, 17(2), 218–230. https://doi.org/https://doi.org/10.1016/j.cgh.2018.09.017
- Bashiardes, S., Zilberman-Schapira, G., & Elinav, E. (2016). Use of Metatranscriptomics in Microbiome Research. Bioinformatics and Biology Insights, 10, BBI.S34610. https://doi.org/10.4137/BBI.S34610
- Bauermeister, A., Mannochio-Russo, H., Costa-Lotufo, L. v, Jarmusch, A. K., & Dorrestein, P. C. (2021). Mass spectrometry-based metabolomics in microbiome investigations. Nature Reviews Microbiology. https://doi.org/10.1038/s41579-021-00621-9
- Bharti, R., & Grimm, D. G. (2021). Current challenges and best-practice protocols for microbiome analysis. Briefings in Bioinformatics, 22(1), 178–193. https://doi.org/10.1093/bib/bbz155
- Caporaso, J. G., Lauber, C. L., Costello, E. K., Berg-Lyons, D., Gonzalez, A., Stombaugh, J., Knights, D., Gajer, P., Ravel, J., Fierer, N., Gordon, J. I., & Knight, R. (2011). Moving pictures of the human microbiome. Genome Biology, 12(5), R50. https://doi.org/10.1186/gb-2011-12-5-r50
- Chen, I.-M. A., Chu, K., Palaniappan, K., Ratner, A., Huang, J., Huntemann, M., Hajek, P., Ritter, S., Varghese, N., Seshadri, R., Roux, S., Woyke, T., Eloe-Fadrosh, E. A., Ivanova, N. N., & Kyrpides, N. C. (2021). The IMG/M data management and analysis system v.6.0: new tools and advanced capabilities. Nucleic Acids Research, 49(D1), D751–D763. https://doi.org/10.1093/nar/gkaa939
- Chong, J., Liu, P., Zhou, G., & Xia, J. (2020). Using MicrobiomeAnalyst for comprehensive statistical, functional, and meta-analysis of microbiome data. Nature Protocols, 15(3), 799–821. https://doi.org/10.1038/s41596-019-0264-1
- Costello, E. K., L, L. C., Micah, H., Noah, F., I, G. J., & Rob, K. (2009). Bacterial Community Variation in Human Body Habitats Across Space and Time. Science, 326(5960), 1694–1697. https://doi.org/10.1126/science.1177486
- de Cárcer, D. A., Cuív, P. Ó., Wang, T., Kang, S., Worthley, D., Whitehall, V., Gordon, I., McSweeney, C., Leggett, B., & Morrison, M. (2011). Numerical ecology validates a biogeographical distribution and gender-based effect on mucosa-associated bacteria along the human colon. The ISME Journal, 5(5), 801–809. https://doi.org/10.1038/ismej.2010.177
- Dhariwal, A., Chong, J., Habib, S., King, I. L., Agellon, L. B., & Xia, J. (2017). MicrobiomeAnalyst: a web-based tool for comprehensive statistical, visual and meta-analysis of microbiome data. Nucleic Acids Research, 45(W1), W180–W188. https://doi.org/10.1093/nar/gkx295
- Eckburg, P. B., Bik, E. M., Bernstein, C. N., Purdom, E., Dethlefsen, L., Sargent, M., Gill, S. R., Nelson, K. E., & Relman, D. A. (2005). Diversity of the Human Intestinal Microbial Flora. Science, 308(5728), 1635–1638. https://doi.org/10.1126/science.1110591
- Findley, K., & Grice, E. A. (2014). The Skin Microbiome: A Focus on Pathogens and Their Association with Skin Disease. PLOS Pathogens, 10(11), e1004436-. https://doi.org/10.1371/journal.ppat.1004436
- Franzosa, E. A., Morgan, X. C., Segata, N., Waldron, L., Reyes, J., Earl, A. M., Giannoukos, G., Boylan, M. R., Ciulla, D., Gevers, D., Izard, J., Garrett, W. S., Chan, A. T., & Huttenhower, C. (2014). Relating the metatranscriptome and metagenome of the human gut. Proceedings of the National Academy of Sciences, 111(22), E2329. https://doi.org/10.1073/pnas.1319284111
- Giannoukos, G., Ciulla, D. M., Huang, K., Haas, B. J., Izard, J., Levin, J. Z., Livny, J., Earl, A. M., Gevers, D., Ward, D. v, Nusbaum, C., Birren, B. W., & Gnirke, A. (2012). Efficient and robust RNA-seq process for cultured bacteria and complex community transcriptomes. Genome Biology, 13(3), r23. https://doi.org/10.1186/gb-2012-13-3-r23
- Gloor, G. B., Wu, J. R., Pawlowsky-Glahn, V., & Egozcue, J. J. (2016). It’s all relative: analyzing microbiome data as compositions. Annals of Epidemiology, 26(5), 322–329. https://doi.org/10.1016/j.annepidem.2016.03.003
- Gonzalez, A., Navas-Molina, J. A., Kosciolek, T., McDonald, D., Vázquez-Baeza, Y., Ackermann, G., DeReus, J., Janssen, S., Swafford, A. D., Orchanian, S. B., Sanders, J. G., Shorenstein, J., Holste, H., Petrus, S., Robbins-Pianka, A., Brislawn, C. J., Wang, M., Rideout, J. R., Bolyen, E., … Knight, R. (2018). Qiita: rapid, web-enabled microbiome meta-analysis. Nature Methods, 15(10), 796–798. https://doi.org/10.1038/s41592-018-0141-9
- Gosalbes, M. J., Durbán, A., Pignatelli, M., Abellan, J. J., Jiménez-Hernández, N., Pérez-Cobas, A. E., Latorre, A., & Moya, A. (2011). Metatranscriptomic Approach to Analyze the Functional Human Gut Microbiota. PLOS ONE, 6(3), e17447-. https://doi.org/10.1371/journal.pone.0017447
- Iorio, A., Biazzo, M., Gardini, S., Muda, A. O., Perno, C. F., Dallapiccola, B., & Putignani, L. (2022). Cross-correlation of virome–bacteriome–host–metabolome to study respiratory health. Trends in Microbiology, 30(1), 34–46. https://doi.org/10.1016/j.tim.2021.04.011
- Jiang, D., Armour, C. R., Hu, C., Mei, M., Tian, C., Sharpton, T. J., & Jiang, Y. (2019). Microbiome Multi-Omics Network Analysis: Statistical Considerations, Limitations, and Opportunities. Frontiers in Genetics, 10. https://www.frontiersin.org/article/10.3389/fgene.2019.00995
- Lagier, J.-C., Armougom, F., Million, M., Hugon, P., Pagnier, I., Robert, C., Bittar, F., Fournous, G., Gimenez, G., Maraninchi, M., Trape, J.-F., Koonin, E. v, la Scola, B., & Raoult, D. (2012). Microbial culturomics: paradigm shift in the human gut microbiome study. Clinical Microbiology and Infection, 18(12), 1185–1193. https://doi.org/https://doi.org/10.1111/1469-0691.12023
- Lee-Sarwar KA, Lasky-Su J, Kelly RS, Litonjua AA, Weiss ST. Metabolome–Microbiome Crosstalk and Human Disease. Metabolites. 2020; 10(5):181. https://doi.org/10.3390/metabo10050181
- Markowitz, V. M., Ivanova, N. N., Szeto, E., Palaniappan, K., Chu, K., Dalevi, D., Chen, I.-M. A., Grechkin, Y., Dubchak, I., Anderson, I., Lykidis, A., Mavromatis, K., Hugenholtz, P., & Kyrpides, N. C. (2008). IMG/M: a data management and analysis system for metagenomes. Nucleic Acids Research, 36(suppl_1), D534–D538. https://doi.org/10.1093/nar/gkm869
- McDonald, D., Embriette, H., W, D. J., T, M. J., Antonio, G., Gail, A., A, A. A., Bahar, B., Caitriona, B., Yingfeng, C., Lindsay, D. G., C, D. P., R, D. R., K, F. A., James, G., A, G. J., Grant, G., L, G. J., Philip, H., … Beau, G. (2018). American Gut: an Open Platform for Citizen Science Microbiome Research. MSystems, 3(3), e00031-18. https://doi.org/10.1128/mSystems.00031-18
- Mitchell, A. L., Almeida, A., Beracochea, M., Boland, M., Burgin, J., Cochrane, G., Crusoe, M. R., Kale, V., Potter, S. C., Richardson, L. J., Sakharova, E., Scheremetjew, M., Korobeynikov, A., Shlemov, A., Kunyavskaya, O., Lapidus, A., & Finn, R. D. (2020). MGnify: the microbiome analysis resource in 2020. Nucleic Acids Research, 48(D1), D570–D578. https://doi.org/10.1093/nar/gkz1035
- Nkrumah-Elie, Y., Elie, M., & Reisdorph, N. (2018). Chapter 14 – Systems Biology Approaches to Asthma Management. In S. J. Szefler, F. Holguin, & M. E. Wechsler (Eds.), Personalizing Asthma Management for the Clinician (pp. 151–160). Elsevier. https://doi.org/10.1016/B978-0-323-48552-4.00014-7
- Rivera-Pinto, J., Egozcue, J. J., Pawlowsky-Glahn, V., Paredes, R., Noguera-Julian, M., Calle, M. L., & Catherine, L. (2022). Balances: a New Perspective for Microbiome Analysis. MSystems, 3(4), e00053-18. https://doi.org/10.1128/mSystems.00053-18
- Sajulga, R., Easterly, C., Riffle, M., Mesuere, B., Muth, T., Mehta, S., Kumar, P., Johnson, J., Gruening, B. A., Schiebenhoefer, H., Kolmeder, C. A., Fuchs, S., Nunn, B. L., Rudney, J., Griffin, T. J., & Jagtap, P. D. (2020). Survey of metaproteomics software tools for functional microbiome analysis. PLOS ONE, 15(11), e0241503-. https://doi.org/10.1371/journal.pone.0241503
- Sarhan, M. S., Hamza, M. A., Youssef, H. H., Patz, S., Becker, M., ElSawey, H., Nemr, R., Daanaa, H.-S. A., Mourad, E. F., Morsi, A. T., Abdelfadeel, M. R., Abbas, M. T., Fayez, M., Ruppel, S., & Hegazi, N. A. (2019). Culturomics of the plant prokaryotic microbiome and the dawn of plant-based culture media – A review. Journal of Advanced Research, 19, 15–27. https://doi.org/https://doi.org/10.1016/j.jare.2019.04.002
- Schiebenhoefer, H., van den Bossche, T., Fuchs, S., Renard, B. Y., Muth, T., & Martens, L. (2019). Challenges and promise at the interface of metaproteomics and genomics: an overview of recent progress in metaproteogenomic data analysis. Expert Review of Proteomics, 16(5), 375–390. https://doi.org/10.1080/14789450.2019.1609944
- Seng, P., Drancourt, M., Gouriet, F., la Scola, B., Fournier, P.-E., Rolain, J. M., & Raoult, D. (2009). Ongoing Revolution in Bacteriology: Routine Identification of Bacteria by Matrix-Assisted Laser Desorption Ionization Time-of-Flight Mass Spectrometry. Clinical Infectious Diseases, 49(4), 543–551. https://doi.org/10.1086/600885
- Shakya, M., Lo, C.-C., & Chain, P. S. G. (2019). Advances and Challenges in Metatranscriptomic Analysis. Frontiers in Genetics, 10. https://www.frontiersin.org/article/10.3389/fgene.2019.00904
- Wang, M., Carver, J. J., Phelan, V. v, Sanchez, L. M., Garg, N., Peng, Y., Nguyen, D. D., Watrous, J., Kapono, C. A., Luzzatto-Knaan, T., Porto, C., Bouslimani, A., Melnik, A. v, Meehan, M. J., Liu, W.-T., Crüsemann, M., Boudreau, P. D., Esquenazi, E., Sandoval-Calderón, M., … Bandeira, N. (2016). Sharing and community curation of mass spectrometry data with Global Natural Products Social Molecular Networking. Nature Biotechnology, 34(8), 828–837. https://doi.org/10.1038/nbt.3597
- Watrous, J., Roach, P., Alexandrov, T., Heath, B. S., Yang, J. Y., Kersten, R. D., van der Voort, M., Pogliano, K., Gross, H., Raaijmakers, J. M., Moore, B. S., Laskin, J., Bandeira, N., & Dorrestein, P. C. (2012). Mass spectral molecular networking of living microbial colonies. Proceedings of the National Academy of Sciences, 109(26), E1743. https://doi.org/10.1073/pnas.120368910