21 The Integrative Human Microbiome Project
The Integrative Human Microbiome Project is an open access article published in Nature under the Creative Commons: By Attribution 4.0 License (http://creativecommons.org/licenses/by/4.0/).
The Integrative Human Microbiome Project
The Integrative HMP (iHMP) Research Network Consortium
Abstract
The NIH Human Microbiome Project (HMP) has been carried out over ten years and two phases to provide resources, methods, and discoveries that link interactions between humans and their microbiomes to health-related outcomes. The recently completed second phase, the Integrative Human Microbiome Project, comprised studies of dynamic changes in the microbiome and host under three conditions: pregnancy and preterm birth; inflammatory bowel diseases; and stressors that affect individuals with prediabetes. The associated research begins to elucidate mechanisms of host–microbiome interactions under these conditions, provides unique data resources (at the HMP Data Coordination Center), and represents a paradigm for future multi-omic studies of the human microbiome.
Main
Although the ’omics era has accelerated all aspects of biological research, its effects have been particularly apparent in studies of microbial communities and the human microbiome. In the 18 years since the publication of the first human genome, studies of the microbiome have grown from culture-based surveys of the oral cavity and gut to molecular profiles of microbial biochemistry in all ecological niches of the human body1,2,3. Epidemiology and model systems have been used to identify associations between changes in the microbiome and conditions ranging from autism4 to cancer5,6,7, and microbial and immunological mechanisms have been identified that affect, for example, the efficacy of drugs used to treat cardiac conditions8 or survival during graft-versus-host disease9.
Contemporary studies of the human microbiome have also been a source of basic biological and translational surprises, exposing a compelling range of novel findings and open questions. Every human being appears to carry their own, largely individual, suite of microbial strains10,11, which are acquired early in life12,13,14, differ between environments and populations15,16, and can persist for years17 or undergo relatively rapid transitions18. Microbial diversity manifests differently in different ecological niches of the body; for example, greater diversity is generally expected in the gut, but can be associated with dysbiotic states and risk of adverse events in the female reproductive tract. The microbiome can be perturbed by conditions such as inflammatory bowel disease and diabetes, but a variety of microbiome-linked health states, and the underpinnings of these links, remain unexplored. How dynamic is the microbiome during processes such as pregnancy or viral infection? Which changes in the microbiome represent causes rather than effects of changes in health? Which molecular elements of a personalized microbiome might be responsible for health outcomes, and how do they integrate with and maintain physiological processes such as the immune system and metabolism? And what ecological elements dictate the success of a microbiota transplant, and why are they successful in treating some individuals and conditions, but not others?
The National Institutes of Health Human Microbiome Project was one of the first large-scale initiatives to address a subset of these questions19 (Fig. 1). Launched in 200720, the first phase of the program sought to determine whether there were common elements to ‘healthy’ microbiomes, in the absence of overt disease. Studies of both a baseline adult population21,22,23 and ‘demonstration’ populations with specific disease states established typical ranges (for some populations) of microbial membership and enzymatic repertoires across the body, combinations of metabolic functions that were either prevalent or strain-specific, and some of the host factors (such as race or ethnicity) that determine this variation. Studies of targeted populations identified ecological states of niches such as the vagina24,25, skin26,27,28, and gut29,30,31,32,33, among many others (https://www.hmpdacc.org/health/projectdemos.php). This first phase of the HMP (HMP1) thus yielded a wealth of community resources: nucleotide sequences of microorganisms and communities from a large number of isolates, individuals, and populations (http://hmpdacc.org)34,35,36,37; protocols to support reproducible body-wide microbiome sampling and data generation38,39,40; and computational methods for microbiome analysis and epidemiology41,42,43,44,45,46,47.
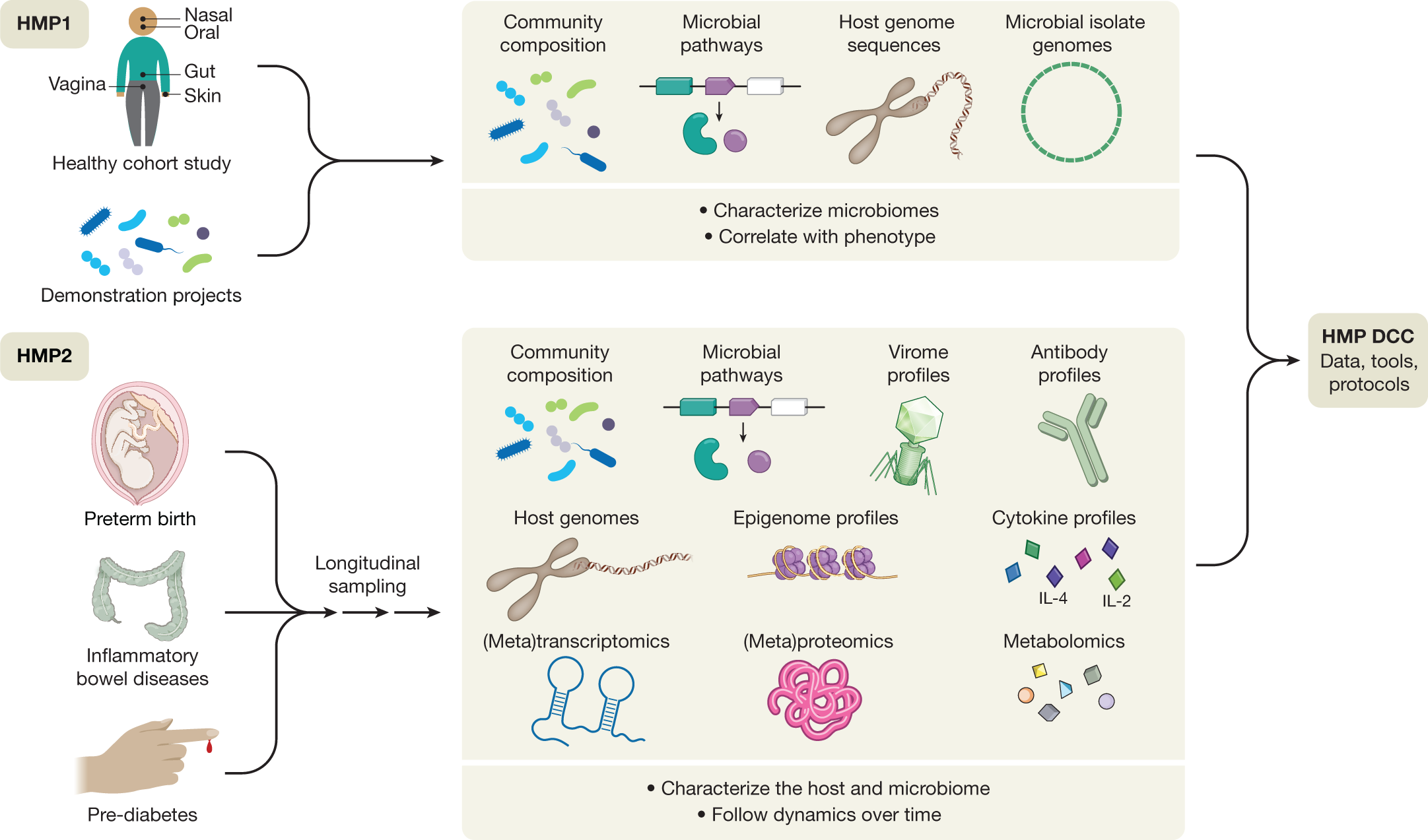
One of the main findings of the HMP1 was that the taxonomic composition of the microbiome alone was often not a good correlate with host phenotype—this tended to be better predicted by prevalent microbial molecular function or personalized strain-specific makeup21. This finding served as the foundation for the development of the second phase of the HMP, the Integrative HMP (iHMP or HMP2)48, which was designed to explore host–microbiome interplay, including immunity, metabolism, and dynamic molecular activity, to gain a more holistic view of host–microbe interactions over time. This multi-omic program sought to expand the resource base available to the microbiome research community, to begin to address the relationship between host and microbiome mechanistically, and to address the questions introduced above. Disease-targeted projects within the HMP2 were therefore encouraged to use multiple complementary approaches in order to assess the mechanisms of human and microbial activity longitudinally and to provide protocols, data, and biospecimens for future work. These projects included three studies that followed the dynamics of human health and disease during conditions with known microbiome interactions, thus addressing important health outcomes directly while also serving as models of ‘typical’ microbiome-associated conditions of broad interest to the research community. These comprised pregnancy and preterm birth (PTB); inflammatory bowel diseases (IBD); and stressors that affect individuals with prediabetes. These studies, which have now reached the first stage of completion49,50,51, together provide a wealth of information and insights about not only microbial dynamics, but also associated human host responses and microbial inter-relationships. A collection of more than 20 manuscripts to date describe some of these results at https://www.nature.com/collections/fiabfcjbfj, and together they provide a rich multi-omic data resource to be mined by future work (http://www.ihmpdcc.org).
The vaginal microbiome, pregnancy and preterm birth
Preterm birth can have devastating consequences for newborn babies, including death and long-term disability. In the United States, approximately 10% of births are premature52, and the incidence is even greater in lower-resource countries. Environmental factors, including the microbiome of the female reproductive tract, are important contributors to prematurity. Notably, these factors have a greater effect in women of African ancestry, who also bear the highest burden of PTB53. Infant mortality has been reduced in recent decades, but the incidence of PTB has not decreased54, and progress in predicting individual risk of PTB has stalled. During pregnancy, the maternal immune system maintains a delicate balance of pro- and anti-inflammatory effectors55, and contributors to PTB include breakdown in maternal–fetal tolerance, vascular disorders, stress, cervical insufficiency, premature rupture of the fetal membranes, and intra-amniotic infection56. Microbial ascension into the uterus is thought to precipitate PTB by disrupting the maternal immune balance, leading to spontaneous preterm labour, and/or by the release of microbial products (for example, collagenases, proteases or toxins) that compromise the integrity of fetal membranes and lead to premature rupture of the membranes57.
The Multi-Omic Microbiome Study: Pregnancy Initiative (MOMS-PI) research group, as part of HMP2, characterized the microbiomes of pregnant women to gauge their effects on risk of PTB (Fig. 2). The project followed 1,527 women longitudinally through pregnancy and involved the collection of 206,437 specimens, including maternal vaginal, buccal, rectal, skin and nares swabs, blood, urine, and birth products, as well as infant cord and cord blood, meconium and first stool, buccal, skin and rectal swabs. Subsets of these specimens underwent 16S rRNA gene taxonomic analysis, metagenomic and metatranscriptomic sequencing, cytokine profiling, lipidomics analysis, and bacterial genome analysis. The MOMS-PI team analysed 12,039 samples from 597 pregnancies to investigate the dynamics of the microbiome and its interactions with the host during pregnancy leading to PTB50.
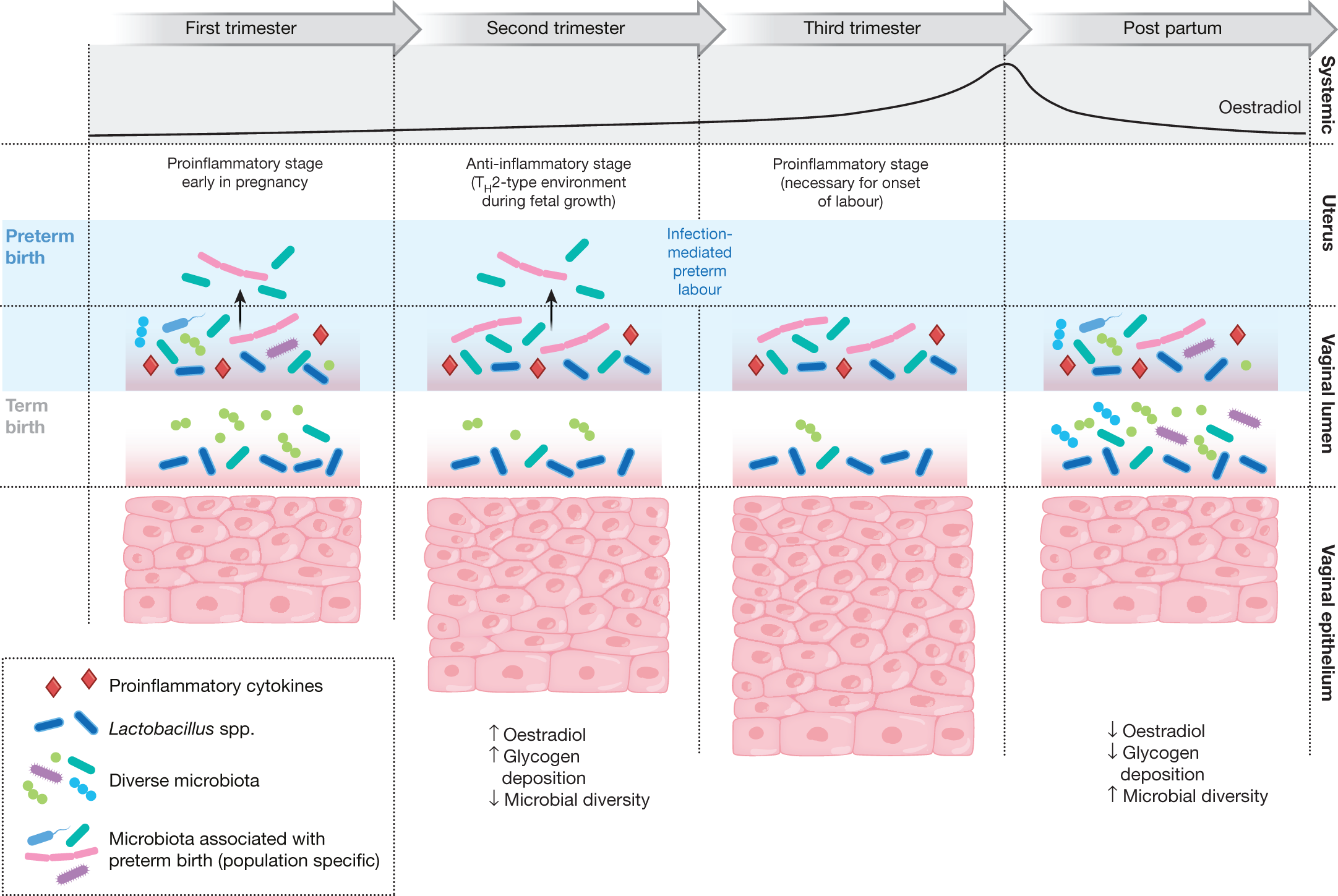
These multi-omic investigations identified temporal changes in the vaginal microbiome associated with full-term pregnancies. Women who often began pregnancy with a vaginal microbiome of greater ecological complexity generally converged towards a more homogeneous Lactobacillus-dominated microbiome by the second trimester58. Interestingly, this trend was most pronounced in women of African ancestry with lower socioeconomic profiles. Although the overall MOMS-PI cohort was demographically diverse, most women who experienced spontaneous PTB at less than 37 weeks of gestation were of African ancestry. The MOMS-PI team (http://vmc.vcu.edu/momspi) also identified signatures of higher risk for PTB in women who experienced spontaneous preterm birth at less than 37 weeks of gestation50. Women who went on to experience spontaneous PTB were less likely to exhibit a vaginal microbiota dominated by Lactobacillus crispatus, as previously reported in other populations59,60,61,62, and were more likely to exhibit an increased proportional abundance of several taxa including Sneathia amnii, Prevotella-related clades, a Lachnospiraceae taxon known as BVAB1, and a Saccharibacteria bacterium known as TM7-H1. Notably, these taxa were also associated with low levels of vitamin D63, suggesting that the vaginal microbiome might mediate a link between PTB risk and vitamin D deficiency64. The signatures of PTB were also reflected in metagenomic and metatranscriptomic measurements, and vaginal pro-inflammatory cytokines (including IL-1β, IL-6, MIP-1β and eotaxin-1) were positively correlated with PTB-associated taxa. Conveniently for future possible interventions, the vaginal microbiomes of mothers who experienced PTB were most distinct from those of control mothers early in pregnancy, and a preliminary model to predict risk of PTB was most sensitive and specific using vaginal microbiome profiles from samples collected before 24 weeks of gestation.
The MOMS-PI research group identified intriguing associations between the vaginal microbiota, host response, and pregnancy outcomes that are consistent with the involvement of microorganisms ascending from the vagina in at least some cases of spontaneous PTB. As an essential next step, the contribution of racial and demographic background to the vaginal microbiome in pregnancy with relation to pregnancy outcomes must be fully explored through harmonized, large-scale studies50. It is clear that PTB has a complex aetiology56. The relative contributions of fetal and maternal genetics and epigenetics, particularly as related to genetic variation of the innate immune system, should be explored. Harmonized large-scale studies would permit the development of population-specific risk assessment algorithms using vaginal microbiome profiles, features from genetic and prenatal (fetal) genetic screens, biomarkers such as cytokines and metabolites, and key clinical features from classic markers of risk including maternal age, body mass index, pregnancy history (including history of PTB), cervical length, and measures of stress and other environmental exposures. With the addition of new data from the microbiome, other environmental factors, and multi-omic inputs, new algorithms promise to improve our ability to predict risk of PTB early in pregnancy, to facilitate clinical trials by identifying high-risk patients, and ultimately to stratify patient populations into treatment groups.
The gut microbiome and inflammatory bowel disease
Studies of the gut microbiome in gastrointestinal disease have a particularly long and detailed history, especially in complex chronic conditions such as the inflammatory bowel diseases (IBD). IBD, including Crohn’s disease and ulcerative colitis, affects millions of individuals worldwide, with increasing incidence over the past 50 years or more coinciding with multiple factors such as westernization, urbanization, shifts in dietary patterns, antimicrobial exposure, and many more that could influence host–microbiome homeostasis65. The microbiome has long been implicated in IBD, potentially as a causative or risk factor66,67, as an explanation for heterogeneity in treatment response (that is, some individuals respond well to relatively benign aminosalicylates or corticosteroids whereas others still experience severe inflammation even after surgical intervention)68, or as a novel point of therapeutic intervention (for example, by transplantation of faecal microbiota69,70). Although meta-omic techniques have been used to identify functionally consistent microbial responses that help to explain the gut microbiome’s role as part of a pro-inflammatory feedback loop in the gut during disease71, and a few strains of microorganisms have been shown to be IBD-specific72, no comprehensive model of specific microbial, molecular, and immune interactions yet exists to explain the disease’s onset and dynamic progression.
Therefore, to better characterize mechanisms of host–microbiome dysregulation during disease, the Inflammatory Bowel Disease Multi’omics Database (IBDMDB) project followed 132 individuals from five clinical centres over the course of one year each as part of HMP2 (Fig. 3). Integrated longitudinal molecular profiles of microbial and host activity were generated by analysing 1,785 stool samples (self-collected and sent by mail every two weeks), 651 intestinal biopsies (collected colonoscopically at baseline), and 529 quarterly blood samples. To the extent possible, multiple molecular profiles were generated from the same sets of samples, including stool metagenomes, metatranscriptomes73, metaproteomes, viromes, metabolomes74,75, host exomes, epigenomes, transcriptomes, and serological profiles, among others, allowing concurrent changes to be observed in multiple types of host and microbial molecular and clinical activity over time. Protocols and results from the study, further information about its infrastructure, and both raw and processed76,77 data products are available through the IBDMDB data portal (http://ibdmdb.org), from the HMP2 Data Coordination Center (DCC; http://ihmpdcc.org), and in the accompanying manuscript49.
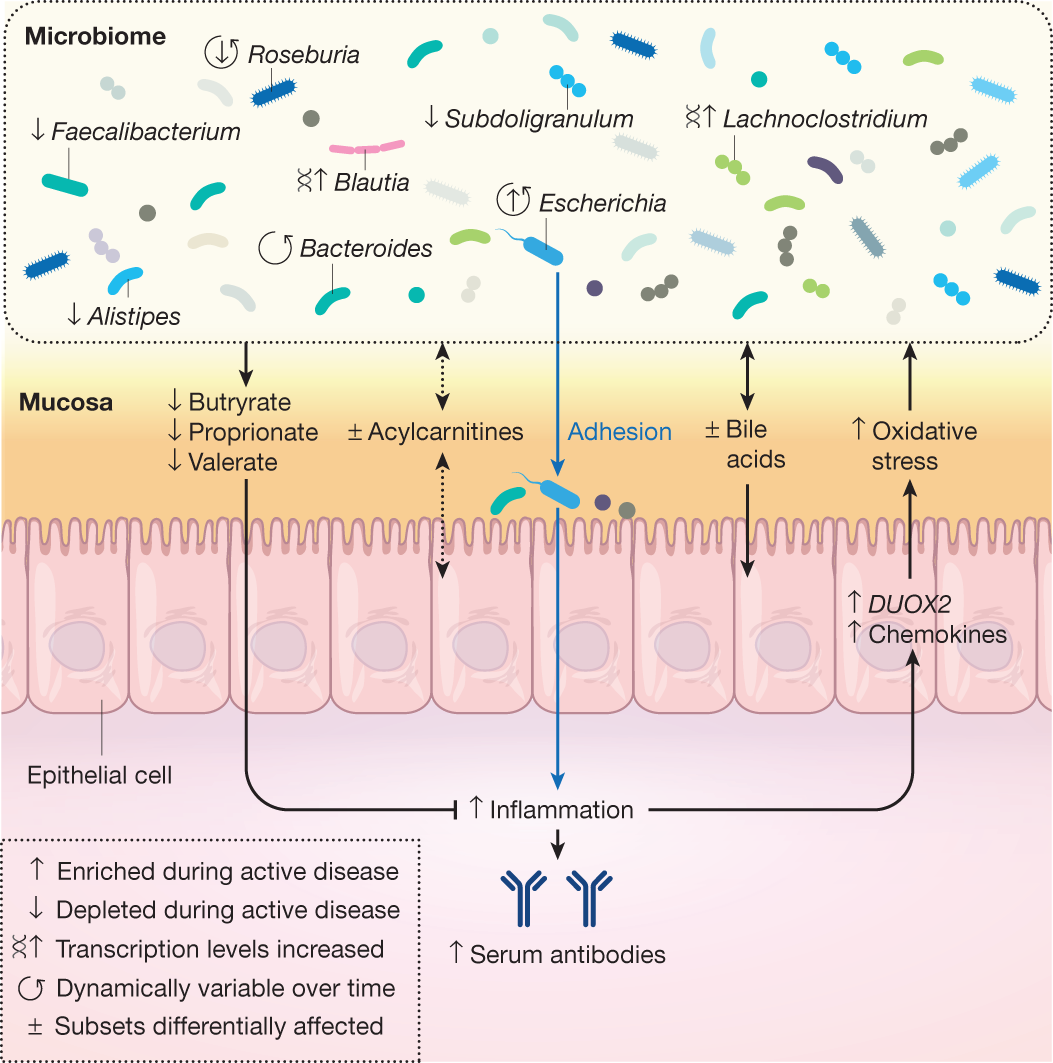
This unique study design allowed the IBDMDB to identify a variety of differences in the microbiome and host immune response over time during the course of the disease. Indeed, these dynamic changes were of much greater magnitude than were cross-sectional differences among clinical phenotypes, which have been emphasized by previous studies67,71,78,79. This was due in part to the prospective nature of the cohort, which recruited patients with Crohn’s disease or ulcerative colitis during both active and quiescent periods of disease, showing that microbial compositions in patients with IBD often revert to more control-like, ‘baseline’ configurations when the disease is not active. By identifying the gut microbial configurations that were most different from baseline—regardless of specific disease state—the study defined a dysbiosis score that called out highly divergent microbial compositions, which share many features common to an overall inflammatory response (for example, tolerance to oxidative stress). This dysbiosis was not unique to the microbial response to inflammation, however, and was associated with other host and biochemical alterations, pointing to new potential directions for management of systemic dysregulation in IBD. These included large shifts in acylcarnitine pools and bile acids, increased serum antibody levels, and alterations in transcription for several microbial species. Concurrent transcriptomics and 16S amplicon mucosal community profiling from biopsies also identified potential host factors that might be able to shape the microbial community, in particular several chemokines, highlighting these as being involved in a potentially dysregulated interaction during periods of disease activity49.
The study’s longitudinal multi-omic profiles further allowed researchers to characterize the stability and dynamics of host–microbiome interactions during disease, in particular highlighting ways in which community state and immune responses are distinctly less stable in participants with IBD than in control, healthy individuals. In numerous cases, the microbiome of a participant with IBD changed completely over the course of only weeks (measured as maximal Bray–Curtis dissimilarity to earlier samples from the same subject), whereas such shifts were rare in individuals without IBD. The main microbial contributors to these large-scale shifts from one time point to the next largely mirrored the differences observed in dysbiosis, and the shifts frequently marked the entrance into or exit from periods of dysbiosis. Finally, the study’s long-term, complementary molecular measurements enabled the construction of a network of more than 2,900 significant host and microbial cellular and molecular interactors during IBD, ranging from specific microbial taxa to human transcripts and small molecule metabolites. This network of mechanistic associations identified several key components that are central to the alterations seen in IBD, highlighting octanoyl carnitine, several lipids and short-chain fatty acids, the taxa Faecalibacterium, Subdoligranulum, Roseburia, Alistipes, and Escherichia, some at both the metagenomic and metatranscriptomic levels, and host regulators of interleukins49. Networks of mechanistic associations such as this may provide the key to disentangling the complex system of interactions that results in chronic inflammation in IBD and in other systemic microbiome-linked immune diseases.
Multi-omics profiling in prediabetes
Type 2 diabetes mellitus (T2D) affects more than 10% of the adult US population, and another 30% show early signs of the disease (referred to as prediabetes)80; 70% of the latter will develop diabetes in their lifetime. T2D is characterized by complex host–microbiome interactions81,82, but little is known about systemic alterations during prediabetes, their effects on biological processes, or the critical transition to full-blown T2D. Prediabetes and T2D are often associated with insulin resistance, and thus studies of individuals with prediabetes or insulin resistance offer unique opportunities to investigate the earliest stages of diabetes. It is essential to create a global and simultaneous profile of both host and microbial molecules in individuals with prediabetes over time, in order to fully understand the molecular pathways that are affected in people with prediabetes and/or insulin resistance and how these conditions affect both biological responses to environmental challenges (for example, viral infections83,84) and the onset of T2D.
To better understand T2D at its earliest stages, as part of iHMP, the Integrated Personal ’Omics Project (IPOP)85 followed 106 healthy and prediabetic individuals during quarterly periods of health, respiratory viral infection (RVI) and other perturbations over about four years51 (Fig. 4). In one such perturbation, a subset of 23 individuals underwent a directed weight gain followed by weight loss86. In total, 1,092 collections across all participants were profiled. For each visit, blood was assayed for host molecular ’omics profiling and two types of samples, nasal swabs and faeces, were collected for microbial profiling. Each participant’s exome was sequenced once; otherwise, for each visit, 13,379 transcripts were profiled from peripheral blood mononuclear cells, 722 metabolites and 302 proteins from plasma, and 62 cytokines and growth factors from serum. In addition, thousands of gut and nasal microbial taxa and computationally predicted genes were profiled using 16S rRNA amplicons. All visits were also intensively characterized by 51 clinical laboratory tests. In addition, because of the focus on T2D, a number of glucose dysregulation tests were performed, including measurements of fasting glucose and haemoglobin A1C levels, oral glucose tolerance tests, and tests of insulin resistance.
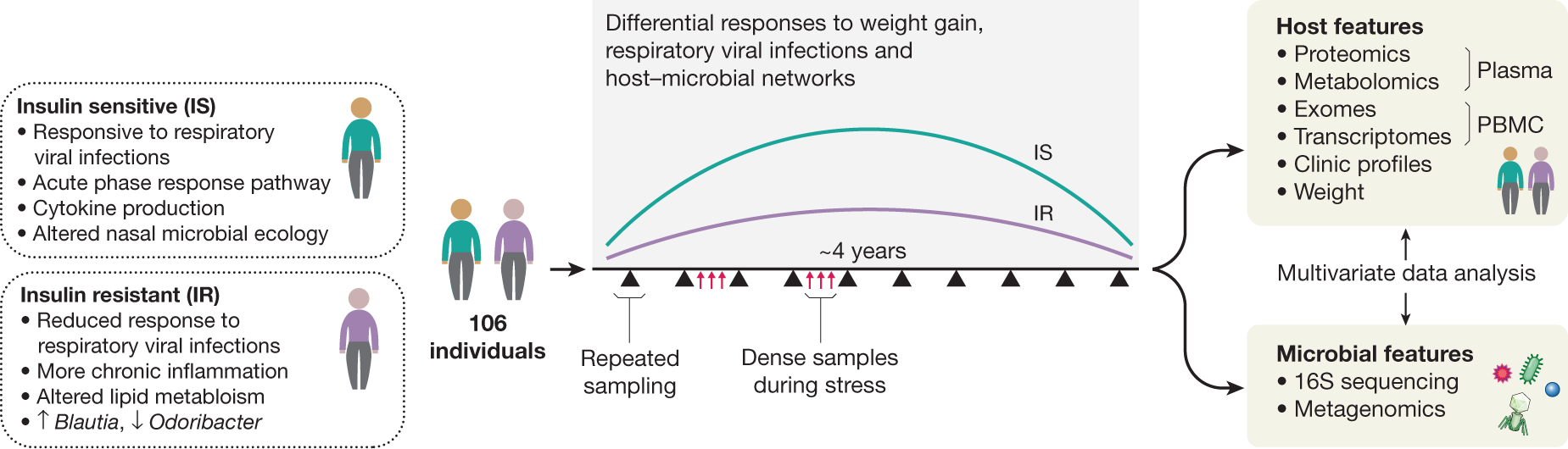
Baseline measurements were generally stable within individuals, even for long periods of time, with only some analytes changing significantly over time51. However, many analytes, such as clinical laboratory measurements, cytokine profiles, and gut microbial taxa (mostly those of low abundance) were highly variable between individuals. Participants who were ultimately insulin-resistant had distinguishable molecular and microbial patterns at baseline from those who were ultimately insulin-sensitive, and an analyte test was devised as part of the study in order to differentiate them. Notably, individuals undergoing RVI or changes in weight showed thousands of specific molecular and microbial changes during these perturbations, and insulin-resistant and insulin-sensitive individuals responded very differently to perturbations. For example, during RVI, insulin-resistant participants showed substantially decreased and delayed inflammatory responses (for example, the acute phase response and IL-1 signalling) and altered gut microbial changes when compared with insulin-sensitive participants (for example, in Lachnospiraceae and Rikenellaceae but not bacilli). Accordingly, there were fewer changes in nasal microbiota in insulin-resistant participants, and both the richness and the diversity of nasal microorganisms decreased during RVI in insulin-sensitive but not insulin-resistant participants. Furthermore, global co-association analyses among the thousands of profiled molecules revealed specific associations in insulin-resistant individuals that differ from those seen in insulin-sensitive participants and vice versa, indicating different patterns of host–microbiome interactions in the two groups51.
Another important goal of the study was to assess how host–microbiome multi-omics and related emerging technologies can be used to better manage patients’ health. We found that taking millions of measurements per individual over time enabled the early detection of potential disease states51,87. These included early detection of T2D, which developed differently among participants and was better detectable with varied assays; for example, some individuals first exhibited measurements in the diabetic range on tests of fasting glucose, whereas others did so on tests of haemoglobin A1c, oral glucose tolerance tests, or even continuous glucose monitoring. These results, together with detailed characterization of glucose dysregulation over time, illustrate the heterogeneity of T2D development. Overall, the data led to microbially linked, clinically actionable health discoveries in a number of diseases in addition to T2D, including metabolic disease, cardiovascular disease, haematological or oncological conditions, and other areas; these signs were often present before symptom onset, demonstrating the power of using big data, including the microbiome, to better manage human health.
Resources from the HMP2
Together, the HMP1 and HMP2 phases have produced a total of 42 terabytes of multi-omic data, which are archived and curated by the DCC at at http://ihmpdcc.org and in public and/or controlled-access repositories such as the Sequence Read Archive (SRA; https://www.ncbi.nlm.nih.gov/sra), the Database of Genotypes and Phenotypes (dbGaP; https://www.ncbi.nlm.nih.gov/gap/), Metabolomics Workbench (https://www.metabolomicsworkbench.org/), and others (Fig. 5). All data on the DCC is available for unrestricted use, with a subset of project metadata also being shared when permitted by institutional review boards (IRBs), and other restricted data (for example, human genome sequences and protected metadata) available through controlled access at dbGaP (projects PRJNA398089, PRJNA430481, PRJNA430482, PRJNA326441, phs001719, phs000256, phs001626, phs001523, and others). The formal data models and associated entity relationship schemas produced by all phases of the HMP are freely available at https://github.com/ihmpdcc/osdf-schemas. The DCC website allows users to find, query, search, visualize, and download data from thousands of samples with associated metadata. Once a user has identified a set of files, conditions, subjects, or phenotypes of interest, he or she can add this set to a shopping cart for further operations. Files can then be directly downloaded for use at the user’s local site or in the cloud. The HMP DCC efforts are thus by design consistent with the NIH’s stated goals to make all data generated from NIH funding findable, accessible, interoperable, and reusable88. The success of these efforts is evidenced by a consistently high rate of user access to the web resources, with 9,000–12,000 user sessions each month, and a greater throughput anticipated after the publication of these resources here.
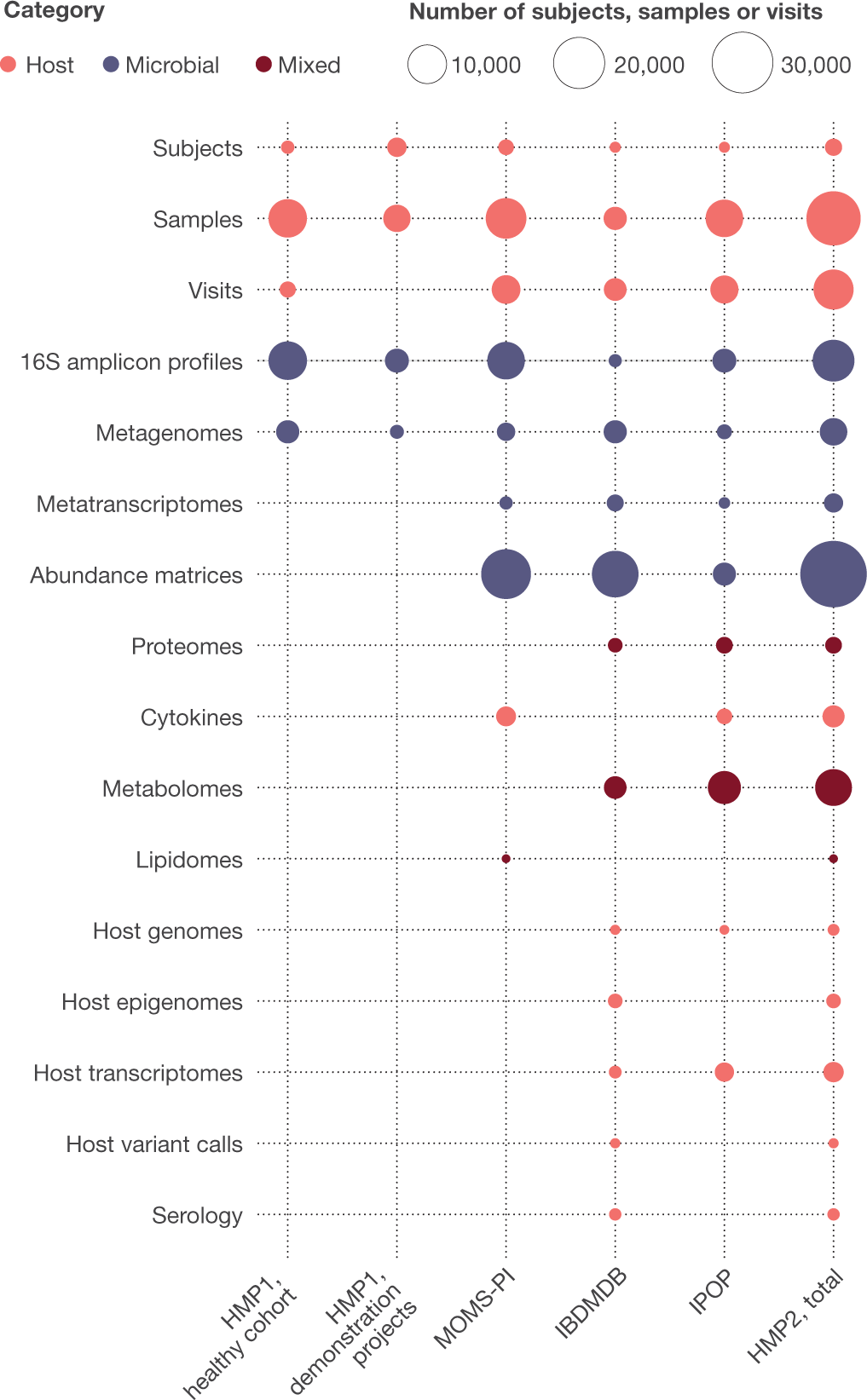
Complementary host–microbiome interactions
Although each of the three HMP2 studies revealed new biology within their respective areas of health and disease, a surprising range of host–microbiome immune and ecological features were common among them. The combination of shotgun metagenomics, untargeted metabolomics, and immunoprofiling was particularly effective, as in all projects this subset of molecular measurements tended to efficiently capture interpretable host and microbial properties that are linked to disease. Conversely, genetic variants were generally difficult to link to the microbiome in such small populations, which were necessary in order to deeply profile multi-omics over time, and we anticipate host sequencing to be more useful when integrated into larger cross-sectional surveys. Another notable property was that, as in most microbiome studies, changes that occurred within individuals, populations, or phenotypes were often much smaller than baseline variation between individuals. This is particularly true at microbiome-relevant time scales, for which repeated measures as rapid as days to weeks were necessary to capture the most specific host–microbiome interactions. Health-associated microbiome interactions can thus manifest in extremely diverse ways among individuals, making a combination of large-scale population surveys with within-subject longitudinal profiles essential for understanding the mechanisms of microbiome-linked disease.
As a result, other aspects of host–microbiome interactions were highly localized and subject-specific within each of the three studies. In all three conditions, microbial changes and associated host responses were strongest when captured at the time the changes occurred, and often within the tissue of origin. It is thus clear from these and other studies that host–microbiome interactions have both localized and systemic effects. Strong local perturbations initiated from either the host or microbial side can induce subsequent spatiotemporal responses that can continue over time and/or in other tissues, presumably with signals carried spatially by circulating small molecules and/or temporally by gene regulation or microbial growth, and involving regulatory circuits with both host and inter-microbial components. Continued coordinated efforts to measure the diverse host and microbial properties involved in each condition will thus be important for developing targeted and, when necessary, personalized therapies for microbiome-associated conditions, as well as for uncovering general principles that govern host–microbiome interactions. Other dynamic interactions that were not measured in all studies, such as an individual’s first microbial exposures near birth and subsequent immune development, may also represent key contributors to baseline microbiome personalization and help to explain disease-linked dynamics based on events that took place years or even decades earlier.
Next steps in microbiome multi-omics
The collective results of the NIH HMP projects, alongside many other studies, show that the microbiome is an integral component of human biology, with a major role in health and well-being. Inter-individual variability and highly diverse host–microbiome responses over time have driven the development of new methods for population microbiome studies using multiple, complementary longitudinal measurements, as well as highlighting the need to follow such studies up with mechanistic models in order to validate causative associations. The successful close of the HMP program itself has left an enduring legacy of multiple scientific generations of trained human microbiome investigators; provided the resulting community with a wealth of data, analytical, and biospecimen resources; and positioned the NIH and other funding agencies to continue work in a broad range of microbiome-linked conditions89. Funding for microbiome science, human and otherwise, is now being coordinated among NIH Centers and Institutes (https://www.niaid.nih.gov/research/trans-nih-microbiome-working-group); other US government agencies including the National Science Foundation, Environmental Protection Agency, Department of Energy, National Institute of Standards and Technology, Department of Agriculture, National Oceanographic and Atmospheric Administration, National Aeronautics and Space Administration, and Department of Defense (https://commonfund.nih.gov/hmp/programhighlights); philanthropic organizations including the Bill and Melinda Gates Foundation, the March of Dimes, the Burroughs Wellcome Fund, the Sloan Foundation, the Keck Foundation, the Juvenile Diabetes Research Foundation, the Crohn’s and Colitis Foundation, and others; and industry and public–private partnerships. Moreover, as complex global projects are launched to tackle aspects of personalized medicine, it is now obvious that it is informative to include components focused on the effect of the human microbiome.
As with any large study, the HMP2 has raised more new questions than it has answered. The aetiologies of baseline inter-individual differences in the microbiome, and of its dynamic changes over time, were not apparent even from the wide range of measurement types incorporated into these three studies and populations. Many immune and biochemical responses appear to be associated with specific strains that are unique to one or a few individual hosts, but it is not clear whether such strains are sufficient or necessary for their associated disease phenotypes. A few mechanisms were identified by which signals in the gut can be transmitted to systemic conditions such as diabetes, but not the specific small molecules or immune cell subsets by which they are likely to be transmitted—particularly in other health conditions that have not yet been studied in such detail. Finally, each HMP2 study was necessarily carried out within a geographically and genetically constrained population, and global differences in early life events, infectious disease exposure, or diet may change how microbiome dynamics contribute to human disease. Human-associated microbiology now clearly extends beyond infectious and gastrointestinal diseases to areas barely imaginable a few decades ago, including metabolism, neoplasia, maternal and child health, and central nervous system function. As the NIH HMP comes to an end, it is clear that its results have revealed a multitude of new avenues of research and technologies for future investigation, and we look forward to new discoveries based on resources from the program and exciting findings yet to come.
References
- Eckburg, P. B. et al. Diversity of the human intestinal microbial flora. Science 308, 1635–1638 (2005).
- Gill, S. R. et al. Metagenomic analysis of the human distal gut microbiome. Science 312, 1355–1359 (2006).
- Costello, E. K. et al. Bacterial community variation in human body habitats across space and time. Science 326, 1694–1697 (2009).
- Hsiao, E. Y. et al. Microbiota modulate behavioral and physiological abnormalities associated with neurodevelopmental disorders. Cell 155, 1451–1463 (2013).
- Gopalakrishnan, V. et al. Gut microbiome modulates response to anti-PD-1 immunotherapy in melanoma patients. Science 359, 97–103 (2018).
- Matson, V. et al. The commensal microbiome is associated with anti-PD-1 efficacy in metastatic melanoma patients. Science 359, 104–108 (2018).
- Routy, B. et al. Gut microbiome influences efficacy of PD-1-based immunotherapy against epithelial tumors. Science 359, 91–97 (2018).
- Haiser, H. J. et al. Predicting and manipulating cardiac drug inactivation by the human gut bacterium Eggerthella lenta. Science 341, 295–298 (2013).
- Taur, Y. et al. The effects of intestinal tract bacterial diversity on mortality following allogeneic hematopoietic stem cell transplantation. Blood 124, 1174–1182 (2014).
- Schloissnig, S. et al. Genomic variation landscape of the human gut microbiome. Nature 493, 45–50 (2013).
- Franzosa, E. A. et al. Identifying personal microbiomes using metagenomic codes. Proc. Natl Acad. Sci. USA 112, E2930–E2938 (2015).
- Dominguez-Bello, M. G. et al. Delivery mode shapes the acquisition and structure of the initial microbiota across multiple body habitats in newborns. Proc. Natl Acad. Sci. USA 107, 11971–11975 (2010).
- Koenig, J. E. et al. Succession of microbial consortia in the developing infant gut microbiome. Proc. Natl Acad. Sci. USA 108, 4578–4585 (2010).
- Vatanen, T. et al. The human gut microbiome in early-onset type 1 diabetes from the TEDDY study. Nature 562, 589–594 (2018).
- Yatsunenko, T. et al. Human gut microbiome viewed across age and geography. Nature 486, 222–227 (2012).
- Pasolli, E. et al. Extensive unexplored human microbiome diversity revealed by over 150,000 genomes from metagenomes spanning age, geography, and lifestyle. Cell 176, 649–662.e620, (2019).
- Faith, J. J. et al. The long-term stability of the human gut microbiota. Science 341, 1237439 (2013).
- Gajer, P. et al. Temporal dynamics of the human vaginal microbiota. Sci. Transl. Med. 4, 132ra152 (2012).
- Gevers, D. et al. The Human Microbiome Project: a community resource for the healthy human microbiome. PLoS Biol. 10, e1001377 (2012).
- Turnbaugh, P. J. et al. The Human Microbiome Project. Nature 449, 804–810 (2007).
- Human Microbiome Project Consortium. Structure, function and diversity of the healthy human microbiome. Nature 486, 207–214 (2012). HMP1 study analysing 5,177 16S rRNA gene sequencng profiles and 681 shotgun metagenomes spanning up to 18 body sites and three time points each from 242 healthy adults; established baseline ranges of taxonomic diversity within and between body sites, as well as strain personalization and functional commonalities.
- Human Microbiome Project Consortium. A framework for human microbiome research. Nature 486, 215–221 (2012).
- Lloyd-Price, J. et al. Strains, functions and dynamics in the expanded Human Microbiome Project. Nature 550, 61–66 (2017). Second wave of HMP1 data (HMP1-II) extending the analysis and data resource to 2,355 total shotgun metagenomes from 265 healthy adults; quantified strain personalization and retention dynamics over time, as well as identifying niche-specific and host-associated microbial community functions.
- Ravel, J. et al. Vaginal microbiome of reproductive-age women. Proc. Natl Acad. Sci. USA 108, 4680–4687 (2011).
- Fettweis, J. M. et al. Differences in vaginal microbiome in African American women versus women of European ancestry. Microbiology 160, 2272–2282 (2014).
- Kong, H. H. et al. Temporal shifts in the skin microbiome associated with disease flares and treatment in children with atopic dermatitis. Genome Res. 22, 850–859 (2012).
- Alekseyenko, A. V. et al. Community differentiation of the cutaneous microbiota in psoriasis. Microbiome 1, 31 (2013).
- Wylie, K. M., Mihindukulasuriya, K. A., Sodergren, E., Weinstock, G. M. & Storch, G. A. Sequence analysis of the human virome in febrile and afebrile children. PLoS One 7, e27735 (2012).
- Lewis, J. D. et al. Inflammation, antibiotics, and diet as environmental stressors of the gut microbiome in pediatric Crohn’s disease. Cell Host Microbe 18, 489–500 (2015).
- Zupancic, M. L. et al. Analysis of the gut microbiota in the old order Amish and its relation to the metabolic syndrome. PLoS One 7, e43052 (2012).
- Erickson, A. R. et al. Integrated metagenomics/metaproteomics reveals human host-microbiota signatures of Crohn’s disease. PLoS One 7, e49138 (2012).
- Frank, D. N. et al. Disease phenotype and genotype are associated with shifts in intestinal-associated microbiota in inflammatory bowel diseases. Inflamm. Bowel Dis. 17, 179–184 (2011).
- La Rosa, P. S. et al. Patterned progression of bacterial populations in the premature infant gut. Proc. Natl Acad. Sci. USA 111, 12522–12527 (2014).
- Fodor, A. A. et al. The “most wanted” taxa from the human microbiome for whole genome sequencing. PLoS One 7, e41294 (2012).
- Nelson, K. E. et al. A catalog of reference genomes from the human microbiome. Science 328, 994–999 (2010).
- Wylie, K. M. et al. Novel bacterial taxa in the human microbiome. PLoS One 7, e35294 (2012).
- Li, K., Bihan, M., Yooseph, S. & Methé, B. A. Analyses of the microbial diversity across the human microbiome. PLoS One 7, e32118 (2012).
- Aagaard, K. et al. The Human Microbiome Project strategy for comprehensive sampling of the human microbiome and why it matters. FASEB J. 27, 1012–1022 (2013).
- Schloss, P. D., Gevers, D. & Westcott, S. L. Reducing the effects of PCR amplification and sequencing artifacts on 16S rRNA-based studies. PLoS One 6, e27310 (2011).
- Jumpstart Consortium Human Microbiome Project Data Generation Working Group. Evaluation of 16S rDNA-based community profiling for human microbiome research. PLoS One 7, e39315 (2012).
- Gevers, D., Pop, M., Schloss, P. D. & Huttenhower, C. Bioinformatics for the Human Microbiome Project. PLoS Comput. Biol. 8, e1002779 (2012).
- Huse, S. M., Ye, Y., Zhou, Y. & Fodor, A. A. A core human microbiome as viewed through 16S rRNA sequence clusters. PLoS One 7, e34242 (2012).
- Markowitz, V. M. et al. IMG/M-HMP: a metagenome comparative analysis system for the Human Microbiome Project. PLoS One 7, e40151 (2012).
- Cantarel, B. L., Lombard, V. & Henrissat, B. Complex carbohydrate utilization by the healthy human microbiome. PLoS One 7, e28742 (2012).
- Rho, M., Wu, Y. W., Tang, H., Doak, T. G. & Ye, Y. Diverse CRISPRs evolving in human microbiomes. PLoS Genet. 8, e1002441 (2012).
- Faust, K. et al. Microbial co-occurrence relationships in the human microbiome. PLoS Comput. Biol. 8, e1002606 (2012).
- Abubucker, S. et al. Metabolic reconstruction for metagenomic data and its application to the human microbiome. PLoS Comput. Biol. 8, e1002358 (2012).
- Integrative HMP (iHMP) Research Network Consortium. The Integrative Human Microbiome Project: dynamic analysis of microbiome-host omics profiles during periods of human health and disease. Cell Host Microbe 16, 276–289 (2014).
- Lloyd-Price, J. et al. Multi-omics of the gut microbial ecosystem in inflammatory bowel diseases. Nature https://doi.org/10.1038/s41586-019-1237-9 (2019). HMP2 Inflammatory Bowel Disease Multi’omics Database (IBDMDB) study profiling 2,965 stool, biopsy, and blood specimens from 132 individuals with Crohn’s disease, ulcerative colitis or neither (control subjects) followed longitudinally for one year each; multi-omic profiling identified taxa, expressed functions, metabolite pools, and host gene expression that were disrupted in the gut during increased disease activity, as well as systemic effects such as serum antibody levels.
- Fettweis, J. M. et al. The vaginal microbiome and preterm birth. Nat. Med. https://doi.org/10.1038/s41591-019-0450-2 (2019). HMP2 preterm birth study of 1,527 pregnancies focusing on 45 spontaneous preterm birth events and 90 case-matched controls, together yielding over 12,000 multi-omically profiled samples; 16S rRNA gene, metagenomic, metatranscriptomic and cytokine profiles identified differential levels of Lactobacillus crispatus, BVAB1, Sneathia amnii, TM7-H1, and additional taxa associated with preterm deliveries.
- Zhou, W. et al. Longitudinal multi-omics of host–microbe dynamics in prediabetes. Nature https://doi.org/10.1038/s41586-019-1236-x (2019). HMP2 prediabetes study of 106 participants spanning 1,092 visits, profiling host transcriptomes, metabolomes, cytokines, and proteomes, as well as microbial taxonomic and genomic changes during healthy periods, respiratory viral infections, immunizations, and other perturbations; most molecular profiles were personalized, perturbed during infections and immunizations, and associated with changes in insulin sensitivity.
- Behrman, R. E. & Butler A. S. (eds.) Preterm Birth: Causes, Consequences, and Prevention (National Academies Press, 2007).
- York, T. P., Eaves, L. J., Neale, M. C. & Strauss, J. F. III. The contribution of genetic and environmental factors to the duration of pregnancy. Am. J. Obstet. Gynecol. 210, 398–405 (2014).
- Liu, L. et al. Global, regional, and national causes of under-5 mortality in 2000–15: an updated systematic analysis with implications for the Sustainable Development Goals. Lancet 388, 3027–3035 (2016).
- Mor, G., Aldo, P. & Alvero, A. B. The unique immunological and microbial aspects of pregnancy. Nat. Rev. Immunol. 17, 469–482 (2017).
- Romero, R., Dey, S. K. & Fisher, S. J. Preterm labor: one syndrome, many causes. Science 345, 760–765 (2014).
- Jefferson, K. K. The bacterial etiology of preterm birth. Adv. Appl. Microbiol. 80, 1–22 (2012).
- Serrano, M. G. et al. Racioethnic diversity in the dynamics of the vaginal microbiome during pregnancy. Nat. Med. https://doi.org/10.1038/s41591-019-0465-8 (2019). HMP2 term birth study of 613 pregnant women, 1,969 non-pregnant women, and an additional 90 pregnant women followed longitudinally profiling vaginal, buccal, blood, urine, and rectal samples; shifts toward Lactobacillus -dominated communities tended to occur early during pregnancy, particularly in women of African or Hispanic descent.
- DiGiulio, D. B. et al. Temporal and spatial variation of the human microbiota during pregnancy. Proc. Natl Acad. Sci. USA 112, 11060–11065 (2015).
- Callahan, B. J. et al. Replication and refinement of a vaginal microbial signature of preterm birth in two racially distinct cohorts of US women. Proc. Natl Acad. Sci. USA 114, 9966–9971 (2017).
- Kindinger, L. M. et al. The interaction between vaginal microbiota, cervical length, and vaginal progesterone treatment for preterm birth risk. Microbiome 5, 6 (2017).
- Brown, R. G. et al. Vaginal dysbiosis increases risk of preterm fetal membrane rupture, neonatal sepsis and is exacerbated by erythromycin. BMC Med. 16, 9 (2018).
- Jefferson, K. K. et al. Relationship between vitamin D status and the vaginal microbiome during pregnancy. J. Perinatol. https://doi.org/10.1038/s41372-019-0343-8 (2019).
- Zhou, S. S., Tao, Y. H., Huang, K., Zhu, B. B. & Tao, F. B. Vitamin D and risk of preterm birth: Up-to-date meta-analysis of randomized controlled trials and observational studies. J. Obstet. Gynaecol. Res. 43, 247–256 (2017).
- Huang, H. et al. Fine-mapping inflammatory bowel disease loci to single-variant resolution. Nature 547, 173–178 (2017).
- Manichanh, C. et al. Reduced diversity of faecal microbiota in Crohn’s disease revealed by a metagenomic approach. Gut 55, 205–211 (2005).
- Frank, D. N. et al. Molecular-phylogenetic characterization of microbial community imbalances in human inflammatory bowel diseases. Proc. Natl Acad. Sci. USA 104, 13780–13785 (2007).
- Kugathasan, S. et al. Prediction of complicated disease course for children newly diagnosed with Crohn’s disease: a multicentre inception cohort study. Lancet 389, 1710–1718 (2017).
- Narula, N. et al. Systematic review and meta-analysis: fecal microbiota transplantation for treatment of active ulcerative colitis. Inflamm. Bowel Dis. 23, 1702–1709 (2017).
- Jeon, S. R., Chai, J., Kim, C. & Lee, C. H. Current evidence for the management of inflammatory bowel diseases using fecal microbiota transplantation. Curr. Infect. Dis. Rep. 20, 21 (2018).
- Morgan, X. C. et al. Dysfunction of the intestinal microbiome in inflammatory bowel disease and treatment. Genome Biol. 13, R79 (2012).
- Hall, A. B. et al. A novel Ruminococcus gnavus clade enriched in inflammatory bowel disease patients. Genome Med. 9, 103 (2017).
- Schirmer, M. et al. Dynamics of metatranscription in the inflammatory bowel disease gut microbiome. Nat. Microbiol. 3, 337–346 (2018).
- Franzosa, E. A. et al. Gut microbiome structure and metabolic activity in inflammatory bowel disease. Nat. Microbiol. 4, 293–305 (2019).
- Mallick, H. et al. Predictive metabolomic profiling of microbial communities using amplicon or metagenomic sequences. Nat. Commun. (in the press).
- Franzosa, E. A. et al. Species-level functional profiling of metagenomes and metatranscriptomes. Nat. Methods 15, 962–968 (2018).
- McIver, L. J. et al. bioBakery: a meta’omic analysis environment. Bioinformatics 34, 1235–1237 (2018).
- Willing, B. P. et al. A pyrosequencing study in twins shows that gastrointestinal microbial profiles vary with inflammatory bowel disease phenotypes. Gastroenterology 139, 1844–1854.e1841 (2010).
- Gevers, D. et al. The treatment-naive microbiome in new-onset Crohn’s disease. Cell Host Microbe 15, 382–392 (2014).
- Cowie, C. C. et al. Full accounting of diabetes and pre-diabetes in the U.S. population in 1988–1994 and 2005–2006. Diabetes Care 32, 287–294 (2009).
- Pickup, J. C. Inflammation and activated innate immunity in the pathogenesis of type 2 diabetes. Diabetes Care 27, 813–823 (2004).
- Qin, J. et al. A metagenome-wide association study of gut microbiota in type 2 diabetes. Nature 490, 55–60 (2012).
- Gamble, D. R., Kinsley, M. L., FitzGerald, M. G., Bolton, R. & Taylor, K. W. Viral antibodies in diabetes mellitus. BMJ 3, 627–630 (1969).
- Mehta, S. H. et al. Prevalence of type 2 diabetes mellitus among persons with hepatitis C virus infection in the United States. Ann. Intern. Med. 133, 592–599 (2000).
- Chen, R. et al. Personal omics profiling reveals dynamic molecular and medical phenotypes. Cell 148, 1293–1307 (2012).
- Piening, B. D. et al. Integrative personal omics profiles during periods of weight gain and loss. Cell Syst. 6, 157–170 e158 (2018).
- Schüssler-Fiorenza Rose, S. et al. A longitudinal big data approach for precision health. Nat. Med. 25, 792–804 (2019). HMP2 Integrative Personalized ’Omics Profiling of 109 individuals enriched for risk of type 2 diabetes studied genomically, immunologically, transcriptomically, proteomically, metabolomically, and using wearable monitoring; multiple molecular pathways were associated with metabolic, cardiovascular and oncological pathophysiology, and a subset were predictive of insulin resistance.
- Wilkinson, M. D. et al. The FAIR Guiding Principles for scientific data management and stewardship. Sci. Data 3, 160018 (2016).
- NIH Human Microbiome Portfolio Analysis Team. A review of 10 years of human microbiome research activities at the US National Institutes of Health, Fiscal Years 2007–2016. Microbiome 7, 31 (2019).
Acknowledgements
We thank the NIH Common Fund (particularly M. E. Perry), the Trans-NIH Microbiome Working Group (TMWG) and the HMP Science Advisors (iHMP advisors: J. Davies, F. Ouellette, E. Chang, and the late S. Falkow) for their support throughout the HMP program, and additional project principal investigators K. Jefferson and R. Xavier. We acknowledge funding from NIH grants UH2/UH3AI083263 and U54HD080784 (G.A.B., J.F.S., K. Jefferson) supported with funds from the Common Fund, the National Center for Complementary and Integrative Health, and the Office of Research on Women’s Health, grant U54DK102557 (C.H., R. Xavier), including funds from the Common Fund, the National Institute of Diabetes and Digestive and Kidney Diseases, the National Center for Complementary and Integrative Health, and the Office of Dietary Supplements and grant U54DK102556 (M.P.S., G.M.W.), with funds from the Common Fund and the National Institute of Diabetes and Digestive and Kidney Diseases.
Reviewer information
Nature thanks Frederic Bushman and the other anonymous reviewer(s) for their contribution to the peer review of this work.
Author information
A list of participants and their affiliations appears at the end of the paper.
These authors contributed equally: Lita M. Proctor, Heather H. Creasy, Jennifer M. Fettweis, Jason Lloyd-Price, Anup Mahurkar, Wenyu Zhou.
Affiliations
Consortia
Contributions
All authors contributed manuscript text and created or edited figures. Individual HMP2 projects discussed were implemented and managed by J.M.F., G.A.B. and J.F.S. (PTB); J.L.-P. and C.H. (IBD); W.Z., M.P.S. and G.M.W. (T2D); H.H.C., A.M. and O.W. (DCC).
Corresponding authors
Ethics declarations
Competing interests
M.S. is a cofounder of Personalis, Qbio, Sensomics, January, Filtricine and Akna and advisor for Genapsys. The other authors declare no competing interests.
Additional information
Publisher’s note: Springer Nature remains neutral with regard to jurisdictional claims in published maps and institutional affiliations.
Rights and permissions
Open Access This article is licensed under a Creative Commons Attribution 4.0 International License, which permits use, sharing, adaptation, distribution and reproduction in any medium or format, as long as you give appropriate credit to the original author(s) and the source, provide a link to the Creative Commons license, and indicate if changes were made. The images or other third party material in this article are included in the article’s Creative Commons license, unless indicated otherwise in a credit line to the material. If material is not included in the article’s Creative Commons license and your intended use is not permitted by statutory regulation or exceeds the permitted use, you will need to obtain permission directly from the copyright holder. To view a copy of this license, visit http://creativecommons.org/licenses/by/4.0/.